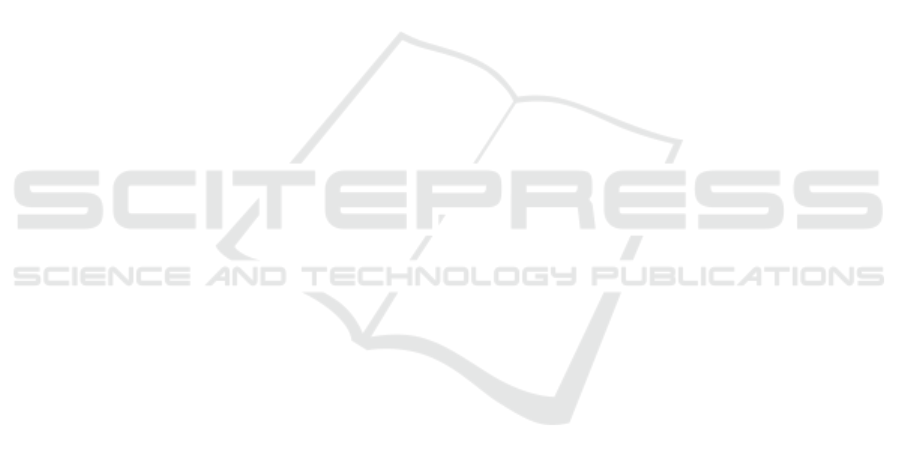
ligence and Lecture Notes in Bioinformatics), 7577
LNCS(PART 6):611–625.
Eigen, D. and Fergus, R. (2015). Predicting depth, surface
normals and semantic labels with a common multi-
scale convolutional architecture. Proceedings of the
IEEE International Conference on Computer Vision,
2015 Inter:2650–2658.
Kim, D., Lee, S., Lee, J., and Kim, J. (2020). Leveraging
Contextual Information for Monocular Depth Estima-
tion. IEEE Access, 8:147808–147817.
Kingma, D. P. and Ba, J. L. (2015). Adam: A method for
stochastic optimization. 3rd International Conference
on Learning Representations, ICLR 2015 - Confer-
ence Track Proceedings, pages 1–15.
Laina, I., Rupprecht, C., Belagiannis, V., Tombari, F., and
Navab, N. (2016). Deeper depth prediction with fully
convolutional residual networks. Proceedings - 2016
4th International Conference on 3D Vision, 3DV 2016,
pages 239–248.
Lee, J. H., Han, M.-K., Ko, D. W., and Suh, I. H. (2019).
From big to small: Multi-scale local planar guid-
ance for monocular depth estimation. arXiv preprint
arXiv:1907.10326.
Li, B., Shen, C., Dai, Y., van den Hengel, A., and He, M.
(2015). Depth and surface normal estimation from
monocular images using regression on deep features
and hierarchical crfs. In 2015 IEEE Conference on
Computer Vision and Pattern Recognition (CVPR),
pages 1119–1127.
Lin, L., Liu, Y., Hu, Y., Yan, X., Xie, K., and Huang, H.
(2022). Capturing, reconstructing, and simulating: the
urbanscene3d dataset. In ECCV.
Liu, Y. and Huang, H. (2021). Virtualcity3d: A large scale
urban scene dataset and simulator.
Marcu, A., Costea, D., Lic
˘
aret¸, V., P
ˆ
ırvu, M., Slus¸anschi,
E., and Leordeanu, M. (2019). SafeUAV: learning to
estimate depth and safe landing areas for UAVs from
synthetic data. Lecture Notes in Computer Science
(including subseries Lecture Notes in Artificial Intel-
ligence and Lecture Notes in Bioinformatics), 11130
LNCS:43–58.
Mayer, N., Ilg, E., Hausser, P., Fischer, P., Cremers, D.,
Dosovitskiy, A., and Brox, T. (2016). A Large Dataset
to Train Convolutional Networks for Disparity, Opti-
cal Flow, and Scene Flow Estimation. Proceedings of
the IEEE Computer Society Conference on Computer
Vision and Pattern Recognition, 2016-Decem:4040–
4048.
Qi, X., Liu, Z., Liao, R., Torr, P. H., Urtasun, R., and Jia, J.
(2020). GeoNet++: Iterative Geometric Neural Net-
work with Edge-Aware Refinement for Joint Depth
and Surface Normal Estimation. IEEE Transactions
on Pattern Analysis and Machine Intelligence, pages
1–1.
Sekkat, A. R., Dupuis, Y., Kumar, V. R., Rashed, H., Yo-
gamani, S., Vasseur, P., and Honeine, P. (2022). Syn-
woodscape: Synthetic surround-view fisheye camera
dataset for autonomous driving. IEEE Robotics and
Automation Letters, 7(3):8502–8509.
Shah, S., Dey, D., Lovett, C., and Kapoor, A. (2017). Air-
sim: High-fidelity visual and physical simulation for
autonomous vehicles. In Field and Service Robotics.
Wang, X., Fouhey, D. F., and Gupta, A. (2015). Designing
deep networks for surface normal estimation. Pro-
ceedings of the IEEE Computer Society Conference
on Computer Vision and Pattern Recognition, 07-12-
June(Figure 2):539–547.
Wang, Z., Bovik, A. C., Sheikh, H. R., and Simoncelli, E. P.
(2004). Image quality assessment: From error visi-
bility to structural similarity. IEEE Transactions on
Image Processing, 13(4):600–612.
Yang, Z., Wang, P., Xu, W., Zhao, L., and Neva-
tia, R. (2018). Unsupervised learning of geometry
from videos with edge-aware depth-normal consis-
tency. 32nd AAAI Conference on Artificial Intelli-
gence, AAAI 2018, 1(c):7493–7500.
Yin, W., Liu, Y., Shen, C., and Yan, Y. (2019). Enforcing
geometric constraints of virtual normal for depth pre-
diction. Proceedings of the IEEE International Con-
ference on Computer Vision, 2019-Octob:5683–5692.
Zhan, H., Weerasekera, C. S., Garg, R., and Reid, I. (2019).
Self-supervised learning for single view depth and
surface normal estimation. Proceedings - IEEE In-
ternational Conference on Robotics and Automation,
2019-May:4811–4817.
Zhang, H., Shen, C., Li, Y., Cao, Y., Liu, Y., and Yan,
Y. (2019). Exploiting temporal consistency for real-
time video depth estimation. Proceedings of the IEEE
International Conference on Computer Vision, 2019-
Octob:1725–1734.
Zhu, P., Wen, L., Bian, X., Ling, H., and Hu, Q. (2018).
Vision Meets Drones: A Challenge. pages 1–11.
ROBOVIS 2022 - Workshop on Robotics, Computer Vision and Intelligent Systems
462