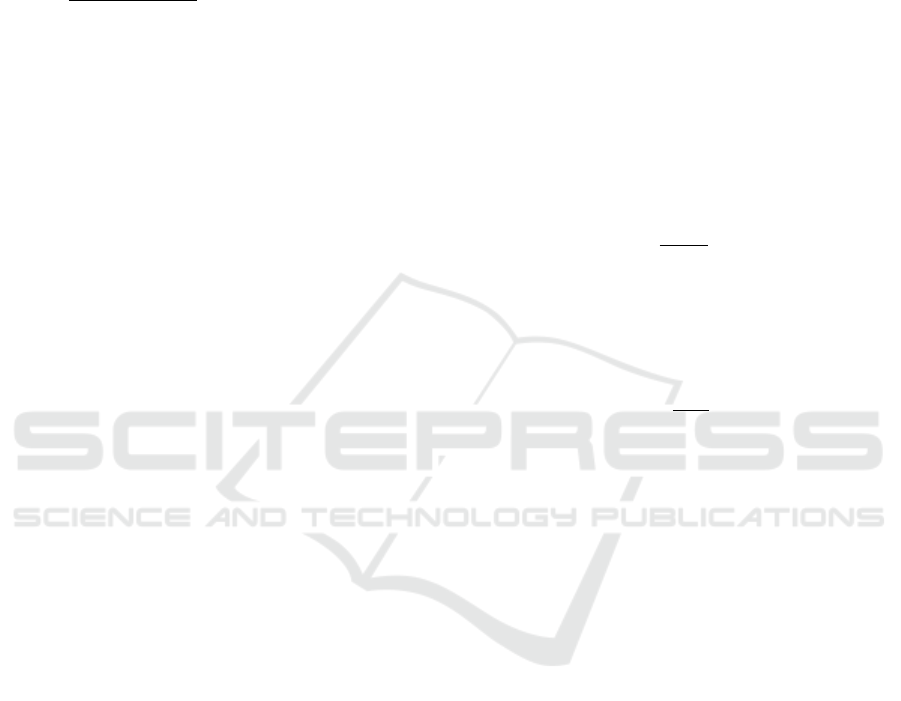
of the implementation of innovations of the informa-
tion potential, which is aimed at increasing the value
of capital and depends on the speed of updating this
strategy. Informational capital and its potential act as
investment capital to maximize the value of intellec-
tual capital:
∑
k
i=1
EVA
ROI
opt
−WACC
−CAPITAL
→ max (4)
where
ROI
opt
is the economic profitability of intellectual
capital;
WACC – weighted average interest rate of the in-
volved intellectual capital;
CAPITAL – the capital of the strategy of attracting
innovations of the information potential.
5. Capital of turning knowledge into a result
The capital of the transformation of knowledge
into a result declares the path of transformations from
an idea to the formalization of knowledge in official
documents and its structuring for communicative use
(Porokhnya, 2009). Therefore, its components are
the following indicators that reflect the characteristic
properties of transformations: an idea as a creative
and spiritual message, and the level of formalization
of knowledge in official documents.
An idea has its own depth of penetration into the
macro or micro world (Roos et al., 2005). Based on
Einstein’s thesis that the development of society re-
quires the improvement of everyday thinking, it is ap-
propriate to consider an idea-concept as a complex of
properties and relationships that determine the char-
acteristics of the image of the object of research. we
can establish a connection between intellectual capital
(figuratively speaking, the mass of intellectual sub-
stance that is at rest or in motion, that is, in its use)
and the strategy of interaction of processes in an eco-
nomic object and its results. The question arises, does
the strategy have energy? It is known that the strategy
has different value, that is, weight. Suppose that, like
any economic potential, it has potential energy, and
when the process of its realization takes place, it also
has kinetic energy. That is, strategy is the energy of
capital that goes to the realization of an idea-concept.
Therefore, it can have its own dimension. Strategy,
like any energy, consists of the energy of rest and the
momentum of intellectual capital. As the speed of this
impulse, we will take the speed of the generation of
an idea-concept in the direction predetermined by the
strategy. To measure images-properties, that is, the
amount of intellectual substance, a unit is introduced,
– image.
Any image of intellectual substance contains the
same number of images-properties that reflect the
properties of the object of the real world. For exam-
ple, the number of images-properties that character-
ize a person is a constant value, a number that can
be established experimentally, as Avogadro’s number
was established at one time (the principle of equiv-
alence in nature). But each person has a different
number of images-relationships characterizing his in-
tellectual capital. This value of images-relationships,
corresponding to intellectual capital, will be assigned
the unit of measurement – intel. Intel measures the
level (mass) of intellectual capital of a person, enter-
prise, state.
The definition of images-properties is a conse-
quence of the same type of process properties during
the realization of an idea-concept in time, which con-
tain a certain number of these images in one unit. We
denote the number of images-properties by N
img
:
N
img
=
100
image
− const (5)
From here we can determine the amount of the
level (mass) of the intellectual capital of the economic
system, which corresponds to the capital of trans-
forming knowledge into a result:
ic =
N
N
img
M
ic
(6)
where
N – the number of images-properties, respectively,
ideas-concepts,
M
ic
- the intellectual mass of image-properties per
image-property for a specific phenomenon, intel / im-
age.
The level of an idea-concept can be represented
in four quantitative measurements with the introduc-
tion of a unit of measurement – id, which contains a
certain integral number of images-objects that char-
acterize the properties of this very idea-concept using
established criteria:
• Elementary level (household, cognitive, which
does not require the formation of new knowl-
edge), where id = 1.
• The technological level associated with the emer-
gence of new technologies, etc., where id =
1000 = 1K.
• Conceptual level containing new knowledge and
discoveries, where id = 1000000 = 1M = 1000K.
• The planetary level is determined by the depth
of penetration of human activity into the macro
and micro world, where id = 1000000000 = 1G =
1000M = 1000000K.
In fConvert
t
k
– informativeness as a measure of
usefulness. The level of structuring of knowledge of
Flexible Evolutionary Model of Machine Learning of Organizational Capital Development Strategies with Optimization of Spent Resources
73