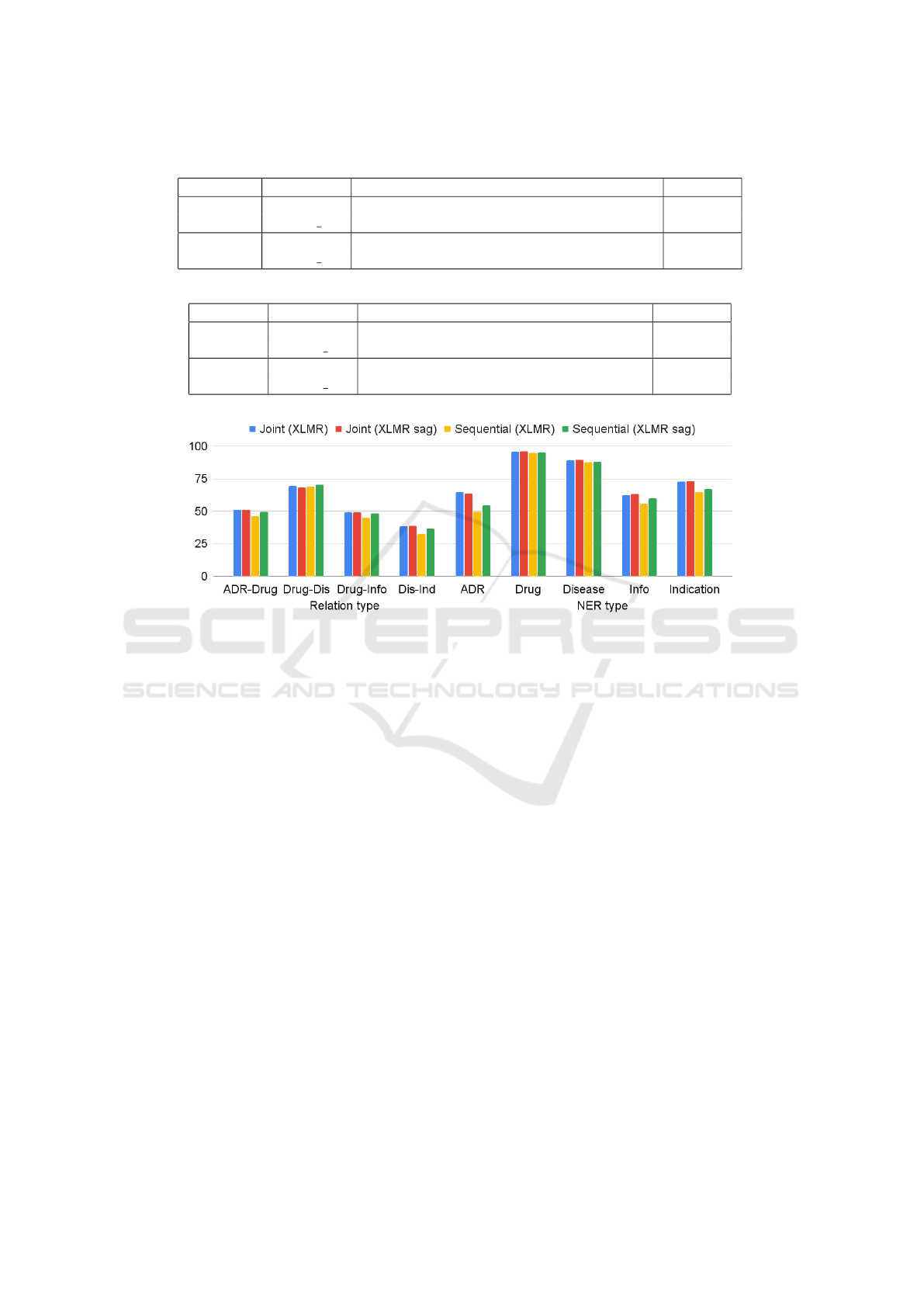
Table 4: Evaluation scores for named relation extraction task. ADR - Adverse Drug Reaction, Drug - Drugname, Dis -
Diseasename, Info - SourceInfoDrug, Ind - Indication
Approach Model ADR-Drug Drug-Dis Drug-Info Dis-Ind f1-macro
Joint XLMR 51.2 69.4 49.2 38.6 52.1
Joint XLMR sag 51.1 68.3 49 38.9 51.8
Sequential XLMR 46.1 69.2 45.1 32.2 48.1
Sequential XLMR sag 49.4 70.4 48.3 36.7 51.2
Table 5: Evaluation scores for named entity recognition task.
Approach Model ADR Drug Disease Info Indication f1-macro
Joint XLMR 64.8 95.7 89.4 62.5 72.9 77.1
Joint XLMR sag 63.8 96.0 89.7 63.3 73.2 77.2
Cascade XLMR 49.6 95.1 87.7 55.6 64.7 70.5
Cascade XLMR sag 54.7 95.3 88.3 60.0 67.2 73.1
Figure 4: Evaluation scores for different language models.
REFERENCES
Devlin, J., Chang, M.-W., Lee, K., and Toutanova, K.
(2018). Bert: Pre-training of deep bidirectional trans-
formers for language understanding. arXiv preprint
arXiv:1810.04805.
Eberts, M. and Ulges, A. (2020). Span-based joint entity
and relation extraction with transformer pre-training.
In ECAI 2020, pages 2006–2013. IOS Press.
Fang, X., Song, Y., and Maeda, A. (2021). Joint extrac-
tion of clinical entities and relations using multi-head
selection method. In 2021 International Conference
on Asian Language Processing (IALP), pages 99–104.
IEEE.
Hamborg, F., Meuschke, N., Breitinger, C., and Gipp, B.
(2017). news-please: A generic news crawler and ex-
tractor. In Proceedings of the 15th International Sym-
posium of Information Science, pages 218–223.
Henry, S., Buchan, K., Filannino, M., Stubbs, A., and
Uzuner, O. (2019). 2018 n2c2 shared task on adverse
drug events and medication extraction in electronic
health records. Journal of the American Medical In-
formatics Association, 27(1):3–12.
Li, F., Zhang, M., Fu, G., and Ji, D. (2017). A neural joint
model for entity and relation extraction from biomed-
ical text. BMC bioinformatics, 18(1):1–11.
Liu, Y., Ott, M., Goyal, N., Du, J., Joshi, M., Chen, D.,
Levy, O., Lewis, M., Zettlemoyer, L., and Stoyanov,
V. (2019). Roberta: A robustly optimized bert pre-
training approach. arXiv preprint arXiv:1907.11692.
Quan, C., Hua, L., Sun, X., and Bai, W. (2016). Multichan-
nel convolutional neural network for biological rela-
tion extraction. BioMed research international, 2016.
Sahu, S. K. and Anand, A. (2018). Drug-drug interac-
tion extraction from biomedical texts using long short-
term memory network. Journal of biomedical infor-
matics, 86:15–24.
Sboev, A., Sboeva, S., Moloshnikov, I., Gryaznov, A., Ry-
bka, R., Naumov, A., Selivanov, A., Rylkov, G., and
Ilyin, V. (2022). Analysis of the full-size russian cor-
pus of internet drug reviews with complex ner label-
ing using deep learning neural networks and language
models. Applied Sciences, 12(1):491:1–34.
Trinh, T. H. and Le, Q. V. (2018). A simple
method for commonsense reasoning. arXiv preprint
arXiv:1806.02847.
Zhang, T., Lin, H., Ren, Y., Yang, L., Xu, B., Yang, Z.,
Wang, J., and Zhang, Y. (2019). Adverse drug re-
action detection via a multihop self-attention mech-
anism. BMC bioinformatics, 20(1):1–11.
Zhu, Y., Kiros, R., Zemel, R., Salakhutdinov, R., Urtasun,
R., Torralba, A., and Fidler, S. (2015). Aligning books
and movies: Towards story-like visual explanations by
watching movies and reading books. In The IEEE In-
ternational Conference on Computer Vision (ICCV).
An Accuracy Comparison of the Joint and Sequential Approaches for End-to-End Related Named Entities Extraction in the Texts of
Russian-Language Reviews Based on Neural Networks
353