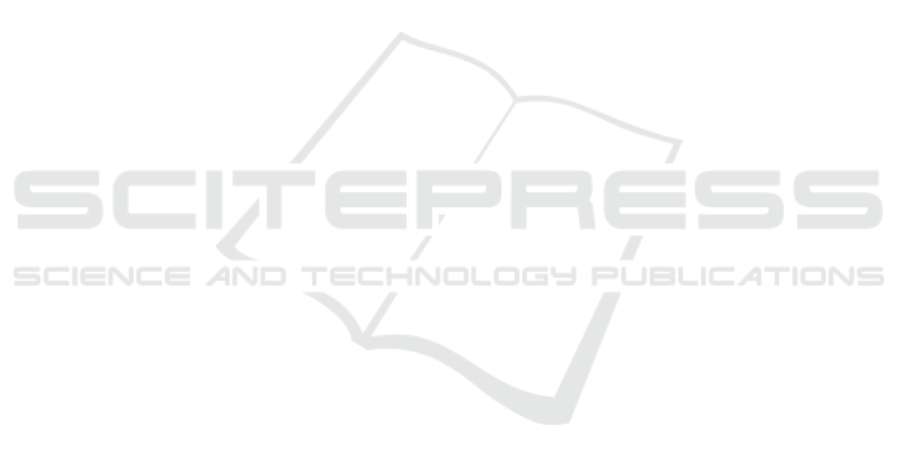
tion that allows either taxonomic (e.g., OWL) or
more generic (e.g., FOL) representations. As stip-
ulated in the PCTO guidelines, the learning path
is evaluated at precise points of the activities to be
performed and also takes into account the moods
of the learners useful for monitoring the learning
progress. Since the semantics of all this informa-
tion can be interpreted by GraphBRAIN, anoma-
lous situations can be detected applying the multi-
strategic reasoning (Ferilli and Redavid, 2020b).
In this case particular information will be com-
municated to KEPLAIR that will modify the sug-
gested learning path. As consequence, the ma-
terial that was suggested to the learners and ap-
proved by the teachers can be modified in order
to improve the learning outcome (e.g. if Graph-
BRAIN detects a certain discomfort in the student
since supplementary material in Maths has been
assigned than KEPLAIR suggests alternative sup-
plementary material). In addition, the supervi-
sion functionality offered by WoMan will make
it possible to assess whether the specific student
is correctly following the process formalised for
him/her in the previous phase. Through this mon-
itoring, corrective actions can be taken in accor-
dance with the tutor in order to have a better result
of the student’s learning performance.
5 CONCLUSIONS AND FUTURE
WORKS
The ITS meta-analytic review presented in (Kulik and
Fletcher, 2016) has proved their efficiency with re-
spect to other forms of tutoring. As the authors them-
selves indicate, it is not easy to determine what the
next generation of ITS will look like, but they will cer-
tainly be influenced by three factors: computer hard-
ware, software, networking, and cognitive science. In
this paper we have outlined a possible framework that
can also handle the moods represented by learning
processes formalised in the WoMan formalism. Fur-
thermore, through GraphBRAIN it will be possible
to handle different knowledge representations and ap-
ply multi-strategic reasoning in order to improve stu-
dents’ learning performance. In addition, by using
KEPLAIR we are able to cover one of the fundamen-
tal requirements of PCTO: empowering students by
allowing them to create proposals for learning paths.
As future work, a comparison with realities outside
the European community is desirable. In particular,
we have planned to test the platform in the Ukrainian
context with the support of the T.H. Shevchenko Na-
tional University ”Chernihiv Colehium”. On the one
hand, the sharing of EU recommendations can be
an opportunity to bring these two realities closer to-
gether; on the other hand, the current difficult situa-
tion requires a massive use of online tools for school
education. Also if any e-learning has different goals
(Matviichuk et al., 2017), the deeper use of modern
technologies, namely elements of the artificial intelli-
gence (AI) proposed in this work, could be reveal new
modality to use an intelligent learning system (ITS).
ACKNOWLEDGEMENTS
This work is partially founded by the Apulia Region
through the ‘Research for Innovation—REFIN’ ini-
tiative [grant number 48B6D67E].
REFERENCES
Abaalkhail, R., Guthier, B., Alharthi, R., and El-Saddik, A.
(2018). Survey on ontologies for affective states and
their influences. Semantic Web, 9(4):441–458.
Bolock, A. E., Abdennadher, S., and Herbert, C. (2021). An
ontology-based framework for psychological monitor-
ing in education during the covid-19 pandemic. Fron-
tiers in Psychology, 12.
Ferilli, S. (2014). Woman: Logic-based workflow learn-
ing and management. IEEE Transactions on Systems,
Man, and Cybernetics: Systems, 44(6):744–756.
Ferilli, S., Esposito, F., Redavid, D., and Angelastro, S.
(2017). Extended process models for activity predic-
tion. In Kryszkiewicz, M., Appice, A.,
´
Sl
´
ezak, D., Ry-
binski, H., Skowron, A., and Ra
´
s, Z. W., editors, Foun-
dations of Intelligent Systems, pages 368–377, Cham.
Springer International Publishing.
Ferilli, S. and Redavid, D. (2020a). The graphbrain sys-
tem for knowledge graph management and advanced
fruition. In Helic, D., Leitner, G., Stettinger, M.,
Felfernig, A., and Ra
´
s, Z. W., editors, Foundations of
Intelligent Systems, pages 308–317, Cham. Springer
International Publishing.
Ferilli, S. and Redavid, D. (2020b). An ontology and knowl-
edge graph infrastructure for digital library knowledge
representation. In Ceci, M., Ferilli, S., and Poggi, A.,
editors, Digital Libraries: The Era of Big Data and
Data Science, pages 47–61, Cham. Springer Interna-
tional Publishing.
Ferilli, S., Redavid, D., Di Pierro, D., and Loop, L. (2022).
An ontology-driven architecture for intelligent tutor-
ing systems with an application to learning object rec-
ommendation. International Journal of Computer In-
formation Systems and Industrial Management Appli-
cations, 14:297–312.
Ferilli, S., Redavid, D., Pierro, D. D., and Loop, L. (2021).
Functionality and architecture for a platform for in-
dependent learners: KEPLAIR. In Abraham, A.,
KEOD 2022 - 14th International Conference on Knowledge Engineering and Ontology Development
258