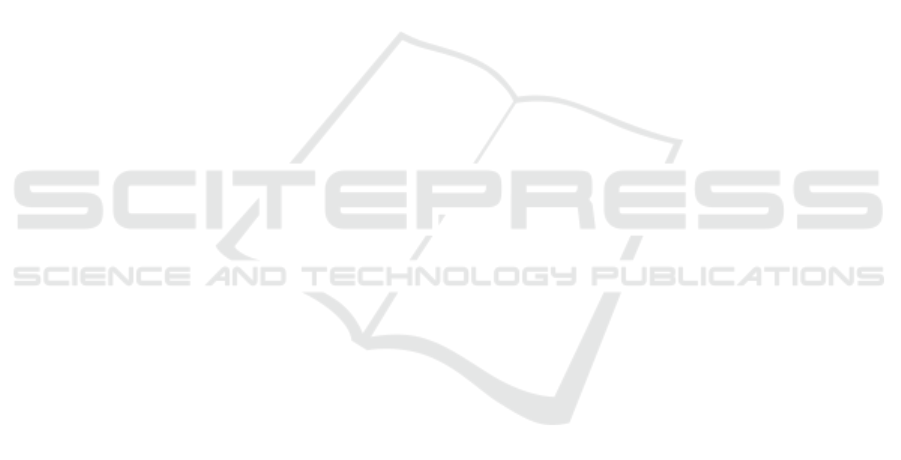
REFERENCES
Abarbanell, J. S. and Bushee, B. J. (1998). Abnormal re-
turns to a fundamental analysis strategy. Accounting
Review, pages 19–45.
Carapuc¸o, J., Neves, R., and Horta, N. (2018). Reinforce-
ment learning applied to forex trading. Applied Soft
Computing, 73:783–794.
Chan, E. (2013). Algorithmic trading: winning strategies
and their rationale, volume 625. John Wiley & Sons.
Chen, L. and Gao, Q. (2019). Application of deep reinforce-
ment learning on automated stock trading. In 2019
IEEE 10th International Conference on Software En-
gineering and Service Science (ICSESS), pages 29–
33. IEEE.
Cultrera, L., Seidenari, L., Becattini, F., Pala, P., and
Del Bimbo, A. (2020). Explaining autonomous driv-
ing by learning end-to-end visual attention. In Pro-
ceedings of the IEEE/CVF Conference on Computer
Vision and Pattern Recognition Workshops, pages
340–341.
Deng, Y., Bao, F., Kong, Y., Ren, Z., and Dai, Q. (2016).
Deep direct reinforcement learning for financial signal
representation and trading. IEEE transactions on neu-
ral networks and learning systems, 28(3):653–664.
Huang, C. Y. (2018). Financial trading as a game: A
deep reinforcement learning approach. arXiv preprint
arXiv:1807.02787.
Lei, K., Zhang, B., Li, Y., Yang, M., and Shen, Y. (2020).
Time-driven feature-aware jointly deep reinforcement
learning for financial signal representation and algo-
rithmic trading. Expert Systems with Applications,
140:112872.
Lim, B., Zohren, S., and Roberts, S. (2019). Enhancing
time-series momentum strategies using deep neural
networks. The Journal of Financial Data Science,
1(4):19–38.
Littman, M. L. (1994). Markov games as a framework
for multi-agent reinforcement learning. In Machine
learning proceedings 1994, pages 157–163. Elsevier.
Mao, H., Alizadeh, M., Menache, I., and Kandula, S.
(2016). Resource management with deep reinforce-
ment learning. In Proceedings of the 15th ACM work-
shop on hot topics in networks, pages 50–56.
Mihatsch, O. and Neuneier, R. (2002). Risk-sensitive re-
inforcement learning. Machine learning, 49(2):267–
290.
Moody, J., Wu, L., Liao, Y., and Saffell, M. (1998). Perfor-
mance functions and reinforcement learning for trad-
ing systems and portfolios. Journal of Forecasting,
17(5-6):441–470.
Murphy, J. J. (1999). Technical analysis of the financial
markets: A comprehensive guide to trading methods
and applications. Penguin.
Schwager, J. D. (2017). A Complete Guide to the Futures
Market: Technical Analysis, Trading Systems, Funda-
mental Analysis, Options, Spreads, and Trading Prin-
ciples. John Wiley & Sons.
Shalev-Shwartz, S., Shammah, S., and Shashua, A. (2016).
Safe, multi-agent, reinforcement learning for au-
tonomous driving. arXiv preprint arXiv:1610.03295.
Singhvi, S. (1988). How to make money in stocks: A win-
ning system in good times or bad. Management Re-
view, 77(9):61–63.
Sutton, R. S. and Barto, A. G. (2018). Reinforcement learn-
ing: An introduction. MIT press.
Vinyals, O., Babuschkin, I., Czarnecki, W. M., Mathieu,
M., Dudzik, A., Chung, J., Choi, D. H., Powell, R.,
Ewalds, T., Georgiev, P., et al. (2019). Grandmas-
ter level in starcraft ii using multi-agent reinforcement
learning. Nature, 575(7782):350–354.
Zhang, Z., Zohren, S., and Roberts, S. (2020). Deep rein-
forcement learning for trading. The Journal of Finan-
cial Data Science, 2(2):25–40.
Zoph, B., Vasudevan, V., Shlens, J., and Le, Q. V. (2018).
Learning transferable architectures for scalable image
recognition. In Proceedings of the IEEE conference on
computer vision and pattern recognition, pages 8697–
8710.
ISAIC 2022 - International Symposium on Automation, Information and Computing
494