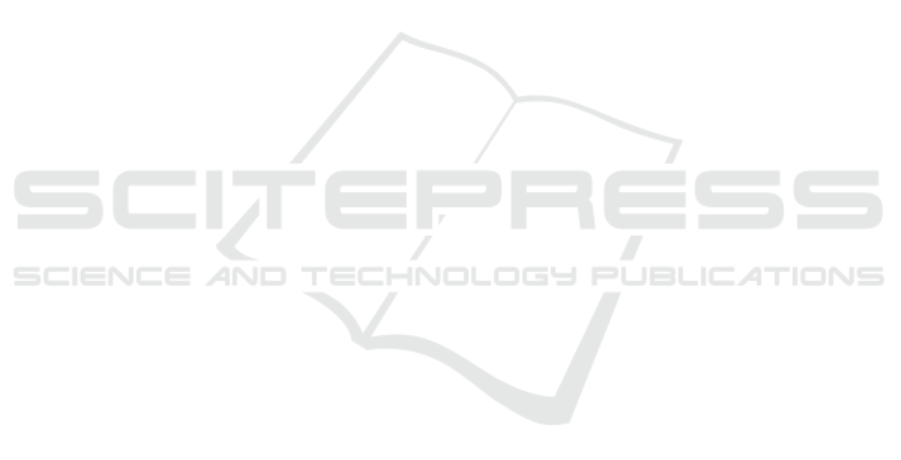
ACKNOWLEDGEMENTS
This work was carried out within the AENEAS
project, which is funded by the Austrian Min-
istry for Climate Action, Environment, Energy, In-
novation and Technology (BMK) under the pro-
gram “Eurostars-2/Road Transport Technology” and
is managed by the Austrian Research Promotion
Agency (FFG). We acknowledge the support and con-
tributions by the entire AENEAS consortium, consist-
ing of DTV-Verkehrsconsult GmbH (Germany) as co-
ordinator, 4D-IT GmbH (Austria) and the AIT.
REFERENCES
Balali, V. and Golparvar-Fard, M. (2015). Segmentation
and recognition of roadway assets from car-mounted
camera video streams using a scalable non-parametric
image parsing method. Automation in Construction,
49:27–39.
Blender-Foundation (2022). Blender - a 3D modelling and
rendering package. Blender Foundation, Stichting
Blender Foundation, Amsterdam.
Chen, M. (2021). Vectorized dataset of roadside noise bar-
riers in china. National Tibetan Plateau Data Center.
Comaniciu, D. and Meer, P. (2002). Mean shift: A robust
approach toward feature space analysis. IEEE Trans.
Pattern Anal. Mach. Intell., 24(5):603–619.
Fang, L., Yang, B., Chen, C., and Fu, H. (2015). Extraction
3d road boundaries from mobile laser scanning point
clouds. In 2nd IEEE International Conference on Spa-
tial Data Mining and Geographical Knowledge Ser-
vices, ICSDM 2015, Fuzhou, China, July 8-10, 2015,
pages 162–165.
Georgakis, G., Mousavian, A., Berg, A. C., and Kosecka, J.
(2017). Synthesizing training data for object detection
in indoor scenes. CoRR, abs/1702.07836.
Golovinskiy, A., Kim, V. G., and Funkhouser, T. (2009).
Shape-based recognition of 3D point clouds in urban
environments. International Conference on Computer
Vision (ICCV).
Golparvar-Fard, M., Balali, V., and de la Garza, J. M.
(2015). Segmentation and recognition of highway as-
sets using image-based 3d point clouds and semantic
texton forests. Journal of Computing in Civil Engi-
neering, 29(1):04014023.
Hinterstoisser, S., Pauly, O., Heibel, H., Marek, M., and
Bokeloh, M. (2019). An annotation saved is an an-
notation earned: Using fully synthetic training for ob-
ject detection. In 2019 IEEE/CVF International Con-
ference on Computer Vision Workshops, ICCV Work-
shops 2019, Seoul, Korea (South), October 27-28,
2019, pages 2787–2796. IEEE.
Li, Y., Wang, W., Li, X., Xie, L., Wang, Y., Guo, R., Xiu,
W., and Tang, S. (2019). Pole-like street furniture seg-
mentation and classification in mobile lidar data by
integrating multiple shape-descriptor constraints. Re-
mote Sensing, 11(24).
Liu, Z., Lin, Y., Cao, Y., Hu, H., Wei, Y., Zhang, Z., Lin,
S., and Guo, B. (2021). Swin transformer: Hierarchi-
cal vision transformer using shifted windows. In Pro-
ceedings of the IEEE/CVF International Conference
on Computer Vision (ICCV).
Makoviychuk, V., Wawrzyniak, L., Guo, Y., Lu, M.,
Storey, K., Macklin, M., Hoeller, D., Rudin, N., All-
shire, A., Handa, A., and State, G. (2021). Isaac
Gym: High Performance GPU-Based Physics Sim-
ulation For Robot Learning. arXiv e-prints, page
arXiv:2108.10470.
Neuhold, G., Ollmann, T., Rota Bulo, S., and Kontschieder,
P. (2017). The mapillary vistas dataset for semantic
understanding of street scenes. In Proceedings of the
IEEE International Conference on Computer Vision
(ICCV).
NVidia (2022). Omniverse. https://developer.nvidia.com/
nvidia-omniverse/. [Online; accessed 26-July-2022].
Rezapour, M. and Ksaibati, K. (2021). Convolutional neu-
ral network for roadside barriers detection: Trans-
fer learning versus non-transfer learning. Signals,
2(1):72–86.
Sainju, A. M. and Jiang, Z. (2020). Mapping road safety
features from streetview imagery: A deep learning ap-
proach. Trans. Data Sci., 1(3):15:1–15:20.
Shotton, J., Johnson, M., and Cipolla, R. (2008). Semantic
texton forests for image categorization and segmenta-
tion. In 2008 IEEE Conference on Computer Vision
and Pattern Recognition, pages 1–8.
Smith, V., Malik, J., and Culler, D. (2013). Classification of
sidewalks in street view images. In 2013 International
Green Computing Conference Proceedings, pages 1–
6.
Stereolabs (last accessed July 28, 2022)). Stereolabs. https:
//www.stereolabs.com/zed-2/.
Tremblay, J., Prakash, A., Acuna, D., Brophy, M., Jam-
pani, V., Anil, C., To, T., Cameracci, E., Boochoon,
S., and Birchfield, S. (2018). Training deep networks
with synthetic data: Bridging the reality gap by do-
main randomization. In 2018 IEEE Conference on
Computer Vision and Pattern Recognition Workshops,
CVPR Workshops 2018, Salt Lake City, UT, USA, June
18-22, 2018, pages 969–977. Computer Vision Foun-
dation / IEEE Computer Society.
Verma, B. K., Zhang, L., and Stockwell, D. R. B. (2017).
Roadside Video Data Analysis: Deep Learning - Deep
Learning, volume 711 of Studies in Computational In-
telligence. Springer.
Xu, H. and Zhang, J. (2020). Aanet: Adaptive aggregation
network for efficient stereo matching. In Proceedings
of the IEEE/CVF Conference on Computer Vision and
Pattern Recognition, pages 1959–1968.
Zhao, H., Qi, X., Shen, X., Shi, J., and Jia, J. (2018).
ICNet for real-time semantic segmentation on high-
resolution images. In ECCV.
Zhao, Y., Gong, S., Gao, X., Ai, W., and Zhu, S.
(2022). Vrkitchen2.0-indoorkit: A tutorial for aug-
mented indoor scene building in omniverse. CoRR,
abs/2206.11887.
RGB-D Structural Classification of Guardrails via Learning from Synthetic Data
453