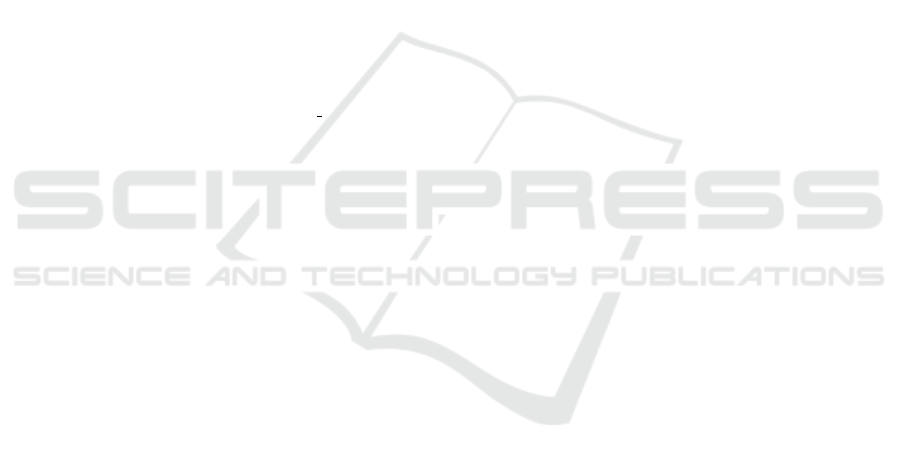
133. CEUR-WS.org. https://ceur-ws.org/Vol-3048/
paper03.pdf.
Bielinskyi, A. O. and Soloviev, V. N. (2018). Complex
network precursors of crashes and critical events in
the cryptocurrency market. CEUR Workshop Pro-
ceedings, 2292:37 – 45. https://ceur-ws.org/Vol-2292/
paper02.pdf.
Bondarenko, M. (2021). Modeling relation between at-
the-money local volatility and realized volatility of
stocks. Neiro-Nechitki Tekhnolohii Modelyuvannya
v Ekonomitsi, 2021(10):46–66. https://doi.org/10.
33111/nfmte.2021.046.
Charles, L., Webber, J., Cornel, I., and Norbert, M., edi-
tors (2015). Recurrence Plots and Their Quantifica-
tions: Expanding Horizons, vol.180 of Springer Pro-
ceedings in Physics. Springer. https://doi.org/10.1007/
978-3-319-29922-8.
Coleman, L. (2012). Explaining crude oil prices using
fundamental measures. Energy Policy, 40:318–324.
https://doi.org/10.1016/j.enpol.2011.10.012.
Dong, Y., Zhang, M., and Zhou, R. (2018). Classification
of network game traffic using machine learning. In
Yuan, H., Geng, J., Liu, C., Bian, F., and Surapunt,
T., editors, Geo-Spatial Knowledge and Intelligence,
pages 134–145, Singapore. Springer Singapore. https:
//doi.org/10.1007/978-981-13-0893-2 15.
D
´
ees, S., Karadeloglou, P., Kaufmann, R. K., and S
´
anchez,
M. (2007). Modelling the world oil market: Assess-
ment of a quarterly econometric model. Energy Pol-
icy, 35(1):178–191. https://doi.org/10.1016/j.enpol.
2005.10.017.
Eckmann, J.-P., Kamphorst, S. O., and Ruelle, D. (1987).
Recurrence plots of dynamical systems. Europhysics
Letters (EPL), 4(9):973–977. https://dx.doi.org/10.
1209/0295-5075/4/9/004.
Eckmann, J.-P. and Ruelle, D. (1985). Ergodic theory of
chaos and strange attractors. Rev. Mod. Phys., 57:617–
656. https://doi.org/10.1103/RevModPhys.57.617.
Fang, T., Zheng, C., and Wang, D. (2023). Forecasting the
crude oil prices with an emd-isbm-fnn model. En-
ergy, 263:125407. https://doi.org/10.1016/j.energy.
2022.125407.
Flood, R. P. and Hodrick, R. J. (1990). On testing for spec-
ulative bubbles. Journal of Economic Perspectives,
4(2):85–101. https://www.aeaweb.org/articles?id=10.
1257/jep.4.2.85.
Goldberger, A. L., Amaral, L. A. N., Glass, L., Haus-
dorff, J. M., Ivanov, P. C., Mark, R. G., Mietus,
J. E., Moody, G. B., Peng, C.-K., and Stanley, H. E.
(2000). Physiobank, physiotoolkit, and physionet:
components of a new research resource for complex
physiologic signals. Circulation, 101(23):e215–e220.
https://doi.org/10.1161/01.cir.101.23.e215.
Guliyev, H. and Mustafayev, E. (2022). Predicting the
changes in the wti crude oil price dynamics using ma-
chine learning models. Resources Policy, 77:102664.
https://doi.org/10.1016/j.resourpol.2022.102664.
He, Q. and Huang, J. (2020). A method for analyz-
ing correlation between multiscale and multivari-
ate systems—multiscale multidimensional cross re-
currence quantification (mmdcrqa). Chaos, Solitons
& Fractals, 139:110066. https://doi.org/10.1016/j.
chaos.2020.110066.
Ji, Q., Bouri, E., Roubaud, D., and Kristoufek, L. (2019).
Information interdependence among energy, cryp-
tocurrency and major commodity markets. Energy
Economics, 81:1042–1055. https://doi.org/10.1016/j.
eneco.2019.06.005.
Kantz, H. and Schreiber, T. (2003). Nonlinear Time Se-
ries Analysis. Cambridge University Press, 2 edition.
https://doi.org/10.1017/CBO9780511755798.
Kilian, L. (2009). Not all oil price shocks are alike: Dis-
entangling demand and supply shocks in the crude
oil market. American Economic Review, 99(3):1053–
69. https://www.aeaweb.org/articles?id=10.1257/aer.
99.3.1053.
Kirchner, M., Schubert, P., Liebherr, M., and Haas, C. T.
(2014). Detrended fluctuation analysis and adap-
tive fractal analysis of stride time data in parkinson’s
disease: Stitching together short gait trials. PLOS
ONE, 9(1):1–6. https://doi.org/10.1371/journal.pone.
0085787.
Kiv, A. E., Soloviev, V. N., Semerikov, S. O., Danylchuk,
H. B., Kibalnyk, L. O., Matviychuk, A. V., and
Striuk, A. M. (2021). Machine learning for pre-
diction of emergent economy dynamics III. In Kiv,
A. E., Soloviev, V. N., and Semerikov, S. O., edi-
tors, Proceedings of the Selected and Revised Papers
of 9th International Conference on Monitoring, Mod-
eling & Management of Emergent Economy (M3E2-
MLPEED 2021), Odessa, Ukraine, May 26-28, 2021,
volume 3048 of CEUR Workshop Proceedings, pages
i–xxxi. CEUR-WS.org. https://ceur-ws.org/Vol-3048/
paper00.pdf.
Kmytiuk, T. and Majore, G. (2021). Time series forecasting
of agricultural product prices using Elman and Jordan
recurrent neural networks. Neiro-Nechitki Tekhnolohii
Modelyuvannya v Ekonomitsi, 2021(10):67–85. https:
//doi.org/10.33111/nfmte.2021.067.
Kobets, V. and Novak, O. (2021). EU countries clustering
for the state of food security using machine learning
techniques. Neiro-Nechitki Tekhnolohii Modelyuvan-
nya v Ekonomitsi, 2021(10):86–118. https://doi.org/
10.33111/nfmte.2021.086.
Kucherova, H., Honcharenko, Y., Ocheretin, D., and Bil-
ska, O. (2021). Fuzzy logic model of usability of
websites of higher education institutions in the con-
text of digitalization of educational services. Neiro-
Nechitki Tekhnolohii Modelyuvannya v Ekonomitsi,
2021(10):119–135. https://doi.org/10.33111/nfmte.
2021.119.
Li, J., Wu, Q., Tian, Y., and Fan, L. (2021). Monthly henry
hub natural gas spot prices forecasting using varia-
tional mode decomposition and deep belief network.
Energy, 227:120478. https://doi.org/10.1016/j.energy.
2021.120478.
Lukianenko, D. and Strelchenko, I. (2021). Neuromodel-
ing of features of crisis contagion on financial mar-
kets between countries with different levels of eco-
nomic development. Neiro-Nechitki Tekhnolohii Mod-
M3E2 2022 - International Conference on Monitoring, Modeling Management of Emergent Economy
132