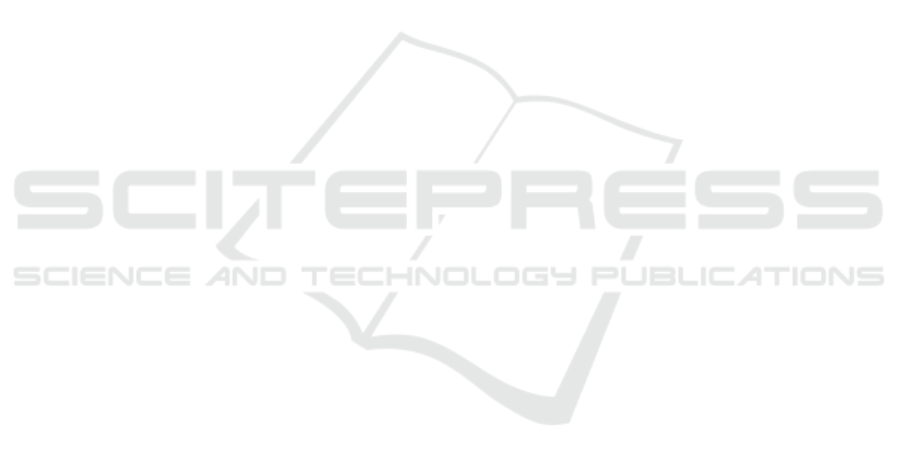
pitfall here is twofold: some pieces of information can
be circulated intact to avoid miscommunicating them
to readers. But what happens if the source of the arti-
cle failed to validate facts or if the author of the arti-
cle is biased? Surely the news media would not want
their brand name to be harmed, by transferring mis-
informative articles. Readers on the other hand, can
easily fall for propaganda or different forms of fake
news, something that can greatly affect social coher-
ence.
5 DISCUSSION AND FUTURE
WORK
In this research the degree of similarity between news
articles, published by different news media, was ex-
plored. In other words, we tried to inspect how dif-
ferently online news media describe news events. To
establish a quantified understanding of that similarity,
three different datasets were created. The first con-
tains unrelated event news, the second related event
news, while the third, same event news articles.
The underlying goal of this task, is to define sim-
ilarity limits for each set, to be able to put findings
in context and finally compare them. Special focus
is given in examining the similarity, between same
events news articles. Even though results are diverse,
in some cases they support our argument; high simi-
larity scores reveal that many news articles, are iden-
tical and/or similar.
This fact suggests that news media fail to inves-
tigate events and describe them in an original way.
Instead, groups of media are formed that circulate
nearly identical piece of information to their readers.
The methodology followed can surely be im-
proved. Even though tfidf and cosine similarity per-
form well, there are numerous representations and
measurement algorithms to consider for feature tasks.
Also, we would like expand this study by collecting
more data, and including additional factors such as
topic models, named entities, and comparison with
other languages. This research contributes in current
literature as there is no relevant research, that exam-
ines the similarities of news articles in Greek, which
is a low-resource language.
ACKNOWLEDGEMENTS
This research is supported by the University of West
Attica.
REFERENCES
Ahmed, S. S. and Hanif, U. (2020). News Recommendation
Algorithm Based on Deep Learning. 06:9.
AL-Smadi, M., Jaradat, Z., AL-Ayyoub, M., and Jararweh,
Y. (2017). Paraphrase identification and semantic text
similarity analysis in Arabic news tweets using lexi-
cal, syntactic, and semantic features. Information Pro-
cessing & Management, 53(3):640–652.
Antonopoulos, N., Konidaris, A., Polykalas, S., and Lam-
prou, E. (2020a). Online Journalism: Crowdsourcing,
and Media Websites in an Era of Participation. Studies
in Media and Communication, 8(1):25.
Antonopoulos, N., Lamprou, E., Kiourexidou, M.,
Konidaris, A., and Polykalas, S. (2020b). Media Web-
sites Services and Users Subscription Models for On-
line Journalism. Media Watch, 11(2).
Bright, J. and Nicholls, T. (2014). The Life and Death of
Political News: Measuring the Impact of the Audience
Agenda Using Online Data. Social Science Computer
Review, 32(2):170–181. Publisher: SAGE Publica-
tions Inc.
Dhyani, D., Ng, W. K., and Bhowmick, S. S. (2002). A
survey of Web metrics. ACM Computing Surveys,
34(4):469–503.
Fan, A., Doshi-Velez, F., and Miratrix, L. (2017). Prior mat-
ters: simple and general methods for evaluating and
improving topic quality in topic modeling. Technical
Report arXiv:1701.03227, arXiv. arXiv:1701.03227
[cs] type: article.
Feng, C., Khan, M., Rahman, A. U., and Ahmad, A.
(2020). News recommendation systems - accomplish-
ments, challenges & future directions. IEEE Access,
8:16702–16725.
Hamborg, F., Donnay, K., and Gipp, B. (2019). Automated
identification of media bias in news articles: an inter-
disciplinary literature review. International Journal
on Digital Libraries, 20(4):391–415.
H.Gomaa, W. and A. Fahmy, A. (2013). A Survey of Text
Similarity Approaches. International Journal of Com-
puter Applications, 68(13):13–18.
Hu, Y., Xin, G., Song, R., Hu, G., Shi, S., Cao, Y., and
Li, H. (2005). Title extraction from bodies of HTML
documents and its application to web page retrieval.
In Proceedings of the 28th annual international ACM
SIGIR conference on Research and development in in-
formation retrieval - SIGIR ’05, page 250, Salvador,
Brazil. ACM Press.
Huang, A.-L. (2008). Similarity measures for text document
clustering.
Ibrahim, H., Darwish, K., and Abdel-sabor, A.-R. Auto-
matic Extraction of Textual Elements from News Web
Pages. page 5.
Leandros, N. and Papadopoulou, L. (2020). Creative de-
struction in the Greek media landscape: New and al-
ternative business models. In Vovou, I., Andonova, Y.
and Kogan, A.F. (Eds), Proceedings of The Creative
Contagion. The creative contagion. Media, industries,
storytelling, communities, pp. 89-97., pages 89–97.
A Text Similarity Study: Understanding How Differently Greek News Media Describe News Events
251