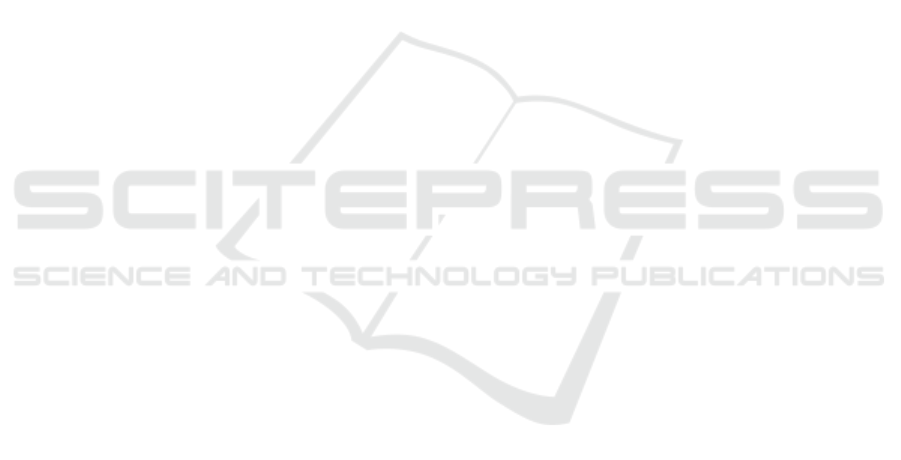
REFERENCES
Akbani, R., Kwek, S., and Japkowicz, N. (2004). Applying
support vector machines to imbalanced datasets. In
European conference on machine learning, pages 39–
50. Springer.
Arrieta, A. B., D
´
ıaz-Rodr
´
ıguez, N., Del Ser, J., Bennetot,
A., Tabik, S., Barbado, A., Garc
´
ıa, S., Gil-L
´
opez, S.,
Molina, D., Benjamins, R., et al. (2020). Explainable
artificial intelligence (xai): Concepts, taxonomies, op-
portunities and challenges toward responsible ai. In-
formation fusion, 58:82–115.
Bongartz, S., Jin, Y., Patern
`
o, F., Rett, J., Santoro, C., and
Spano, L. D. (2012). Adaptive user interfaces for
smart environments with the support of model-based
languages. In International Joint Conference on Am-
bient Intelligence, pages 33–48. Springer.
Chawla, N. V., Bowyer, K. W., Hall, L. O., and Kegelmeyer,
W. P. (2002). Smote: synthetic minority over-
sampling technique. Journal of artificial intelligence
research, 16:321–357.
Chen, Z. and Liu, B. (2018). Lifelong machine learning.
Synthesis Lectures on Artificial Intelligence and Ma-
chine Learning, 12(3):1–207.
Fan, H. and Poole, M. S. (2006). What is personaliza-
tion? perspectives on the design and implementation
of personalization in information systems. Journal of
Organizational Computing and Electronic Commerce,
16(3-4):179–202.
Hasenj
¨
ager, M., Heckmann, M., and Wersing, H. (2019).
A survey of personalization for advanced driver assis-
tance systems. IEEE Transactions on Intelligent Vehi-
cles, 5(2):335–344.
Hussain, J., Ul Hassan, A., Muhammad Bilal, H. S., Ali, R.,
Afzal, M., Hussain, S., Bang, J., Banos, O., and Lee,
S. (2018). Model-based adaptive user interface based
on context and user experience evaluation. Journal on
Multimodal User Interfaces, 12(1):1–16.
Khan, I. and Khusro, S. (2020). Towards the design of
context-aware adaptive user interfaces to minimize
drivers’ distractions. Mobile Information Systems,
2020.
Krause, A. F., Essig, K., Piefke, M., and Schack, T.
(2013). No-prop-fast-a high-speed multilayer neu-
ral network learning algorithm: mnist benchmark and
eye-tracking data classification. In International Con-
ference on Engineering Applications of Neural Net-
works, pages 446–455. Springer.
Kural, K., Besselink, I., Xu, Y., Tomar, A., and Nijmeijer,
H. (2016). A driver support system for improved ma-
neuvering of articulated vehicles using an unmanned
aerial vehicle. In HVTT14: International Sympo-
sium on Heavy Vehicle Transport Technology, Ro-
torua, New Zealand.
Kusumakar, R., Kural, K., Tomar, A. S., and Pyman, B.
(2017). Autonomous parking for articulated vehicles.
HAN University of Applied Science, Netherlands.
Lilis, Y., Zidianakis, E., Partarakis, N., Antona, M., and
Stephanidis, C. (2017). Personalizing hmi elements
in adas using ontology meta-models and rule based
reasoning. In International Conference on Universal
Access in Human-Computer Interaction, pages 383–
401. Springer.
Lin, N., Zong, C., Tomizuka, M., Song, P., Zhang, Z., and
Li, G. (2014). An overview on study of identification
of driver behavior characteristics for automotive con-
trol. Mathematical Problems in Engineering, 2014.
Ribeiro, P., Krause, A. F., Meesters, P., Kural, K., van Kolf-
schoten, J., B
¨
uchner, M.-A., Ohlmann, J., Ressel, C.,
Benders, J., and Essig, K. (2021). A vr truck docking
simulator platform for developing personalized driver
assistance. Applied Sciences, 11(19):8911.
Riener, A., Boll, S., and Kun, A. L. (2016). Automotive
user interfaces in the age of automation (dagstuhl sem-
inar 16262). In Dagstuhl reports, volume 6. Schloss
Dagstuhl-Leibniz-Zentrum fuer Informatik.
Stuerzlinger, W., Chapuis, O., Phillips, D., and Roussel, N.
(2006). User interface fac¸ades: towards fully adapt-
able user interfaces. In Proceedings of the 19th an-
nual ACM symposium on User interface software and
technology, pages 309–318.
Sun, X., Li, J., Tang, P., Zhou, S., Peng, X., Li, H. N., and
Wang, Q. (2020). Exploring personalised autonomous
vehicles to influence user trust. Cognitive Computa-
tion, 12(6):1170–1186.
Todi, K., Bailly, G., Leiva, L., and Oulasvirta, A. (2021).
Adapting user interfaces with model-based reinforce-
ment learning. In Proceedings of the 2021 CHI Con-
ference on Human Factors in Computing Systems,
pages 1–13.
Ulahannan, A., Cain, R., Thompson, S., Skrypchuk, L.,
Mouzakitis, A., Jennings, P., and Birrell, S. (2020).
User expectations of partial driving automation capa-
bilities and their effect on information design prefer-
ences in the vehicle. Applied Ergonomics, 82:102969.
Ulahannan, A., Thompson, S., Jennings, P., and Birrell,
S. (2021). Using glance behaviour to inform the de-
sign of adaptive hmi for partially automated vehicles.
IEEE Transactions on Intelligent Transportation Sys-
tems, 23(5):4877–4892.
Union, I. R. T. (2007). A scientific study “etac” european
truck accident causation: Executive summary and rec-
ommendations.
Vaswani, A., Shazeer, N., Parmar, N., Uszkoreit, J., Jones,
L., Gomez, A. N., Kaiser, Ł., and Polosukhin, I.
(2017). Attention is all you need. Advances in neural
information processing systems, 30.
Wang, W., Xi, J., and Chen, H. (2014). Modeling and rec-
ognizing driver behavior based on driving data: A sur-
vey. Mathematical Problems in Engineering, 2014.
Wang, Y., Yao, Q., Kwok, J. T., and Ni, L. M. (2020). Gen-
eralizing from a few examples: A survey on few-shot
learning. ACM computing surveys (csur), 53(3):1–34.
Toward the Design of Personalised Adaptive Driver Assistance for Truck Docking
137