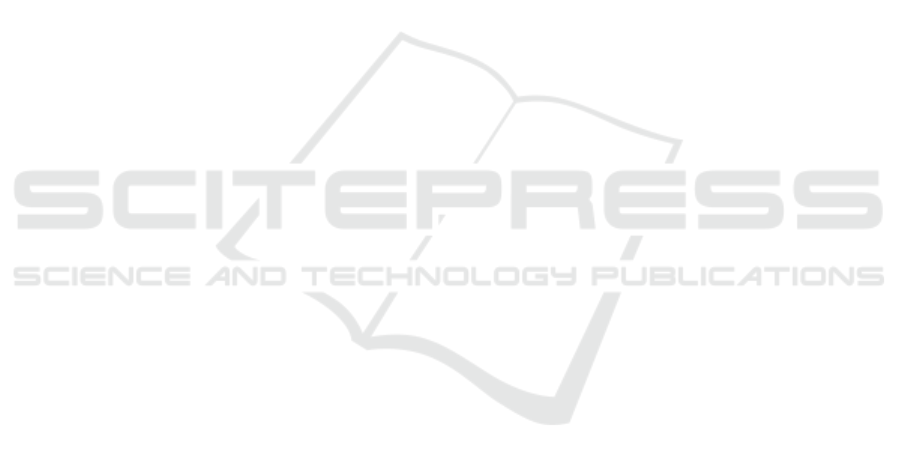
Fong, T., Thorpe, C., and Baur, C. (2001). Collaborative
control: A robot-centric model for vehicle teleoper-
ation, volume 1. Carnegie Mellon University, The
Robotics Institute Pittsburgh.
Gateau, T., Chanel, C. P. C., Le, M.-H., and Dehais, F.
(2016). Considering human’s non-deterministic be-
havior and his availability state when designing a col-
laborative human-robots system. In 2016 IEEE/RSJ
International Conference on Intelligent Robots and
Systems (IROS), pages 4391–4397.
Haksar, R. N. and Schwager, M. (2018). Distributed Deep
Reinforcement Learning for Fighting Forest Fires
with a Network of Aerial Robots. IEEE International
Conference on Intelligent Robots and Systems, pages
1067–1074.
Hung, S. M. and Givigi, S. N. (2017). A Q-Learning
Approach to Flocking with UAVs in a Stochastic
Environment. IEEE Transactions on Cybernetics,
47(1):186–197.
Isufaj, R., Omeri, M., and Piera, M. A. (2021). Multi-uav
conflict resolution with graph convolutional reinforce-
ment learning. CoRR, abs/2111.14598.
Jafari, M., Xu, H., and Carrillo, L. R. G. (2020). A
biologically-inspired reinforcement learning based in-
telligent distributed flocking control for Multi-Agent
Systems in presence of uncertain system and dynamic
environment. IFAC Journal of Systems and Control,
13:100096.
Liu, C. H., Chen, Z., and Zhan, Y. (2019). Energy-Efficient
Distributed Mobile Crowd Sensing: A Deep Learning
Approach. IEEE Journal on Selected Areas in Com-
munications, 37(6):1262–1276.
Liu, C. H., Ma, X., Gao, X., and Tang, J. (2020a). Dis-
tributed Energy-Efficient Multi-UAV Navigation for
Long-Term Communication Coverage by Deep Rein-
forcement Learning. IEEE Transactions on Mobile
Computing, 19(6):1274–1285.
Liu, Y., Liu, H., Tian, Y., and Sun, C. (2020b). Reinforce-
ment learning based two-level control framework of
UAV swarm for cooperative persistent surveillance in
an unknown urban area. Aerospace Science and Tech-
nology, 98:105671.
Lowe, R., Wu, Y., Tamar, A., Harb, J., Abbeel, P., and
Mordatch, I. (2017). Multi-agent actor-critic for
mixed cooperative-competitive environments. CoRR,
abs/1706.02275.
Mnih, V., Kavukcuoglu, K., Silver, D., Graves, A.,
Antonoglou, I., Wierstra, D., and Riedmiller, M.
(2013). Playing atari with deep reinforcement learn-
ing.
Suzanne Barber, K., Goel, A., and Martin, C. E. (2000).
Dynamic adaptive autonomy in multi-agent systems.
Journal of Experimental & Theoretical Artificial In-
telligence, 12(2):129–147.
Tan, M. (1993). Multi-Agent Reinforcement Learning:
Independent vs. Cooperative Agents. Mach. Learn.
Proc. 1993, pages 330–337.
Tang, J., Song, J., Ou, J., Luo, J., Zhang, X., and Wong,
K. K. (2020). Minimum Throughput Maximization
for Multi-UAV Enabled WPCN: A Deep Reinforce-
ment Learning Method. IEEE Access, 8:9124–9132.
Undertaking, S. E. S. A. R. . J. (2017). European drones
outlook study : unlocking the value for Europe. Pub-
lications Office.
WANG, C., WU, L., YAN, C., WANG, Z., LONG, H., and
YU, C. (2020). Coactive design of explainable agent-
based task planning and deep reinforcement learning
for human-UAVs teamwork. Chinese Journal of Aero-
nautics, 33(11):2930–2945.
Wang, L., Wang, K., Pan, C., Xu, W., Aslam, N., and
Hanzo, L. (2021). Multi-Agent Deep Reinforcement
Learning-Based Trajectory Planning for Multi-UAV
Assisted Mobile Edge Computing. IEEE Transac-
tions on Cognitive Communications and Networking,
7(1):73–84.
Wang, W., Lu, X., Liu, S., Xiao, L., and Yang, B.
(2020). Energy Efficient Relay in UAV Networks
Against Jamming: A Reinforcement Learning Based
Approach. IEEE Vehicular Technology Conference,
2020-May:0–4.
Wu, X., Wang, C., Niu, Y., Hu, X., and Fan, C. (2018).
Adaptive human-in-the-loop multi-target recognition
improved by learning. International Journal of Ad-
vanced Robotic Systems, 15(3):1729881418774222.
ICCAS 2022 - International Conference on Cognitive Aircraft Systems
42