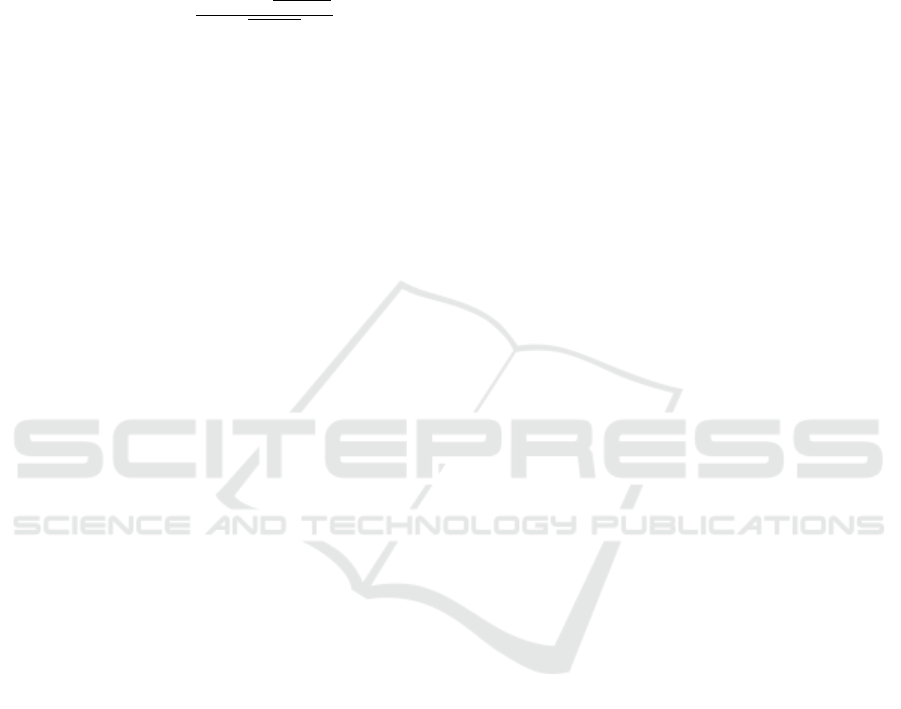
cally significant. If MI(n, c) is less than zero, then n
and c are called complementary.
The t-score also takes into account the frequency
of occurrence of a keyword and its combination, an-
swering the question of how non-random the strength
of the association between the word combinations is:
t − score =
f (n, c) −
f (n)· f (c)
N
p
f (n, c)
(2)
4 IDENTIFICATION OF WORD
COMBINATIONS BASED ON
THE STATISTICAL METHOD
The aim of the work is a comparative analysis of dif-
ferent associative measures based on the corpus of the
Kazakh language. In addition, the dependence of the
results (the list of word combinations derived from
the same measure) on the text material (text type) is
investigated.
Our dataset includes a parallel Russian-Kazakh
corpus, which has been developed over three years
(Khairova et al., 2019), and an XML dictionary
of synonyms with criminally related vocabulary
(Khairova et al., 2021). The parallel Kazakh-Russian
corpus includes texts from four news sites of the
Kazakh information Internet space zakon.kz, cara-
van.kz, lenta.kz, nur.kz for the period from April 2018
to June 2021.
At the moment the volume of the parallel Kazakh-
Russian corpus is 3000 texts in Russian and 3000
in Kazakh, including two thousand texts containing
agreed Kazakh-Russian sentences.
We extracted the vocabulary for our XML dic-
tionary of synonyms manually from the English,
Ukrainian, Kazakh and Russian texts on criminal mat-
ters. Seven main thematic categories were identified
for the terms, Movement, Traffic Accident, Injure,
Offense, Arrest, Trial, PD. The choice of categories
was due to the fact that the information resources
from which the texts were taken contained the largest
amount of data on the three criminal areas of “Po-
lice”, “Transfer”, “Crime” and their aforementioned
subspecies. This made it possible to make our dictio-
nary narrowly focused. All terms were also divided
by parts of speech, i.e. only nouns, verbs, adjectives
and word combinations were included in the dictio-
nary. Figure 1 shows a fragment of the dictionary,
which now includes about 650 basic words (over 320
nouns, over 100 adjectives, about 170 verbs and 40
word combinations) and over 2500 synonyms. It is
currently still under active development.
Our study was based on the corpus of news texts
“nur.kz”, “zakon.kz”, “patrul.kz”, “caravan.kz”, “in-
form.kz”, which includes 857 texts.
Table 1 contains data for 15 word combinations
with word “police” sorted by value of MI parame-
ter. The columns of the table, in addition to the word
combination itself, show the following characteris-
tics: Freq Word 1 & Word 2 – frequency of matching,
Freq Word 1 – frequency of word combination, Freq
Word 2 – key word, MI – MI value, T-score – t-score
value.
The analysis of the data in table 1 (15 word com-
binations in total) shows that the ranks of the word
combinations obtained using different indicators do
not coincide.
It should also be noted that different dimensions
affect the frequency of the words composing a word
combination and the frequency of their combinabil-
ity. Thus, MI is considered to be sensitive to low-
frequency words, while t-score is useful for finding
high-frequency word combinations.
We compared the automatically generated word
combinations on different association indices with
data from different dictionaries. The mate-
rial served as collections of 2 nouns without
homonyms (sozdik.kz) and 1 adjective (Kazakh-
Russian, Russian-Kazakh Terminological Dictionary.
Jurisprudence).
We call the above word combinations “correct”.
Below are graphs showing MI values, t-score mea-
surements on the ordinate axis and bigram ranks on
the abscissa axis. Black colour indicates “correct”
word combinations from the dictionary “sozdik.kz”
(4, 10 ranks) and “Kazakh-Russian, Russian-Kazakh
terminological dictionary. Jurisprudence” (rank 7) in-
dicates an additional phrase found in the dictionary.
The same tendency is observed for all obtained
word combinations: the smaller the value of the
measure, the greater the probability that these word
combinations will not be registered as regular word
combinations in dictionaries of the Kazakh language.
Thus, we can say that the compatibility data given in
dictionaries corresponds to the data obtained on the
basis of associative measures.
As a result of the experiment, it seems important
to identify phrases that are not registered in any of the
dictionaries. The analysis of such word combinations
shows that the bigrams located at the top of the list by
degree of probability (sorted in descending order of
one of the dimensions) turn out to be stable, so they
can be included in the list.
As mentioned above, other statistical criterion
methods based on linguistic models should also work.
This idea has been adopted and implemented in the fa-
Experimental Verification of Collocation Detection Methods
15