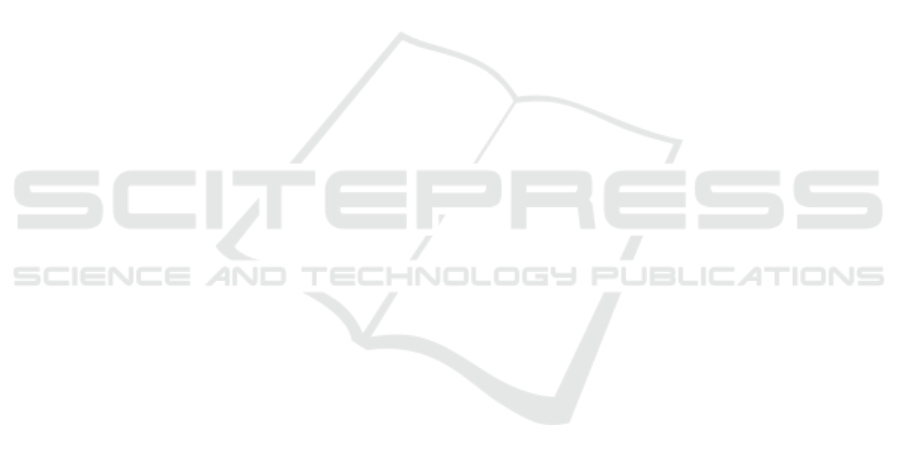
6 CONCLUSION
In this paper, we revisit the MLE estimate of the
local intrinsic dimensionality which has been used in
previous works on adversarial detection. An analysis
of the extracted LID features and their theoretical
properties allows us to redefine an LID-based feature
using unfolded local growth rate estimates that are
significantly more discriminative than the aggregated
LID measure.
Limitations. While our method allows to achieve al-
most perfect to perfect results in the considered test
scenario and for the given datasets, we do not claim
to have solved the actual problem. We use the evalu-
ation setting as proposed in previous works (e.g.(Ma
et al., 2018)) where each attack method is evaluated
separately and with constant attack parameters. For a
deployment in real-world scenarios, the robustness of
a detector under potential disguise mechanisms needs
to be verified. An extended study on the transfer-
ability of our method from one attack to the other
can be found in the supplementary material. It shows
first promising resulting in this respect but also leaves
room for further improvement.
REFERENCES
Amsaleg, L., Bailey, J., Barbe, A., Erfani, S. M., Furon, T.,
Houle, M. E., Radovanovi
´
c, M., and Nguyen, X. V.
(2021). High intrinsic dimensionality facilitates ad-
versarial attack: Theoretical evidence. IEEE Transac-
tions on Information Forensics and Security, 16:854–
865.
Amsaleg, L., Chelly, O., Furon, T., Girard, S., Houle, M. E.,
Kawarabayashi, K.-i., and Nett, M. (2015). Estimat-
ing local intrinsic dimensionality. In SIGKDD, page
29–38, New York, NY, USA. Association for Comput-
ing Machinery.
Andriushchenko, M., Croce, F., Flammarion, N., and Hein,
M. (2020). Square attack: a query-efficient black-box
adversarial attack via random search. In ECCV.
Carlini, N. and Wagner, D. (2017a). Magnet and ”efficient
defenses against adversarial attacks” are not robust to
adversarial examples.
Carlini, N. and Wagner, D. A. (2017b). Towards evaluating
the robustness of neural networks. IEEE Symposium
on Security and Privacy (SP), pages 39–57.
Croce, F. and Hein, M. (2020a). Minimally distorted adver-
sarial examples with a fast adaptive boundary attack.
In ICML.
Croce, F. and Hein, M. (2020b). Reliable evaluation of
adversarial robustness with an ensemble of diverse
parameter-free attacks. In ICML.
Evtimov, I., Eykholt, K., Fernandes, E., Kohno, T., Li, B.,
Prakash, A., Rahmati, A., and Song, D. (2017). Ro-
bust physical-world attacks on deep learning models.
CVPR.
Feinman, R., Curtin, R. R., Shintre, S., and Gardner, A. B.
(2017). Detecting adversarial samples from artifacts.
ICML, abs/1703.00410.
Goodfellow, I., Shlens, J., and Szegedy, C. (2015). Ex-
plaining and harnessing adversarial examples. ICLR,
abs/1412.6572.
Harder, P., Pfreundt, F.-J., Keuper, M., and Keuper, J.
(2021). Spectraldefense: Detecting adversarial attacks
on cnns in the fourier domain. In IJCNN.
Houle, M. E. (2017a). Local intrinsic dimensionality i: An
extreme-value-theoretic foundation for similarity ap-
plications. In Beecks, C., Borutta, F., Kr
¨
oger, P., and
Seidl, T., editors, Similarity Search and Applications,
pages 64–79, Cham. Springer International Publish-
ing.
Houle, M. E. (2017b). Local intrinsic dimensionality ii:
Multivariate analysis and distributional support. In
Beecks, C., Borutta, F., Kr
¨
oger, P., and Seidl, T., edi-
tors, Similarity Search and Applications, pages 80–95,
Cham. Springer International Publishing.
Houle, M. E., Kashima, H., and Nett, M. (2012). Gener-
alized expansion dimension. In IEEE 12th Interna-
tional Conference on Data Mining Workshops, pages
587–594.
Karger, D. R. and Ruhl, M. (2002). Finding nearest neigh-
bors in growth-restricted metrics. In Proceedings of
the Thiry-Fourth Annual ACM Symposium on Theory
of Computing, page 741–750, New York, NY, USA.
Association for Computing Machinery.
Kurakin, A., Goodfellow, I., and Bengio, S. (2017). Adver-
sarial examples in the physical world. In ICLR.
Lee, K., Lee, K., Lee, H., and Shin, J. (2018). A simple uni-
fied framework for detecting out-of-distribution sam-
ples and adversarial attacks. In NeurIPS.
Lorenz, P., Harder, P., Straßel, D., Keuper, M., and Keu-
per, J. (2021). Detecting autoattack perturbations in
the frequency domain. In ICML 2021 Workshop on
Adversarial Machine Learning.
Lorenz, P., Strassel, D., Keuper, M., and Keuper, J. (2022).
Is robustbench/autoattack a suitable benchmark for
adversarial robustness? In The AAAI-22 Workshop
on Adversarial Machine Learning and Beyond.
Ma, X., Li, B., Wang, Y., Erfani, S., Wijewickrema, S.,
Houle, M., Schoenebeck, G., Song, D., and Bailey,
J. (2018). Characterizing adversarial subspaces using
local intrinsic dimensionality. ICLR, abs/1801.02613.
Madry, A., Makelov, A., Schmidt, L., Tsipras, D., and
Vladu, A. (2018). Towards deep learning models re-
sistant to adversarial attacks. ICLR, abs/1706.06083.
Moosavi-Dezfooli, S.-M., Fawzi, A., and Frossard, P.
(2016). Deepfool: A simple and accurate method to
fool deep neural networks. CVPR, pages 2574–2582.
Rice, L., Wong, E., and Kolter, Z. (2020). Overfitting in
adversarially robust deep learning. In ICML, pages
8093–8104. PMLR.
Sharif, M., Bhagavatula, S., Bauer, L., and Reiter, M. K.
(2019). A general framework for adversarial examples
Unfolding Local Growth Rate Estimates for (Almost) Perfect Adversarial Detection
35