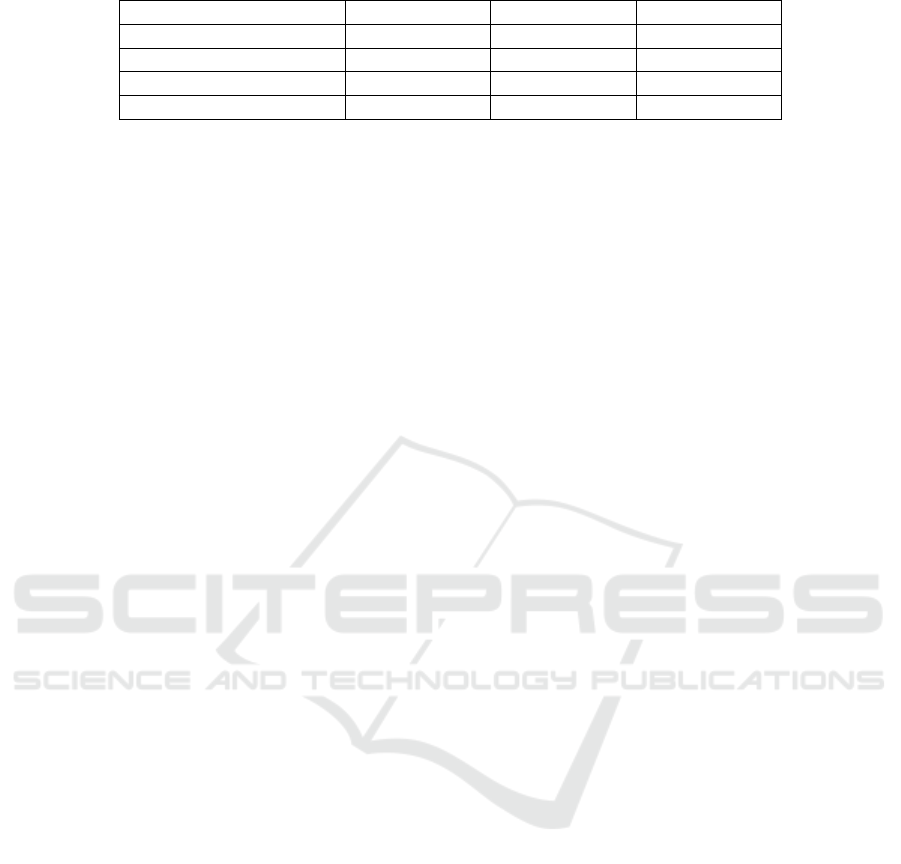
Table 1: Average and standard deviation over S test sets.
Model Sensitivity Specificity F1
LLE+LDA 0.555(±0.425) 0.453(±0.321) 0.440(±0.298)
Previous proposed model 0.643(±0.227) 0.894(±0.106) 0.609(±0.084)
Proposed model⋆ 0.653(±0.124) 0.889(±0.178) 0.742(±0.098)
Proposed model 0.871(±0.134) 0.838(±0.102) 0.859(±0.113)
5 CONCLUSIONS AND FUTURE
WORKS
In this work, we propose improving the methods to
arrive at an overload forecasting model in a complex,
multivariate, and highly unbalanced problem using a
Gram matrix-based encoding.
We take advantage of the benefits of the CNNs to
generate a model that allows us to know the relation-
ships of these matrices with the overload.
The experimental results show that we have over-
come the approach in previous works and state of the
art.
Using an explanation method called Grad-
CAM++, we established some interesting study sets
for expert review, for example, the relationship be-
tween pressure and timing of equipment maintenance
and fine grain size and pressure to explain some over-
loads.
Also, this behavior could allow us to increase the
forecast distance. In the future, we will integrate the
care information in the same network to generate a
model specialized mainly in those elements that most
influence the occurrence of overloads.
REFERENCES
Apelt, T., Asprey, S., and Thornhill, N. (2001). Inferential
measurement of sag mill parameters. Minerals engi-
neering, 14(6):575–591.
Bardinas, J., Aldrich, C., and Napier, L. (2018). Predict-
ing the operating states of grinding circuits by use of
recurrence texture analysis of time series data. Pro-
cesses, 6(2):17.
Bergmeir, C. and Ben
´
ıtez, J. M. (2012). On the use of cross-
validation for time series predictor evaluation. Infor-
mation Sciences, 191:192–213.
C
´
esar, G. Q. and Daniel, S. H. (2009). Multivariable model
predictive control of a simulated sag plant. IFAC Pro-
ceedings Volumes, 42(23):37–42.
Chattopadhay, A., Sarkar, A., Howlader, P., and Balasub-
ramanian, V. N. (2018). Grad-cam++: Generalized
gradient-based visual explanations for deep convolu-
tional networks. In 2018 IEEE winter conference on
applications of computer vision (WACV), pages 839–
847. IEEE.
Forbes, M. G. and Gough, B. (2003). Model predictive con-
trol of sag mills and flotation circuits.
Hermosilla, R., Valle, C., Allende, H., Lucic, E., and Es-
pinoza, P. (2021). Semi-autogeonous (sag) mill over-
load forecasting. In Iberoamerican Congress on Pat-
tern Recognition, pages 392–401. Springer.
Ko, Y.-D. and Shang, H. (2011). Sag mill system di-
agnosis using multivariate process variable analy-
sis. The Canadian Journal of Chemical Engineering,
89(6):1492–1501.
Liang, J., Hou, L., Luan, Z., and Huang, W. (2019). Feature
selection with conditional mutual information consid-
ering feature interaction. Symmetry, 11(7):858.
McClure, K. and Gopaluni, R. (2015). Overload de-
tection in semi-autogenous grinding: A nonlinear
process monitoring approach. IFAC-PapersOnLine,
48(8):960–965.
Northey, S., Haque, N., and Mudd, G. (2013). Using sus-
tainability reporting to assess the environmental foot-
print of copper mining. Journal of Cleaner Pro-
duction, 40:118–128. Special Volume: Sustainable
consumption and production for Asia: Sustainability
through green design and practice.
Pontt, J., Valderrama, W., Olivares, M., Rojas, F., Robles,
H., L’Huissiers, S., and Leiva, F. (2012). Uso eficiente
de la energia en procesos mineros. Centro de automa-
tizacion para la industria minera, Chile.
Powell, M., Van der Westhuizen, A., and Mainza, A. (2009).
Applying grindcurves to mill operation and optimisa-
tion. Minerals Engineering, 22(7-8):625–632.
Salazar, J.-L., Vald
´
es-Gonz
´
alez, H., Vyhmesiter, E., and
Cubillos, F. (2014). Model predictive control of semi-
autogenous mills (sag). Minerals Engineering, 64:92–
96.
Strohmayr, S. and Valery, W. (2001). Sag mill circuit op-
timisation at ernest henry mining. In Proceedings of
the SAG 2001 Conference, Vancouver, BC, Canada,
volume 30.
Varas, P., Carvajal, R., and Ag
¨
uero, J. C. (2019). State
estimation for sag mills utilizing a simplified model
with an alternative measurement. In 2019 IEEE
CHILEAN Conference on Electrical, Electronics En-
gineering, Information and Communication Technolo-
gies (CHILECON), pages 1–7. IEEE.
Wakefield, B., Lindner, B., McCoy, J., and Auret, L. (2018).
Monitoring of a simulated milling circuit: Fault diag-
nosis and economic impact. Minerals Engineering,
120:132–151.
Wang, J., Li, S., Ji, W., Jiang, T., and Song, B. (2022). A
t-cnn time series classification method based on gram
matrix. Scientific Reports, 12(1):1–14.
Wang, X., Yi, J., Zhou, Z., and Yang, C. (2020). Optimal
speed control for a semi-autogenous mill based on dis-
crete element method. Processes, 8(2):233.
ISAIC 2022 - International Symposium on Automation, Information and Computing
396