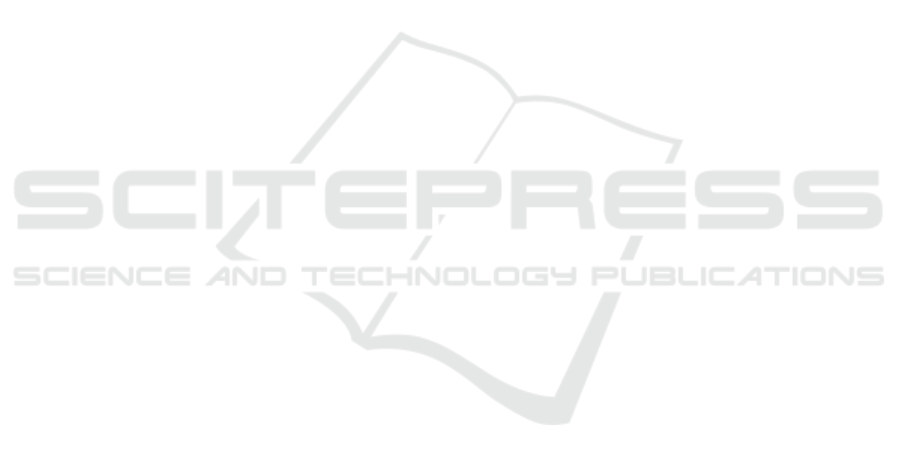
REFERENCES
Arrieta, A., Diaz-Rordiguez, N., Ser, J. D., Bennetot, A.,
Tabik, S., Barbado, A., Garcia, S., Gil-L
´
opez, S.,
Molina, D., and Benjamins, R. (2020). Explainable
artificial intelligence (xai): Concepts, taxonomies, op-
portunities and challenges toward responsible ai. In
Information Fusion.
Baker, C., Fillmore, C., and Lowe, J. (1998). The berkeley
framenet project. In In Proceedings of the Coling-Acl.
Barba, E., Procopio, L., and Navigli, R. (2021). Consec:
Word sense disambiguation as continuous sense com-
prehension. In Conference on Empirical Methods in
Natural Language Processing.
Beetz, M., Bessler, D., Haidu, A., Pomarlan, M.,
Bozcuoglu, A. K., and Bartels, G. (2018). Know rob
2.0 — a 2nd generation knowledge processing frame-
work for cognition-enabled robotic agents. In Inter-
national Conference on Robotics and Automation.
Bevilacqua, M., Pasini, T., Raganato, A., and Navigli, R.
(2021). Recent trends in word sense disambiguation:
A survey. In International Joint Conference on Artifi-
cial Intelligence.
Das, A., Datta, S., Gkioxari, G., S. Lee, D. P., and Ba-
tra, D. (2017). Embodied question answering. https://
arxiv.org/abs/1711.11543. Accessed:2022-07-11.
Diaconu, A. (2020). Learning functional programs
with function invention and reuse. https://
arxiv.org/abs/2011.08881. Accessed: 2022-07-11.
Duan, J., Yu, S., Tan, H. L., Zhu, H., and Tan, C. (2022). A
survey of embodied ai: From simulators to research
tasks. https://arxiv.org/abs/2103.04918. Accessed:
2022-07-11.
Eggert, J., Deigmoeller, J., Fischer, L., and Richter, A.
(2019). Memory nets: Knowledge representation for
intelligent agent operations in real world. In 11th
International Conference on Knowledge Engineering
and Ontology Development. SCITEPRESS.
Eggert, J., Deigmoeller, J., Fischer, L., and Richter, A.
(2020). Action representation for intelligent agents
using memory nets. In Communications in Computer
and Information Science. SPRINGER.
Fellbaum, C. (1998). WordNet: An Electronic Lexical
Database. Bradford Books.
Gordon, D., Kembhavi, A., Rastegari, M., Redmon,
J., Fox, D., and Farhadi, A.(2018). Iqa: Vi-
sual question answering in interactive environments.
https://arxiv.org/abs/1712.03316. Accessed: 2022-07-
11.
He, P., Liu, X., Gao, J., and Chen, W. (2021). Deberta:
Decoding-enhanced bert with disentangled attention.
In Conference on Learning Representations.
Honnibal, M. and Montani, I. (2017). spaCy 2: Natural lan-
guage understanding with Bloom embeddings, con-
volutional neural networks and incremental parsing.
https://sentometrics-research.com/publication/72.
Accessed: 2022-07-11.
Jiao, A. (2020). An intelligent chatbot system based on en-
tity extraction using rasa nlu and neural network. In J.
Phys.: Conf. Ser.
Kolve, E., Mottaghi, R., Han, W., VanderBilt, E., Weihs,
L., Herrasti, A., Gordon, D., Zhu, Y., Gupta,
A., and Farhadi, A. (2019). Ai2-thor: An in-
teractive 3d environment for visual ai. https://
arxiv.org/abs/1712.05474.Accessed: 2022-07-11.
Losing, V., Fischer, L., and Deigmoeller, J. (2021). Extrac-
tion of common-sense relations from procedural task
instructions using BERT. In Proceedings of the 11th
Global Wordnet Conference.
Miller, G. A., Chodorow, M., Landes, S., Leacock, C., and
Thomas, R. G. (1994). Using a semantic concordance
for sense identification. In Human Language Technol-
ogy: Proceedings of a Workshop held at Plainsboro.
Pandya, H. A. and Bhatt, B. S. (2019). Question answer-
ing survey: Directions, challenges, datasets, evalua-
tion matrices. https://arxiv.org/abs/2112.03572. Ac-
cessed: 2022-07-11.
Paulius, D. and Sun, Y. (2018). A survey of knowledge rep-
resentation in service robotics. In Robotics and Au-
tonomous Systems.
Puig, X., Ra, K., Boben, M., Li, J., Wang, T., Fidler, S.,
and Torralba, A. (2018). VirtualHome: Simulating
household activities via programs. In Conference on
Computer Vision and Pattern Recognition.
Schuler, K. K. (2005). VerbNet: A Broad-Coverage, Com-
prehensive Verb Lexicon. University of Pennsylvania.
Speer, R., Chin, J., and Havasi, C. (2017). Conceptnet 5.5:
An open multilingual graph of general knowledge. In
Proceedings of AAAI-31.
Spinner, T., Schlegel, U., Sch
¨
afer, H., and El-Assady, M.
(2019). explainer: A visual analytics framework for
interactive and explainable machine learning. In IEEE
Transactions On Visualization And Computer Graph-
ics.
Tan, S., Ge, M., Guo, D., Liu, H., and Sun, F.
(2021). Knowledge-based embodied question an-
swering. https://arxiv.org/abs/2109.07872. Accessed:
2022-07-11.
Tangiuchi, T., Mochihashi, D., Nagai, T., Uchida, S., Inoue,
N., Kobayashi, I., Nakamura, T., Hagiwara, Y., Iwa-
hashi, N., and Inamura, T. Survey on frontiers of lan-
guage and robotics. https://arxiv.org/abs/2112.03572.
Accessed: 2022-07-11.
Thosar, M., Zug, S., Skaria, A. M., and Jain, A. (2018).
A review of knowledge bases for service robots in
household environments. In 6th International Work-
shop on Artificial Intelligence and Cognition.
Tiddi, I. and Schlobach, S. (2022). Knowledge graphs as
tools for explainable machine learning: A survey. In
Artificial Intelligence.
Tjoa, E. and Guan, C. (2020). A survey on explainable arti-
ficial intelligence (xai): Toward medical xai. In IEEE
Transactions On Neural Networks And Learning Sys-
tems.
Yu, L., Chen, X., Gkioxari, G., Bansal, M., Berg, T. L., and
Batra, D. (2019). Multi-target embodied question an-
swering. https://arxiv.org/abs/1904.04686. Accessed:
2022-07-11.
KEOD 2022 - 14th International Conference on Knowledge Engineering and Ontology Development
176