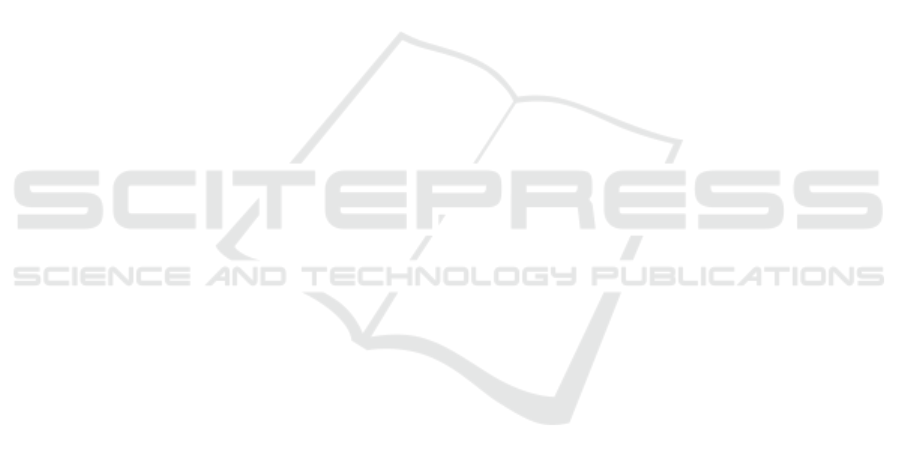
meaning, such as a fall.
For example, the system detect falls based on the
location and activation of motion sensors. Falls are
particularly dangerous for elderly people and rapid
detection of them is important in minimising the dam-
age caused by them. The system is also able to detect
significant changes in behaviour. For example, if a
person usually leaves his or her bed fewer than two
times a night but starts leaving it much more than that,
that may be indicative of an acute condition, for ex-
ample a urinary tract infection requiring them to visit
the bathroom multiple times. Of course there may be
any number of other explanations but unusual changes
in behaviour may well be significant and require some
form of intervention by a carer.
One of the areas of research we have been explor-
ing is predicting falls based on sensor activations. Are
there patterns of sensor activation, such as might be
generated by broken or restless sleep, that are predic-
tive of falls the following day? Being able to deter-
mine that a fall is more likely than normal could be
particularly useful in preventing them or in making
them less likely. For example if the system suggested
that a fall was quite likely on a particular day, the per-
son might modify their schedule so that they are less
likely to have a fall, or make the consequences of a
fall less damaging.
However, research in this area is very difficult be-
cause there are many obstacles to obtaining any useful
data. Trials of AALs during which falls are likely are
difficult to arrange, have ethical implications and re-
quire a reasonable length of time and number of par-
ticipants. Correlating any falls with sensor activations
requires the participant to recall accurately when the
fall occured. Also, although the consequences of falls
can be devastating, they are, fortunately, quite rare
amongst people living alone.
Consequently, when we ran an ethically approved
major trial of HalleyAssist we took the opportunity
to ask participants to record the date, time and loca-
tion of any falls. The primary purpose of the trial was
to assess the acceptance of the system. We certainly
were not hoping for falls. However, if falls occurred
we wanted to be in a position to analyse the data as-
sociated with them. To that end we asked participants
of the trial to keep a ’falls diary’ where they recorded
when and where any falls happened.
The trial ran for ten months starting 28th February
2020 till 31st December 2020.
During this time, one of the participants, an el-
derly woman who had a history of falls, fell 31 times
during the trial. We have used her records of when
falls occured and records from HalleyAssist to see if
it is possible to see if there is any predictive power
in sensor activations. In this initial trial we explored
the association between a poor night’s sleep and the
likelihood of a fall the following day. Poor sleep has
been associated with falls (Noh et al., 2017). We were
interested in whether sensors indicating a poor night’s
sleep could be used to predict an increased likelihood
of a fall the following day.
Based on the sensor activations we can success-
fully predict days when a fall will occur 61% of the
time and days when a fall will not occur 65% of the
time.
We think these results are interesting but acknowl-
edge that they are hardly conclusive. There are many
caveats associated with these results which we discuss
later in the paper. Nevertheless, they are encouraging
and we plan to continue with further collection and
analysis of data.
The remainder of this paper is structured as fol-
lows. Section 2 discusses related research in this area.
Section 3 describes the trials and their outcomes. Sec-
tion 4 provides an analysis of the sensor and fall data.
Finally, Section 5 is our conclusion where we sum-
marise our work and discuss future research.
2 RELATED RESEARCH
Ambient Assisted Living Systems (AALs) have at-
tracted a great deal of research attention over the past
ten years (Forkan et al., 2019a; Demiris and Hensel,
2008; Forkan et al., 2019b). These systems are an
application of the Internet of Things where data from
sensors is processed by a central hub or a cloud pro-
cess which then issues commands to actuators to con-
trol the living environment in an unobtrusive manner.
HalleyAssist is a AAL system that helps older peo-
ple remain living in their own home or in a minimal
supported accommodation by providing unobtrusive
assistance and monitoring (Forkan et al., 2019b).
As well as supporting day to day activities such
as providing lighting, heating and cooling AALs can
also provide reminders of appointments, notification
of the weather as well as reminders to take medi-
cation, eat sufficient food and drink sufficient water
(Demiris and Hensel, 2008).
However, they can also be used to identify events
and trends that a carer should be notified of. So for
example, long term trends such as isolation, wander-
ing or sleeplessness might be detected and the carer
informed. Such changes might be due to chronic or
acute illnesses such as dementia or infection or they
might report on some particular event requiring im-
mediate response, the most common of which is a fall
(Forkan et al., 2019b).
Fall Prediction Amongst the Elderly Using Data from an Ambient Assisted Living System
219