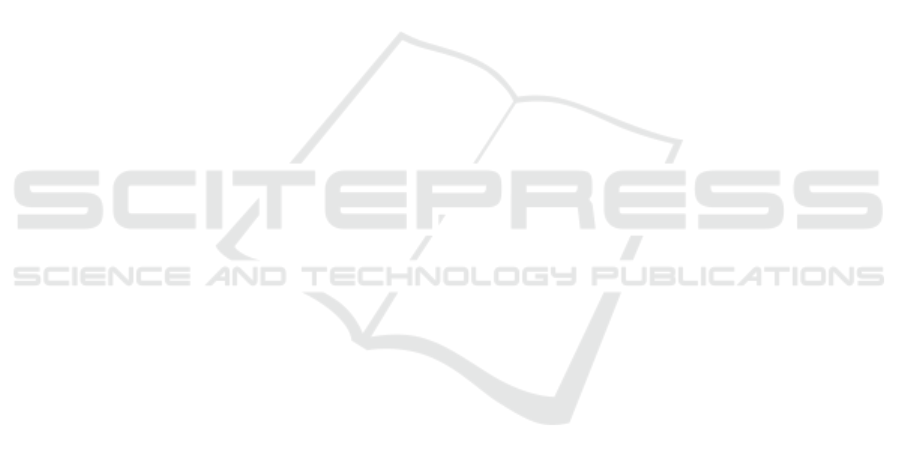
6 CONCLUSION
In response to audience demand, user experience
enhancement is an imperative action for the sports
broadcasting industry. This paper presented a step
forward to accomplish this enhancement, based on
players’ positional data extraction and representation.
Extracting this data has complex associated problems
and the results reflect this complexity. However, there
are one or more proposed alternatives for each prob-
lem encountered. The proposed solution reflects on-
going work. The current solution can be integrated
into sports broadcasting. Future work includes the
overlay of specific game-related events.The results
achieved so far create good expectations for the up-
coming developments and for the potential of the
MOGSports business case within the media and en-
tertainment industries.
ACKNOWLEDGEMENTS
This work has been supported by the H2020 DATA-
CLOUD project, funded by European Union’s Hori-
zon 2020 research and innovation programme under
grant agreement No 101016835, as well as by the Na-
tional Funds through the Portuguese funding agency,
FCT - Fundac¸
˜
ao para a Ci
ˆ
encia e a Tecnologia, within
project UIDB/50014/2020.
REFERENCES
Chen, J. and Little, J. J. (2019). Sports camera calibration
via synthetic data. In 2019 IEEE/CVF Conference on
Computer Vision and Pattern Recognition Workshops
(CVPRW), pages 2497–2504.
Cioppa, A., Deliege, A., Magera, F., Giancola, S., Bar-
nich, O., Ghanem, B., and Van Droogenbroeck, M.
(2021). Camera calibration and player localization in
soccernet-v2 and investigation of their representations
for action spotting. In Proceedings of the IEEE/CVF
Conference on Computer Vision and Pattern Recogni-
tion, pages 4537–4546.
Cuevas, C., Quilon Gonzalez, D., and Garc
´
ıa, N. (2020).
Techniques and applications for soccer video analysis:
A survey. Multimedia Tools and Applications, 79.
Ivasic-Kos, M., Host, K., and Pobar, M. (2021). Application
of deep learning methods for detection and tracking of
players. In Deep Learning Applications. IntechOpen.
Jocher, G., Chaurasia, A., Stoken, A., Borovec, J.,
NanoCode012, Kwon, Y., TaoXie, Fang, J., imyhxy,
Michael, K., Lorna, V, A., Montes, D., Nadar, J.,
Laughing, tkianai, yxNONG, Skalski, P., Wang, Z.,
Hogan, A., Fati, C., Mammana, L., AlexWang1900,
Patel, D., Yiwei, D., You, F., Hajek, J., Diaconu, L.,
and Minh, M. T. (2022). ultralytics/yolov5: v6.1 -
TensorRT, TensorFlow Edge TPU and OpenVINO Ex-
port and Inference.
Nguyen, T., Chen, S. W., Shivakumar, S. S., Taylor, C. J.,
and Kumar, V. (2018). Unsupervised deep homogra-
phy: A fast and robust homography estimation model.
IEEE Robotics and Automation Letters, 3(3):2346–
2353.
Nie, X., Chen, S., and Hamid, R. (2021). A robust and effi-
cient framework for sports-field registration. In 2021
IEEE Winter Conference on Applications of Computer
Vision (WACV), pages 1935–1943.
Rematas, K., Kemelmacher-Shlizerman, I., Curless, B., and
Seitz, S. (2018). Soccer on your tabletop. In Proceed-
ings of the IEEE conference on computer vision and
pattern recognition, pages 4738–4747.
Sah, M. and Direkoglu, C. (2021). Review and evaluation of
player detection methods in field sports: Comparing
conventional and deep learning based methods. Mul-
timedia Tools and Applications.
Theiner, J., Gritz, W., M
¨
uller-Budack, E., Rein, R., Mem-
mert, D., and Ewerth, R. (2022). Extraction of po-
sitional player data from broadcast soccer videos. In
Proceedings of the IEEE/CVF Winter Conference on
Applications of Computer Vision, pages 823–833.
Wojke, N., Bewley, A., and Paulus, D. (2017). Simple on-
line and realtime tracking with a deep association met-
ric. In 2017 IEEE international conference on image
processing (ICIP), pages 3645–3649. IEEE.
Tracking Data Visual Representations for Sports Broadcasting Enrichment
131