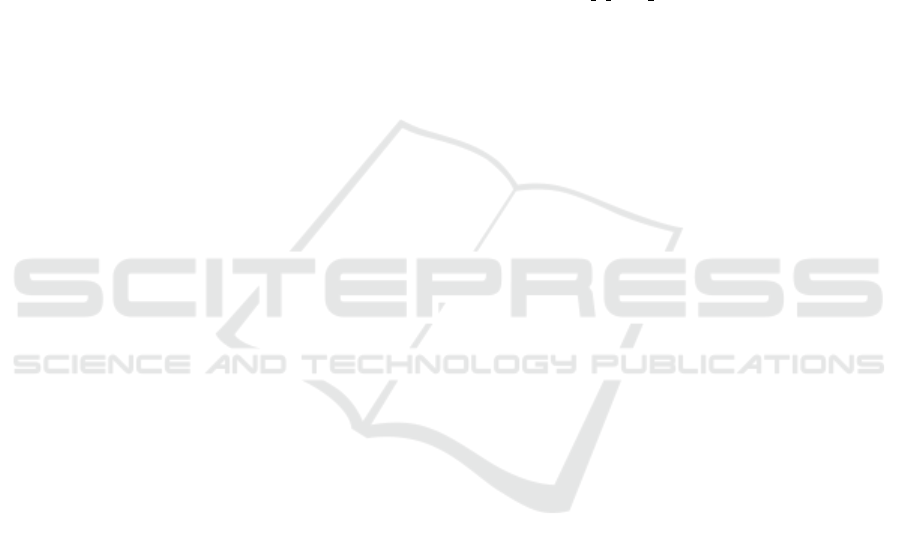
Danylchuk, H., Chebanova, N., Reznik, N., and Vitkovskyi,
Y. (2019). Modeling of investment attractiveness
of countries using entropy analysis of regional stock
markets. Global J. Environ. Sci. Manage, 5:227–235.
De Jongh, J. J. and Meyer, D. F. (2019). The mul-
tidimensional regional economic development index
(MREDI) applied in the north-west province: A
rural regional application. Administratio Publica,
27(3):162–185. https://cutt.ly/EVTaGVA.
Dorozynski, T. and Kuna-Marszałek, A. (2016). Invest-
ment attractiveness. The case of the Visegrad Group
countries. Comparative Economic Research. Central
and Eastern Europe, 19(1):117–138. https://doi.org/
10.1515/cer-2016-0007.
EBA (2020). Indeks investytsiinoi pryvablyvosti Ukrainy
2020 [Investment attractiveness index of Ukraine
2020]. https://cutt.ly/MU2j5w5.
Godlewska-Majkowska, H. (2018). Investment attrac-
tiveness of polish municipalities in relation to lo-
cal entrepreneurship. Olsztyn Economic Journal,
13(2):103–122. https://doi.org/10.31648/oej.2764.
Gorbatiuk, K., Mantalyuk, O., Proskurovych, O., and
Valkov, O. (2019). Analysis of regional develop-
ment disparities in ukraine using fuzzy clustering. In
Kiv, A., Semerikov, S., Soloviev, V. N., Kibalnyk,
L., Danylchuk, H., and Matviychuk, A., editors, Pro-
ceedings of the Selected Papers of the 8th Interna-
tional Conference on Monitoring, Modeling & Man-
agement of Emergent Economy, M3E2-EEMLPEED
2019, Odessa, Ukraine, May 22-24, 2019, volume
2422 of CEUR Workshop Proceedings, pages 194–
210. CEUR-WS.org. http://ceur-ws.org/Vol-2422/
paper16.pdf.
Heritage Foundation (2020). Index of Economic Freedom
2020. https://www.heritage.org/index/ranking.
Hryhoruk, P., Grygoruk, S., Khrushch, N., and Hov-
orushchenko, T. (2020a). Using non-metric multidi-
mensional scaling for assessment of regions’ econ-
omy in the context of their sustainable development.
In Kiv, A., editor, Proceedings of the Selected Papers
of the Special Edition of International Conference on
Monitoring, Modeling & Management of Emergent
Economy (M3E2-MLPEED 2020), Odessa, Ukraine,
July 13-18, 2020, volume 2713 of CEUR Workshop
Proceedings, pages 315–333. CEUR-WS.org. http:
//ceur-ws.org/Vol-2713/paper35.pdf.
Hryhoruk, P., Khrushch, N., and Grygoruk, S. (2019a). An
approach to design a composite index of economic
development and identifying the bounds of its levels.
In Proceedings of the 2019 9th International Confer-
ence on Advanced computer information technologies
ASIT’2019, pages 48–51, Ceske Budejovice Czech
Republic.
Hryhoruk, P., Khrushch, N., and Grygoruk, S. (2019b). The
rating model of Ukraine’s regions according to the
level of economic development. Periodicals of En-
gineering and Natural Sciences, 7(2):712–722. https:
//cutt.ly/BjAgnAL.
Hryhoruk, P., Khrushch, N., and Grygoruk, S. (2020b).
Using multidimensional scaling for assessment eco-
nomic development of regions. Journal of Industrial
Engineering & Production Research, 31(4):597–607.
https://cutt.ly/hVTsxRJ.
Hryhoruk, P., Khrushch, N., and Grygoruk, S. (2021).
Environmental safety assessment: a regional dimen-
sion. IOP Conference Series: Earth and Environ-
mental Science, 628:012026. https://doi.org/10.1088/
1755-1315/628/1/012026.
IMDWCC (2020). IMD World Competitiveness Cen-
ter. World Competitiveness Ranking. https://cutt.ly/
RU2kryT.
Jac, I. and Vondrackova, M. (2018). The perception
of selected aspects of investment attractiveness by
businesses making investments in the Czech Repub-
lic. E+M Ekonomie a Management, 20(3):103–
122. https://dspace.tul.cz/bitstream/handle/15240/
20918/EM
3 2017 08.pdf.
Kaminskyi, A., Miroshnychenko, I., and Pysanets, K.
(2019). Risk and return for cryptocurrencies as alter-
native investment: Kohonen maps clustering. Neiro-
Nechitki Tekhnolohii Modelyuvannya v Ekonomitsi,
2019(8):175–193.
Kearney (2020). Kearney Foreign Direct Investment
(FDI) Confidence Index. https://www.kearney.com/
foreign-direct-investment-confidence-index.
Kikkas, X. N. and Krasnozhenova, E. (2018). Modeling
investment attractiveness of Germany. In Proceed-
ings of the 5th International Multidisciplinary Scien-
tific Conference on Social Sciences and Arts SGEM
2018, pages 515–522, Sofia Bulgaria.
KOF (2020). KOF Globalization Index. https://cutt.ly/
DU2kuCW.
Korenyuk, P. and Kopil, E. (2018). Conceptual approaches
and methods for determining the investment attrac-
tiveness of the national economy. Economic Jour-
nal of Lesya Ukrainka Eastern European National
University, 2(14):56–62. https://doi.org/10.29038/
2411-4014-2018-02-56-62.
Kyshakevcyh, B. and Nakhaeva, M. (2021). Intehralna
otsinka investytsiinoi pryvablyvosti rehioniv Ukrainy
[Integrated assessment of investment attractiveness of
regions in Ukraine]. Herald of Khmelnytskyi Na-
tional University Economic sciences, (4):51–58. https:
//cutt.ly/MVTpdJ3.
Kyshakevych, B., Nahayeva, M., and Kry
´
nski, A. (2020).
The economic essence of the investment attractiveness
of the regions. Scientific Journal of Polonia Univer-
sity, 37(6):46–53. https://doi.org/10.23856/3704.
Lagler, K. (2020). Use of world experience in increasing
investment attractiveness of Ukraine regions. Eco-
nomics, Finance And Management Review, 2:63–70.
Leshchuk, H. (2020). Systematyzatsiia pidkhodiv do otsini-
uvannia investytsiinoi pryvablyvosti rehionu [System-
atization of the approaches to estimating the invest-
ment attractiveness of a region]. Uzhorod National
University Herald. International Economic Relations
and World Economy, (29):93–98. https://doi.org/10.
32782/2413-9971/2020-29-18.
Mazziotta, M. and Pareto, A. A. (2014). Composite index
for measuring Italian regions’ development over time.
M3E2 2022 - International Conference on Monitoring, Modeling Management of Emergent Economy
154