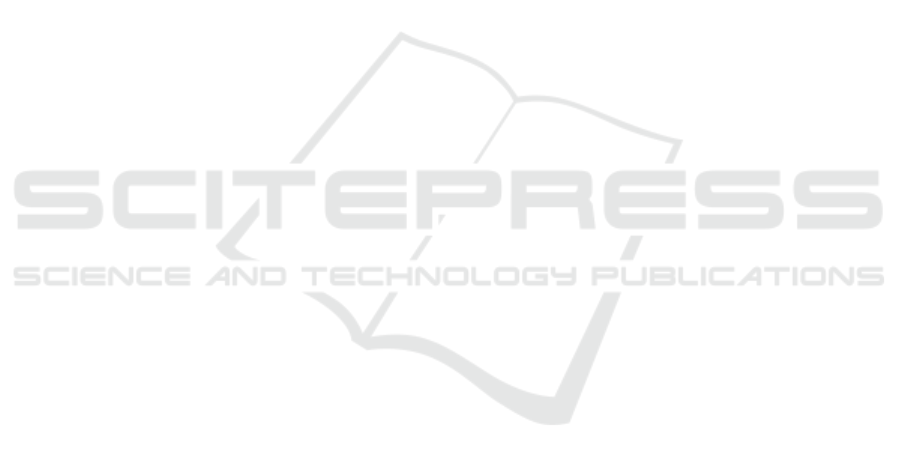
5 CONCLUSION
The use of food recommendation systems has sig-
nificantly increased in online food services in recent
years, aiming at providing users with personalized
food recommendations. In this paper, a novel ex-
plainable and health-aware food recommender system
is developed and its performance is investigated on
the real-world food social network. In terms of Pre-
cision, Recall, F1, AUC, and NDCG, the developed
EHFRS performed significantly better than state-of-
the-art food recommendation models.
In addition, traditional food recommendation
models generally focus on single-user scenarios, how-
ever, most real-life interactions take place in groups,
In future, we plan to developed food group recom-
mendation models. In addition, in future, we aim to
enhance the performance of the food recommendation
by incorporating additional user information.
ACKNOWLEDGEMENTS
The project is supported by the Academy of Fin-
land (project number 326291) and the University of
Oulu Academy of Finland Profi5 on Digihealth. This
work also was supported in part by the Ministry of
Education and Culture, Finland (OKM/20/626/2022).
Moreover, SA was supported by the Kermanshah
University of Technology, Iran, under grant number
S/P/F/5.
REFERENCES
Ahmadian, M., Ahmadi, M., and Ahmadian, S. (2022a). A
reliable deep representation learning to improve trust-
aware recommendation systems. Expert Systems with
Applications, 197:116697.
Ahmadian, S., Joorabloo, N., Jalili, M., and Ahmadian, M.
(2022b). Alleviating data sparsity problem in time-
aware recommender systems using a reliable rating
profile enrichment approach. Expert Systems with Ap-
plications, 187:115849.
Asani, E., Vahdat-Nejad, H., and Sadri, J. (2021). Restau-
rant recommender system based on sentiment analy-
sis. Machine Learning with Applications, 6:100114.
Bianchini, D., De Antonellis, V., De Franceschi, N., and
Melchiori, M. (2017). Prefer: A prescription-based
food recommender system. Computer Standards &
Interfaces, 54:64–75.
Chavan, P., Thoms, B., and Isaacs, J. (2021). A recom-
mender system for healthy food choices: Building a
hybrid model for recipe recommendations using big
data sets. In Proceedings of the 54th Hawaii Interna-
tional Conference on System Sciences, page 3774.
Chen, M., Jia, X., Gorbonos, E., Hoang, C. T., Yu, X., and
Liu, Y. (2020). Eating healthier: Exploring nutrition
information for healthier recipe recommendation. In-
formation Processing & Management, 57(6):102051.
Elsweiler, D., Trattner, C., and Harvey, M. (2017). Exploit-
ing food choice biases for healthier recipe recommen-
dation. In Proceedings of the 40th international acm
sigir conference on research and development in in-
formation retrieval, pages 575–584.
Forouzandeh, S. and Aghdam, A. R. (2019). Health rec-
ommender system in social networks: A case of face-
book. Webology, 16(1).
Gao, X., Feng, F., He, X., Huang, H., Guan, X., Feng, C.,
Ming, Z., and Chua, T.-S. (2019). Hierarchical at-
tention network for visually-aware food recommenda-
tion. IEEE Transactions on Multimedia, 22(6):1647–
1659.
Gao, X., Feng, F., Huang, H., Mao, X.-L., Lan, T., and Chi,
Z. (2022). Food recommendation with graph convolu-
tional network. Information Sciences, 584:170–183.
Ghosh, P., Bhattacharjee, D., and Nasipuri, M. (2021). Dy-
namic diet planner: A personal diet recommender sys-
tem based on daily activity and physical condition.
IRBM, 42(6):442–456.
Lawlor, D. A. and Pearce, N. (2013). The vienna declaration
on nutrition and non-communicable diseases.
Meng, L., Feng, F., He, X., Gao, X., and Chua, T.-S. (2020).
Heterogeneous fusion of semantic and collaborative
information for visually-aware food recommendation.
In Proceedings of the 28th ACM International Confer-
ence on Multimedia, pages 3460–3468.
Organization, W. H. et al. (2007). Population nutrient in-
take goals for preventing diet-related chronic diseases.
http://www. who. int/nutrition/topics/.
Organization, W. H. et al. (2014). Global status re-
port on noncommunicable diseases 2014. Number
WHO/NMH/NVI/15.1. World Health Organization.
Rostami, M. and Oussalah, M. (2022a). Cancer predic-
tion using graph-based gene selection and explainable
classifier. Finnish Journal of eHealth and eWelfare,
14(1):61–78.
Rostami, M. and Oussalah, M. (2022b). A novel explain-
able covid-19 diagnosis method by integration of fea-
ture selection with random forest. Informatics in
Medicine Unlocked, 30:100941.
Shabanabegum, S., Anusha, P., Seethalakshmi, E., Shun-
mugam, M., Vadivukkarasi, K., and Vijayakumar, P.
(2020). Iot enabled food recommender with nir sys-
tem. Materials Today: Proceedings.
Shaikh, S. G., Suresh Kumar, B., and Narang, G. (2022).
Recommender system for health care analysis using
machine learning technique: a review. Theoretical Is-
sues in Ergonomics Science, pages 1–30.
Tian, Y., Zhang, C., Metoyer, R., and Chawla, N. V. (2021).
Recipe recommendation with hierarchical graph at-
tention network. Frontiers in Big Data, 4:1–13.
Wang, W., Duan, L.-Y., Jiang, H., Jing, P., Song, X., and
Nie, L. (2021). Market2dish: Health-aware food
recommendation. ACM Transactions on Multime-
dia Computing, Communications, and Applications
(TOMM), 17(1):1–19.
A Novel Explainable and Health-aware Food Recommender System
215