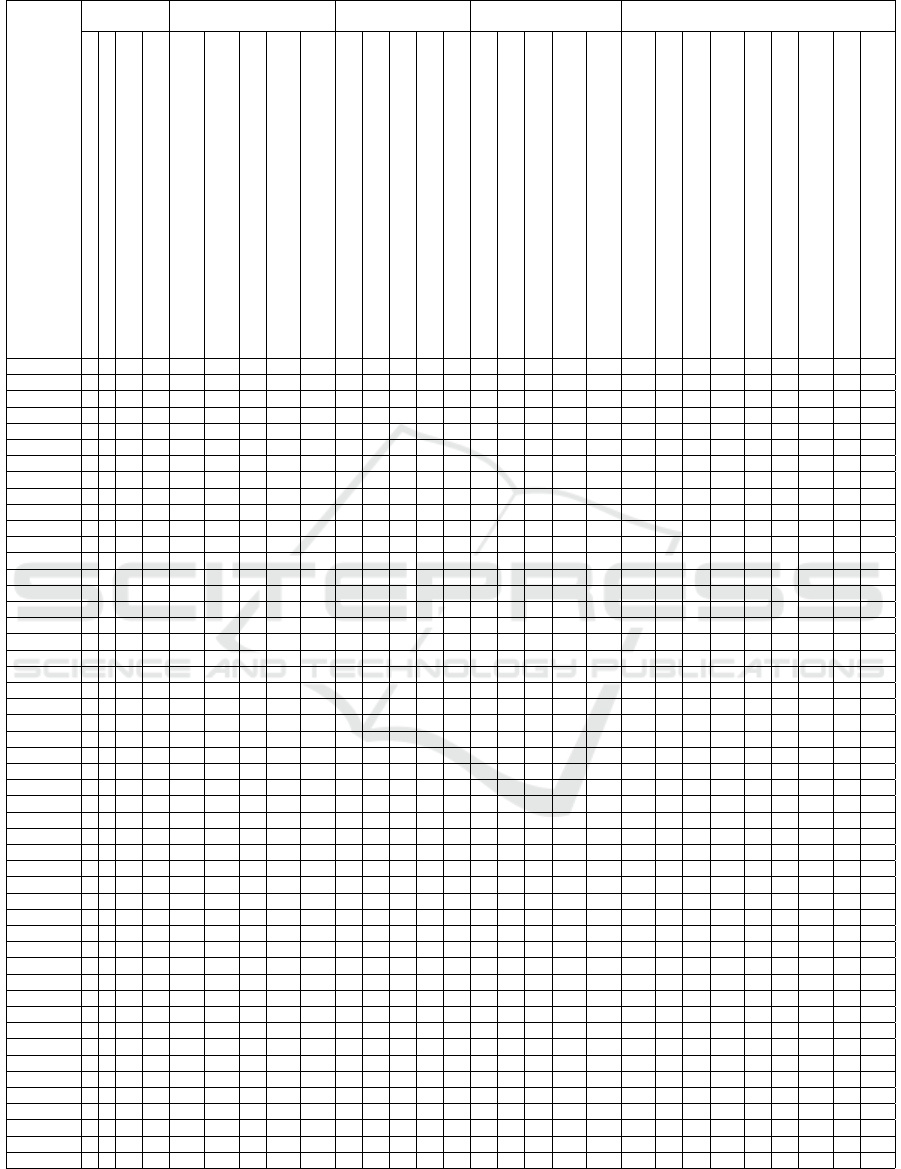
Table 1: Indicators of evaluation of international competitiveness of countries’ universities.
OVERALL
RANKING
RESOURCES
2020 SCORES
ENVIRONMENT
2020 SCORES
CONNECTIVITY
2020 SCORES
OUTPUT
2020 SCORES
Country
Rank 2020
Rank 2019
Score 2020
Score 2019
Government expenditure on tertiary
education as a percentage of GDP
Total expenditure on tertiary
education as a percentage of GDP
Total expenditure per student USD PPP
Expenditure in tertiary institutions
for R&D as a percent of GDP
Expenditure in tertiary institutions
for R&D per head of population
Proportion of female students
Proportion of female academic staff
Data quality
Qualitative index of environment
WEF Survey
Proportion of international students
Proportion of articles with international collaborators
Webometrics VISIBILITY index divided by population
Rating of knowledge transfer
between university and companies
Percentage of university research
publications co-authored with industry
Total number of documents produced
by higher education institutions
Total documents produced per head of population
Average impact of articles
Weighted Shanghai ranking scores for
universities per head of population
Shanghai scores for best three universities
Tertiary enrollment rates
Percentage of population aged 24-64
with a tertiary qualification
Number of researchers in the nation per head of population
Unemployment rate of the tertiary
educated compared with school leavers
Argentina 40 38 46 45,1 56,7 48,4 13,8 13,4 4,7 100 97,1 100 67,8 51,3 9,1 52,4 7 54,1 19,5 2,1 6,2 45,9 2,6 13,1 90 61,6 14,9 30,9
Australia 9 8 82,2 80,9 37,7 70,6 42,9 64 51,2 100 91,3 100 98,1 81,9 78,9 72,8 56 68,1 41,4 15,9 85,3 84,3 76,8 39,3 100 79 55 32,6
Austria 12 12 79,3 77,2 81,9 64,8 48,7 68,6 63,7 100 84,7 100 72 68,3 63,1 86,9 54 84,8 100 3,3 49,4 86 57 22 85,1 56,5 62,5 31,3
Belgium 13 13 75,6 73,6 63,8 55,4 48,3 53 45 100 97,1 100 75,8 82,2 31,8 89,3 28,4 82,5 78,8 4,8 56,6 94,2 51,4 31,4 79,7 70,2 59,9 39,1
Brazil 41 40 45,6 44,1 49,9 66,5 37,9 n.a. n.a. 100 91,4 88,6 63,8 41,8 0,9 43,8 6,9 40,3 26,2 11,6 7,5 45,3 3,8 21,2 51,3 31,8 10,7 39,7
Bulgaria 45 44 42,7 41,8 32,3 40,3 17,8 4,3 1,6 100 97,9 93,2 53,1 54,7 16,8 57,5 10,9 46 44,3 0,8 14,6 55,2 3,3 2,7 71,2 38,1 25,8 45,2
Canada 7 6 83,2 81,9 62,5 86,8 62,9 63,6 53 100 88,6 90,9 73,3 87,1 47,4 68,8 69,2 86,3 59 17,2 62,6 82 44 42,9 88,2 100 51,8 33,7
Chile 31 32 54,3 51,3 48,4 100 22,3 14,8 6 100 85,1 100 81,4 54,8 1,4 81,6 14,3 62,3 28,3 2,2 16,1 63,4 8,2 11,8 88,5 43,5 6,1 30,2
China 26 27 56,8 54,7 42,5 50,7 20 15 4,4 100 n.a. 88,6 76,6 73 1,3 34,1 8,4 65,9 32 70,7 6,8 59,3 7,5 39,6 49,1 16,7 15 n.a.
Croatia 43 43 43,6 42,1 49,9 36,8 18 24,9 11,2 100 97,8 93,2 47,3 47 1,6 58,3 11 35,5 50,8 0,9 30,4 52,9 17,2 8 66,5 39,2 22,6 31,2
Czech Rep. 29 26 54,8 55,2 36,3 35,1 26,6 34,4 22,2 100 76,9 100 69,3 61,1 46,1 62 29,3 53,6 55,7 2,9 37 62,8 22 14,7 64,1 41,9 44,7 40,4
Denmark 3 5 85,7 82,5 80,4 62,7 44,9 100 91,4 100 88,6 95,5 67,4 80,6 39,5 85,3 47,5 89,2 85,2 4,3 100 97,1 83,3 38,8 80,6 65,7 95,7 21
Finland 8 9 82,8 80,4 80,8 61,9 46,6 68,5 54,6 100 100 100 81,6 93,8 30 82,3 64,7 90 77 2,9 70,8 86 72,2 23,9 88,2 78,1 81,3 41,3
France 17 17 68,6 67,6 57 53,6 43 44,3 35 100 87,9 100 73,1 69,6 37,4 77,7 23,8 70,3 68,8 13,5 28,2 75,6 28,9 40,6 65,6 63,7 53,8 39,8
Germany 16 16 70,5 69,6 51,3 44,9 46,3 51,2 46 97 78,6 100 61,6 86,8 30,8 67,8 38,6 87,9 76 21 34,2 79,1 32,9 39,7 70,2 50,2 61 37
Greece 37 37 47,4 47 35,1 26 10,9 31,8 15,5 97,1 68,6 93,2 26,9 49,2 12,5 68,7 35,2 43,7 61,5 2,5 31,2 73,3 21 14,1 100 54,8 38,2 36
Hong Kong 14 15 72,7 70,2 50,1 55,6 64,7 39,8 43,3 100 n.a. 90,9 97,2 76,7 42 54,3 48,2 82,5 35,8 3,5 63,7 95,9 54,9 26,3 74,3 50,9 41,4 41,4
Hungary 33 35 51,3 48,5 34,7 39,7 30 17,6 8,8 100 80,5 100 51,6 47 36,6 70,9 22,1 58,6 82,8 1,6 22,3 69,2 14,4 10,7 48,5 43,4 35,4 54,6
India 49 49 39,6 38,8 54,8 59,1 13 2,4 0,3 96,2 81,2 90,9 58,1 74,6 0,5 27,2 0,9 57,8 19 14,9 1,5 47,1 0,6 12,5 27,4 18,3 2,6 12,6
Indonesia 50 50 35 33,5 25,7 25 7,9 4,6 1 100 86,2 100 64,7 71,6 0,3 23,6 4,4 72,5 31,4 3,1 1,6 45,3 0 0 36,4 20,5 2,6 26,4
Iran 47 48 42,2 39,2 50,2 51,9 15 n.a. n.a. 92,1 62,2 81,8 67 52,8 1,6 33,7 5,1 52,1 10,6 7,3 12 51,7 5 15,2 69,6 36,9 8,1 n.a.
Ireland 19 19 66 64,7 28,7 29,6 35,2 25,2 33,7 100 90 100 68,6 87,6 32,6 75,1 60,1 88,2 63 2,4 64,8 80,8 47,6 18,7 77,8 81,1 49,8 36,8
Israel 18 18 67,4 67,3 39,4 52,1 29,6 50,9 34,6 100 n.a. 95,5 73,3 74,9 10,6 66,3 34,6 91,1 49,5 3,2 48,5 77,9 51,5 30,6 63,4 88 100 34,6
Italy 30 30 54,5 53,4 28,6 33 30,8 32,1 22,5 100 74,2 100 63,8 60 19,5 62,9 18 60,5 54,2 15,7 34,9 77,3 29,4 24,6 61,9 33,4 27,8 35,6
Japan 20 20 61,9 61,7 21,2 51,2 51 37,6 28,9 95,4 56,8 100 83,2 70,8 15,7 39 18,9 57 78 17,2 18,4 50,6 14,5 42,9 63,6 89,7 64,3 34,5
Korea 24 23 58 57,4 32,7 64,4 27,8 37,7 25,7 83,4 70,2 100 58 56,3 8,3 37,9 14,8 62,6 61,4 12,4 32,3 56,4 24,1 24,8 94,3 84,7 91,1 25,2
Malaysia 27 28 56,1 54,5 56,5 75,1 39,4 48 22,9 100 100 95,5 78,6 83,7 29,6 59,5 7,5 79,4 16,3 3,6 15,1 55,8 5,8 14 43,7 37,7 28,6 21,6
Mexico 48 47 41,7 41,1 47,2 50,5 19,5 12,7 4,1 100 n.a. 95,5 82,4 48,5 2,1 53 3,8 52,9 19,5 3 3,2 42,4 0,8 11,1 40,2 31,1 3 20,7
Netherlands 10 10 81,6 80,2 59,8 62,7 51,8 58,3 54,3 100 91,7 100 79,3 88 40,4 82,5 47,5 96,7 85,4 9 70,7 97,7 59,4 37,5 85 66,2 60,7 34,7
New Zealand 14 14 72,7 71,5 44,1 64,5 39,7 33,6 21,4 100 99,7 100 89,7 86,5 72 77,3 55,8 76,1 46,4 2,2 59,5 79,1 64,6 18,4 82 67,9 49,1 33,9
Norway 11 11 80,5 77,8 89,9 70,7 58,4 68,8 74,8 100 92,6 100 66,9 85,9 11,6 80,8 58,9 81 61,8 3,1 78,2 87,2 63 28,1 82 75,3 78,5 32,2
Poland 32 31 52,6 52,2 48,4 43,9 23,8 33,3 17,2 100 90 100 81,9 58,3 15,1 42,3 17,3 60,4 32,3 6,4 22,8 58,1 7,3 14,1 67,8 53,4 30,6 49,1
Portugal 25 25 57,6 56,8 39,4 42,6 29,3 55,3 31 100 88,6 100 60,9 71,7 23,5 71,9 33,9 64,5 41,5 3,6 47,2 66,9 26,7 18,7 63,9 43,1 52 33,7
Romania 44 45 43 41,7 32,9 42,8 28,9 5,2 2,4 100 100 95,5 76 45,2 17,7 36,6 10,2 54 32,1 2,3 15,9 50,6 2,7 5,9 48,2 29,6 10,8 45,8
Russia 35 35 49,1 48,5 37,3 42,5 22,5 9,8 4,6 100 100 100 70,2 60,1 15 36,2 8,4 43,9 20,1 8,8 8,3 47,7 2,9 21,7 81,9 97,9 34,6 47,7
Saudi Arabia 22 22 59,3 59,3 100 77,7 53,1 n.a. n.a. 96,3 81,7 79,5 50,5 69,3 17,1 100 3,9 68,9 29,6 3,1 12,7 76,7 7,8 24,8 69,7 41,2 n.a. 9,4
Serbia 42 41 44,2 43,4 55,8 48,7 17,4 32,9 8,8 100 93,1 90,9 42,3 52,9 16,3 62,1 8,5 52,1 23,7 1,1 20,5 53,5 9,3 7,4 66,5 37,2 25,2 28,7
Singapore 4 7 84,5 81,3 50,1 53,7 100 63,4 100 100 74,1 95,5 82 94 100 87,7 36,7 91,7 38,6 2,8 66,5 94,8 41,4 26,5 84,8 86,5 81,6 30,6
Slovakia 38 33 47,2 49,6 35,8 37 30,3 21,2 11,9 100 91,5 100 64,2 44,8 25,3 57,7 16,8 35,6 64,4 1 24,2 63,4 4,7 2,9 46,6 42,5 33,9 46,4
Slovenia 28 29 55,4 53,6 44,5 38,3 29,9 20,3 12,6 100 85,1 100 63,7 65,3 14,3 71,1 25,1 63,1 53,6 0,7 48,8 67,4 31,4 7,4 78,6 56,1 54,2 35,9
SAR 34 34 49,7 48,7 37,4 49,9 28,9 26,2 6,1 100 n.a. 88,6 86,7 45,3 11,9 68,6 3,7 54,8 36,9 3,7 8,6 69,8 5,8 18,6 22,4 12,4 6 100
Spain 23 24 58,6 57,3 41,6 45,9 33,5 32 21,6 100 86,9 100 69,9 59,5 11,9 61,6 30,7 57 46,2 12,7 37 65,7 29,9 22 88,9 64,4 34,8 39,7
Sweden 5 4 84,3 82,9 71,2 59,7 64,6 83,2 73,7 100 89,7 100 75,2 76,9 24,8 86,8 59,6 83,1 86,2 6,3 83,5 89,5 82,5 38,8 67 74,7 92 24,4
Switzerland 2 2 90,1 88,6 64,9 51,7 75,4 88 97,6 99,4 71 100 69,5 100 65,2 91,3 79,7 100 76,9 5,8 91,7 100 100 44,2 59,6 75,6 63,7 30,4
Taiwan 21 21 60,5 60,5 33,5 51,8 32,8 29,3 26,2 100 72 93,2 86,9 72,3 16,2 45,4 44,3 80 38,3 5,1 29,1 55,2 20,3 19,7 84,5 84,5 76,1 25
Thailand 46 46 42,3 41,2 32,1 34,8 13,7 13,7 4,2 100 100 95,5 71,9 60,1 4,8 57,8 10,2 65,5 34,7 2,1 4,3 53,5 1,8 11,3 49,3 28,1 14,7 18,2
Turkey 39 42 46,3 43,3 71,1 70,5 27,9 31,5 14,8 92 88 100 44,9 51,3 5,5 30,6 7,6 57,4 16,6 7,2 11,9 44,2 4,1 11,2 94,7 35,9 16,8 23,2
Ukraine 36 38 47,8 45,1 76,4 63,9 10,8 3,2 0,5 100 n.a. 90,9 60,6 62,4 11,6 41,2 8 45,8 60,4 1,3 4,2 33,1 0 0 83,4 84,4 12 58,3
UK 6 3 83,6 84,5 28,2 64,7 63,1 38,8 29,7 100 89,5 100 89,5 75,5 65,8 72,1 63,7 82,1 68,9 31,1 63,1 86 58,1 73,7 60 79,1 53,1 34,4
USA 1 1 100 100 42,6 91,2 80,1 35,7 37,7 100 98,2 100 100 90,8 19 45 100 92,3 58,4 100 41,2 78,5 43,4 100 88,2 81,9 51,6 48,7
M3E2 2022 - International Conference on Monitoring, Modeling Management of Emergent Economy
208