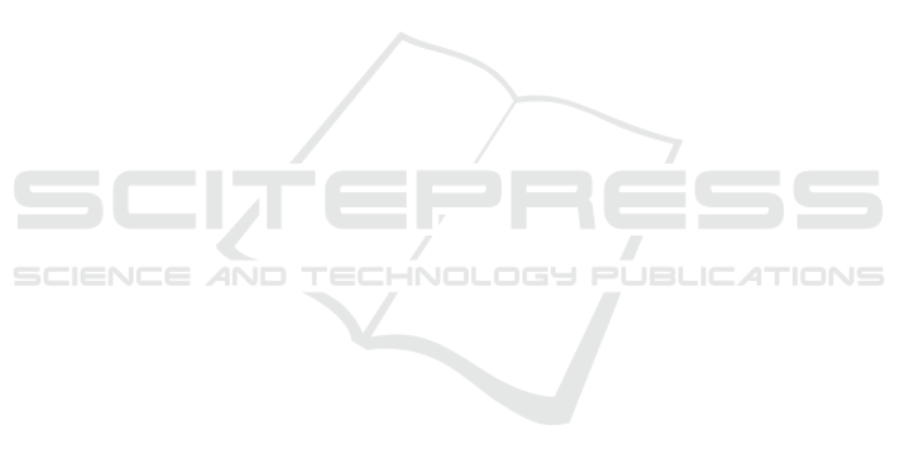
ACKNOWLEDGEMENTS
This work was conducted under the research project
“Machine Learning based Breast Cancer Diagnosis
and Treatment”, 2020-2023. The authors would like
to thank the Moroccan Ministry of Higher Education
and Scientific Research, Digital Development Agency
(ADD), CNRST, and UM6P for their support.
REFERENCES
Debelee, T. G., Kebede, S. R., Schwenker, F., and She-
warega, Z. M. (2020). Deep Learning in Selected Can-
cers’ Image Analysis—A Survey. Journal of Imaging
2020, Vol. 6, Page 121, 6(11):121.
Dong, N., Zhao, L., Wu, C., and Chang, J. (2020). Inception
v3 based cervical cell classification combined with ar-
tificially extracted features. Applied Soft Computing,
93:106311.
Emerson, P. and Emerson, P. (2011). The original Borda
count and partial voting. Social Choice and Welfare
2011 40:2, 40(2):353–358.
Eun, T. J. and Perkins, R. B. (2020). Screening for Cer-
vical Cancer. The Medical clinics of North America,
104(6):1063.
Fei-Fei, L., Deng, J., and Li, K. (2009). ImageNet: Con-
structing a large-scale image database. Journal of Vi-
sion, 9(8):1037–1037.
Gravitt, P. E., Silver, M. I., Hussey, H. M., Arrossi, S.,
Huchko, M., Jeronimo, J., Kapambwe, S., Kumar, S.,
Meza, G., Nervi, L., Paz-Soldan, V. A., and Woo, Y. L.
(2021). Achieving equity in cervical cancer screen-
ing in low- and middle-income countries (LMICs):
Strengthening health systems using a systems think-
ing approach. Preventive Medicine, 144:106322.
Hussain, E., Mahanta, L. B., Borah, H., and Das, C. R.
(2020). Liquid based-cytology Pap smear dataset for
automated multi-class diagnosis of pre-cancerous and
cervical cancer lesions. Data in Brief, 30:105589.
Idlahcen, F., Himmi, M. M., and Mahmoudi, A. (2020).
CNN-based Approach for Cervical Cancer Classifica-
tion in Whole-Slide Histopathology Images.
Idlahcen, F. and Idri, A. (2022). Systematic Map of Data
Mining for Gynecologic Oncology. pages 466–475.
Idri, A. and Abnane, I. (2017). Fuzzy Analogy Based Effort
Estimation: An Empirical Comparative Study. IEEE
CIT 2017 - 17th IEEE International Conference on
Computer and Information Technology, pages 114–
121.
Idri, A., Abnane, I., and Abran, A. (2018). Evaluating
Pred(p) and standardized accuracy criteria in software
development effort estimation. Journal of Software:
Evolution and Process, 30(4):e1925.
Idri, A., Hosni, M., and Abran, A. (2016). Improved esti-
mation of software development effort using Classical
and Fuzzy Analogy ensembles. Applied Soft Comput-
ing, 49:990–1019.
Kurnianingsih, Allehaibi, K. H. S., Nugroho, L. E.,
Widyawan, Lazuardi, L., Prabuwono, A. S., and Man-
toro, T. (2019). Segmentation and classification of
cervical cells using deep learning. IEEE Access,
7:116925–116941.
Laengsri, V., Kerdpin, U., Plabplueng, C., Treeratanapi-
boon, L., and Nuchnoi, P. (2018). Cervical Cancer
Markers: Epigenetics and microRNAs. Lab Medicine,
49(2):97–111.
Lin, H., Hu, Y., Chen, S., Yao, J., and Zhang, L. (2019).
Fine-grained classification of cervical cells using mor-
phological and appearance based convolutional neural
networks. IEEE Access, 7:71541–71549.
Mart
´
ınez-M
´
as, J., Bueno-Crespo, A., Khazendar, S.,
Remezal-Solano, M., Mart
´
ınez-Cend
´
an, J. P., Jassim,
S., Du, H., Assam, H. A., Bourne, T., and Timmer-
man, D. (2019). Evaluation of machine learning meth-
ods with Fourier Transform features for classifying
ovarian tumors based on ultrasound images. PLOS
ONE, 14(7):e0219388.
Ottoni, A. L., Nepomuceno, E. G., de Oliveira, M. S.,
and de Oliveira, D. C. (2019). Tuning of reinforce-
ment learning parameters applied to SOP using the
Scott–Knott method. Soft Computing, 24(6):4441–
4453.
Promworn, Y., Pattanasak, S., Pintavirooj, C., and Piyawat-
tanametha, W. (2019). Comparisons of pap smear
classification with deep learning models. Proceed-
ings of the 14th Annual IEEE International Confer-
ence on Nano/Micro Engineered and Molecular Sys-
tems, NEMS 2019, pages 282–285.
Singh, J. and Sharma, S. (2019). Prediction of Cervical
Cancer Using Machine Learning Techniques. Inter-
national Journal of Applied Engineering Research,
14(11):2570–2577.
Sung, H., Ferlay, J., Siegel, R. L., Laversanne, M., Soer-
jomataram, I., Jemal, A., and Bray, F. (2021). Global
Cancer Statistics 2020: GLOBOCAN Estimates of In-
cidence and Mortality Worldwide for 36 Cancers in
185 Countries. CA: a cancer journal for clinicians,
71(3):209–249.
Tainio, K., Athanasiou, A., Tikkinen, K. A., Aaltonen, R.,
C
´
ardenas, J., Hern
´
andes, Glazer-Livson, S., Jakobs-
son, M., Joronen, K., Kiviharju, M., Louvanto, K.,
Oksjoki, S., T
¨
ahtinen, R., Virtanen, S., Nieminen, P.,
Kyrgiou, M., and Kalliala, I. (2018). Clinical course
of untreated cervical intraepithelial neoplasia grade
2 under active surveillance: systematic review and
meta-analysis. The BMJ, 360.
Taqi, S. A., Sami, S. A., Sami, L. B., and Zaki, S. A.
(2018). A review of artifacts in histopathology. Jour-
nal of Oral and Maxillofacial Pathology : JOMFP,
22(2):279.
Wilailak, S., Kengsakul, M., and Kehoe, S. (2021). World-
wide initiatives to eliminate cervical cancer. Interna-
tional journal of gynaecology and obstetrics: the of-
ficial organ of the International Federation of Gynae-
cology and Obstetrics, 155 Suppl 1(S1):102–106.
Whole-slide Classification of HE-stained Cervix Uteri Tissue using Deep Neural Networks
329