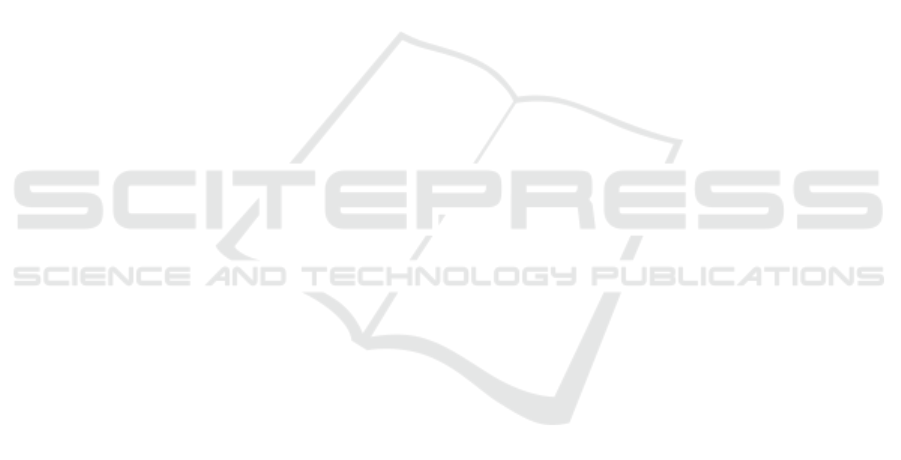
men cells classification using linear discriminant anal-
ysis on higher order spectra features of cell shape. Pat-
tern Recognition Letters, 125:534–541.
Apte, C., Grossman, E., Pednault, E. P., Rosen, B. K.,
Tipu, F. A., and White, B. (1999). Probabilistic
estimation-based data mining for discovering insur-
ance risks. IEEE Intelligent Systems and their Appli-
cations, 14(6):49–58.
Boutell, M. R., Luo, J., Shen, X., and Brown, C. M.
(2004). Learning multi-label scene classification. Pat-
tern recognition, 37(9):1757–1771.
Dem
ˇ
sar, J. (2006). Statistical comparisons of classifiers
over multiple data sets. The Journal of Machine
Learning Research, 7:1–30.
Fernandes, S. E., Pereira, D. R., Ramos, C. C., Souza,
A. N., Gastaldello, D. S., and Papa, J. P. (2018). A
probabilistic optimum-path forest classifier for non-
technical losses detection. IEEE Transactions on
Smart Grid, 10(3):3226–3235.
Gorman, R. P. and Sejnowski, T. J. (1988). Analysis of
hidden units in a layered network trained to classify
sonar targets. Neural networks, 1(1):75–89.
Huang, Y. and Meng, S. (2019). Automobile insurance clas-
sification ratemaking based on telematics driving data.
Decision Support Systems, 127:113156.
Kuncheva, L. I. (2006). On the optimality of na
¨
ıve bayes
with dependent binary features. Pattern Recognition
Letters, 27(7):830–837.
Lin, H.-T., Lin, C.-J., and Weng, R. C. (2007). A note
on platt’s probabilistic outputs for support vector ma-
chines. Machine Learning, 68(3):267–276.
Lupi Filho, G. (2012). Comparac¸
˜
ao entre os crit
´
erios
de diagn
´
osticos por an
´
alise cromatogr
´
afica de gases
dissolvidos em
´
oleo isolante de transformador de
pot
ˆ
encia. PhD thesis, Universidade de S
˜
ao Paulo.
Ma, J., Xiao, B., and Deng, C. (2020). Graph based
semi-supervised classification with probabilistic near-
est neighbors. Pattern Recognition Letters, 133:94–
101.
Madzarov, G., Gjorgjevikj, D., and Chorbev, I. (2009).
A multi-class svm classifier utilizing binary decision
tree. Informatica, 33(2).
Nagabhushan, P. and Pai, R. M. (1999). Modified region de-
composition method and optimal depth decision tree
in the recognition of non-uniform sized characters–
an experimentation with kannada characters. Pattern
Recognition Letters, 20(14):1467–1475.
Nemenyi, P. (1963). Distribution-free Multiple Compar-
isons. Princeton University.
Papa, J. P., Falc
˜
ao, A. X., Albuquerque, V. H. C., and
Tavares, J. M. R. S. (2012). Efficient supervised
optimum-path forest classification for large datasets.
Pattern Recognition, 45(1):512–520.
Papa, J. P., Falc
˜
ao, A. X., and Suzuki, C. T. N. (2009). Su-
pervised pattern classification based on optimum-path
forest. International Journal of Imaging Systems and
Technology, 19(2):120–131.
Papa, J. P., Fernandes, S. E. N., and Falc
˜
ao, A. X. (2017).
Optimum-Path Forest based on k-connectivity: The-
ory and Applications. Pattern Recognition Letters,
87:117–126.
Pedregosa, F., Varoquaux, G., Gramfort, A., Michel, V.,
Thirion, B., Grisel, O., Blondel, M., Prettenhofer,
P., Weiss, R., Dubourg, V., Vanderplas, J., Passos,
A., Cournapeau, D., Brucher, M., Perrot, M., and
Duchesnay, E. (2011). Scikit-learn: Machine learning
in Python. Journal of Machine Learning Research,
12:2825–2830.
Platt, J. C. (1999). Probabilistic outputs for support vector
machines and comparisons to regularized likelihood
methods. In Advances in Large Margin Classifiers,
pages 61–74. MIT Press.
Rocha, L. M., Cappabianco, F. A. M., and Falc
˜
ao, A. X.
(2009). Data clustering as an optimum-path forest
problem with applications in image analysis. Inter-
national Journal of Imaging Systems and Technology,
19(2):50–68.
Sanmartin, E. F., Damrich, S., and Hamprecht, F. A.
(2019). Probabilistic watershed: Sampling all
spanning forests for seeded segmentation and semi-
supervised learning. In Advances in Neural Informa-
tion Processing Systems, pages 2776–2787.
Souza, R. W. R., Oliveira, J. V. C., Passos, L. A., Ding, W.,
Papa, J. P., and Albuquerque, V. H. (2019). A novel
approach for optimum-path forest classification using
fuzzy logic. IEEE Transactions on Fuzzy Systems.
Vapnik, V. N. (1999). An overview of statistical learn-
ing theory. IEEE transactions on neural networks,
10(5):988–999.
Wilcoxon, F. (1945). Individual comparisons by ranking
methods. Biometrics Bulletin, 1(6):80–83.
Yang, Y. and Loog, M. (2018). A benchmark and compar-
ison of active learning for logistic regression. Pattern
Recognition, 83:401–415.
VISAPP 2023 - 18th International Conference on Computer Vision Theory and Applications
368