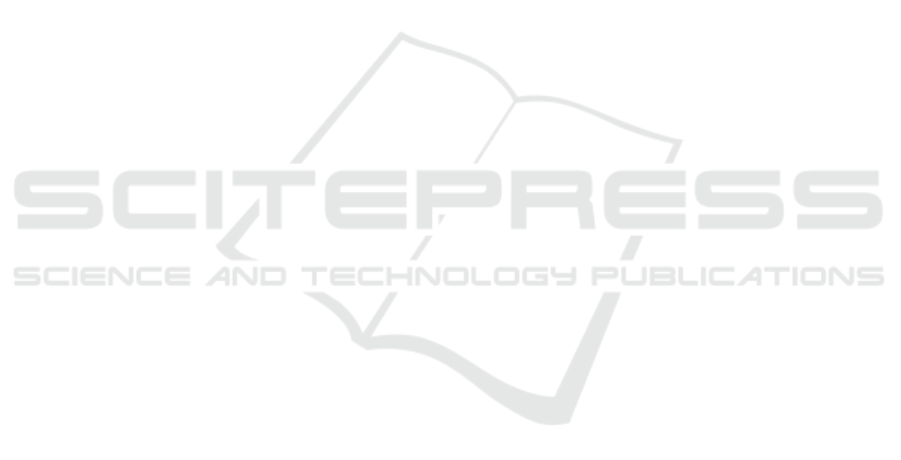
Hendrycks, D. and Gimpel, K. (2017). A baseline for de-
tecting misclassified and out-of-distribution examples
in neural networks. ICLR.
Holzinger, A. (2016). Interactive machine learning for
health informatics: when do we need the human-in-
the-loop? Brain Informatics, 3(2):119–131.
Hu, Y. and Khan, L. (2021). Uncertainty-aware reli-
able text classification. In Proceedings of the 27th
ACM SIGKDD Conference on Knowledge Discovery
& Data Mining, pages 628–636.
Joulin, A., Grave,
´
E., Bojanowski, P., and Mikolov, T.
(2017). Bag of tricks for efficient text classification.
In Proceedings of the 15th Conference of the Euro-
pean Chapter of the Association for Computational
Linguistics: Volume 2, Short Papers, pages 427–431.
Karmakharm, T., Aletras, N., and Bontcheva, K. (2019).
Journalist-in-the-loop: Continuous learning as a ser-
vice for rumour analysis. In Proceedings of the 2019
Conference on Empirical Methods in Natural Lan-
guage Processing and the 9th International Joint Con-
ference on Natural Language Processing (EMNLP-
IJCNLP): System Demonstrations, pages 115–120.
Keim, D., Andrienko, G., Fekete, J.-D., G
¨
org, C., Kohlham-
mer, J., and Melanc¸on, G. (2008). Visual analytics:
Definition, process, and challenges. In Information
visualization, pages 154–175. Springer.
Kim, Y. (2014). Convolutional neural networks for sentence
classification. In Proceedings of the 2014 Conference
on Empirical Methods in Natural Language Process-
ing (EMNLP), pages 1746–1751, Doha, Qatar. Asso-
ciation for Computational Linguistics.
Kivlichan, I., Lin, Z., Liu, J., and Vasserman, L. (2021).
Measuring and improving model-moderator collabo-
ration using uncertainty estimation. In Proceedings
of the 5th Workshop on Online Abuse and Harms
(WOAH 2021), pages 36–53.
Knaeble, M., Nadj, M., and Maedche, A. (2020). Oracle or
teacher? a systematic overview of research on inter-
active labeling for machine learning. In Wirtschaftsin-
formatik (Zentrale Tracks), pages 2–16.
Lewis, D. D. and Gale, W. A. (1994). A sequential algo-
rithm for training text classifiers. In SIGIR’94, pages
3–12. Springer.
Li, Y., Chen, J., and Feng, L. (2012). Dealing with un-
certainty: A survey of theories and practices. IEEE
Transactions on Knowledge and Data Engineering,
25(11):2463–2482.
Minaee, S., Kalchbrenner, N., Cambria, E., Nikzad, N.,
Chenaghlu, M., and Gao, J. (2021). Deep learning–
based text classification: A comprehensive review.
ACM Computing Surveys (CSUR), 54(3):1–40.
Ovadia, Y., Fertig, E., Ren, J., Nado, Z., Sculley, D.,
Nowozin, S., Dillon, J., Lakshminarayanan, B., and
Snoek, J. (2019). Can you trust your model’s uncer-
tainty? evaluating predictive uncertainty under dataset
shift. Advances in neural information processing sys-
tems, 32.
Pavlopoulos, J., Malakasiotis, P., and Androutsopoulos, I.
(2017). Deep learning for user comment moderation.
ACL 2017, page 25.
Ribeiro, M. T., Wu, T., Guestrin, C., and Singh, S. (2020).
Beyond accuracy: Behavioral testing of nlp models
with checklist. In Proceedings of the 58th Annual
Meeting of the Association for Computational Lin-
guistics, pages 4902–4912.
Roelofs, R., Shankar, V., Recht, B., Fridovich-Keil, S.,
Hardt, M., Miller, J., and Schmidt, L. (2019). A meta-
analysis of overfitting in machine learning. Advances
in Neural Information Processing Systems, 32.
Sacha, D., Senaratne, H., Kwon, B. C., Ellis, G., and Keim,
D. A. (2015). The role of uncertainty, awareness, and
trust in visual analytics. IEEE transactions on visual-
ization and computer graphics, 22(1):240–249.
Sacha, D., Stoffel, A., Stoffel, F., Kwon, B. C., Ellis, G., and
Keim, D. A. (2014). Knowledge generation model for
visual analytics. IEEE transactions on visualization
and computer graphics, 20(12):1604–1613.
Sebastiani, F. (2002). Machine learning in automated
text categorization. ACM computing surveys (CSUR),
34(1):1–47.
Settles, B. (1995). Active learning literature survey. Sci-
ence, 10(3):237–304.
Sun, Y., Wong, A. K., and Kamel, M. S. (2009). Classifica-
tion of imbalanced data: A review. International jour-
nal of pattern recognition and artificial intelligence,
23(04):687–719.
Tropmann-Frick, M. and Andersen, J. S. (2019). To-
wards visual data science-an exploration. In Interna-
tional Conference on Human Interaction and Emerg-
ing Technologies, pages 371–377. Springer.
Wang, Z. J., Choi, D., Xu, S., and Yang, D. (2021). Putting
humans in the natural language processing loop: A
survey. In Proceedings of the First Workshop on
Bridging Human–Computer Interaction and Natural
Language Processing, pages 47–52.
Waseem, Z. (2016). Are you a racist or am i seeing things?
annotator influence on hate speech detection on twit-
ter. In Proceedings of the first workshop on NLP and
computational social science, pages 138–142.
Wu, X., Xiao, L., Sun, Y., Zhang, J., Ma, T., and He, L.
(2022). A survey of human-in-the-loop for machine
learning. Future Generation Computer Systems.
Yang, Z., Dai, Z., Yang, Y., Carbonell, J., Salakhutdinov,
R. R., and Le, Q. V. (2019). Xlnet: Generalized au-
toregressive pretraining for language understanding.
Advances in neural information processing systems,
32.
Zhang, X., Chen, F., Lu, C.-T., and Ramakrishnan, N.
(2019). Mitigating uncertainty in document classifi-
cation. In Proceedings of the 2019 Conference of the
North American Chapter of the Association for Com-
putational Linguistics: Human Language Technolo-
gies, volume 1, pages 3126–3136.
Zhao, P., Zhang, Y.-J., and Zhou, Z.-H. (2021). Exploratory
machine learning with unknown unknowns. In Pro-
ceedings of the AAAI Conference on Artificial Intelli-
gence, volume 35, pages 10999–11006.
ICAART 2023 - 15th International Conference on Agents and Artificial Intelligence
24