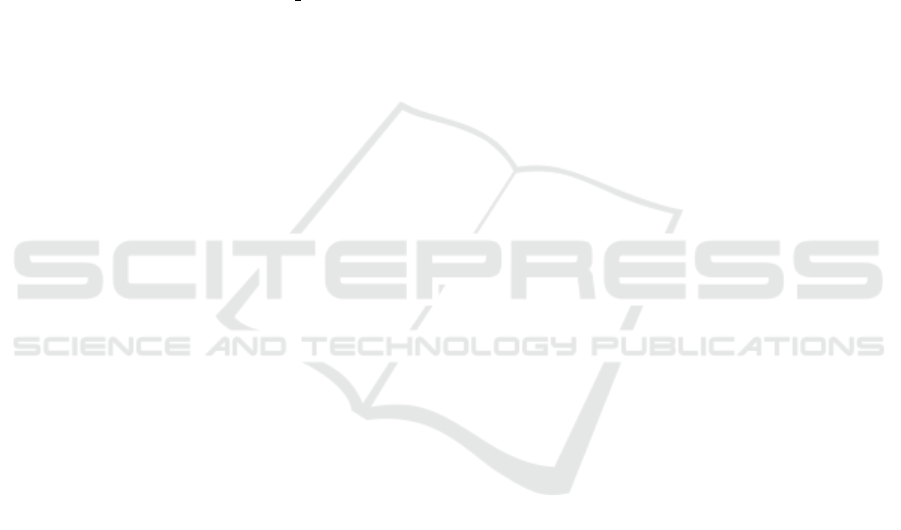
domain. IEEE Transactions on Image Processing,
21(12):4695–4708.
Neubert, T., Kraetzer, C., and Dittmann, J. (2019). A Face
Morphing Detection Concept with a Frequency and a
Spatial Domain Feature Space for Images on eMRTD.
In Proceedings of the ACM Workshop, pages 95–100.
Neubert, T., Makrushin, A., Hildebrandt, M., Kraetzer, C.,
and Dittmann, J. (2018). Extended stirtrace bench-
marking of biometric and forensic qualities of mor-
phed face images. IET Biometrics, 7(4):325–332.
NIST (2020). International Face Performance
Conference. https://www.nist.gov/news-
events/events/2020/10/international-face-
performance-conference-ifpc-2020. (accessed:
September 1, 2022).
NIST (2022). NIST FRVT MORPH.
https://pages.nist.gov/frvt/html/frvt morph.html.
(accessed: September 1, 2022).
Phillips, P. J., Flynn, P., Scruggs, T., Bowyer, K., Chang,
J. K., Hoffman, K., Marques, J., Min, J., and Worek,
W. (2005). Overview of the Face Recognition Grand
Challenge. In Proceedings of IEEE Computer Society
Conference on Computer Vision and Pattern Recogni-
tion, volume 1, pages 947– 954.
Raghavendra, R., Raja, K. B., and Busch, C. (2016). Detect-
ing morphed face images. In 2016 IEEE 8th Interna-
tional Conference on Biometrics Theory, Applications
and Systems (BTAS), pages 1–7.
Raghavendra, R., Raja, K. B., Venkatesh, S., and Busch, C.
(2017). Transferable Deep-CNN Features for Detect-
ing Digital and Print-Scanned Morphed Face Images.
2017 IEEE Conference on Computer Vision and Pat-
tern Recognition Workshops (CVPRW), pages 1822–
1830.
Raja, K., Ferrara, M., Franco, A., Spreeuwers, L., Batskos,
I., Wit, F., Gomez-Barrero, M., Scherhag, U., Fischer,
D., Venkatesh, S., Singh, J. M., Li, G., Bergeron, L.,
Isadskiy, S., Raghavendra, R., Rathgeb, C., Frings, D.,
Seidel, U., Knopjes, F., and Busch, C. (2020). Morph-
ing Attack Detection - Database, Evaluation Platform
and Benchmarking. IEEE Transactions on Informa-
tion Forensics and Security, PP:1–1.
Ramachandra, R., Venkatesh, S., Raja, K., and Busch, C.
(2019). Towards making morphing attack detection
robust using hybrid scale-space colour texture fea-
tures. In 2019 IEEE 5th International Conference
on Identity, Security, and Behavior Analysis (ISBA),
pages 1–8.
Research, T. C. and Service, D. I. (2020). Im-
age Manipulation Attack Resolving Solutions.
https://cordis.europa.eu/project/id/883356. (accessed:
September 1, 2022).
Ruiz, N., Chong, E., and Rehg, J. (2018). Fine-grained head
pose estimation without keypoints. In The IEEE Con-
ference on Conference on Computer Vision and Pat-
tern Recognition (CVPR) Workshops.
Russakovsky, O., Deng, J., Su, H., Krause, J., Satheesh,
S., Ma, S., Huang, Z., Karpathy, A., Khosla, A.,
Bernstein, M., Berg, A. C., and Fei-Fei, L. (2015).
ImageNet Large Scale Visual Recognition Challenge.
International Journal of Computer Vision (IJCV),
115(3):211–252.
Sarkar, E., Korshunov, P., Colbois, L., and Marcel, S.
(2020). Vulnerability analysis of face morphing at-
tacks from landmarks and generative adversarial net-
works. arXiv preprint.
Satya, M. (2016). Face Morph Using OpenCV.
www.learnopencv.com/face-morph-using-opencv-
cpp-python/. (accessed: September 1, 2022).
Scherhag, U., Debiasi, L., Rathgeb, C., Busch, C., and Uhl,
A. (2019). Detection of Face Morphing Attacks Based
on PRNU Analysis. IEEE Transactions on Biomet-
rics, Behavior, and Identity Science, 1(4):302–317.
Scherhag, U., Raghavendra, R., Raja, K. B., Gomez-
Barrero, M., Rathgeb, C., and Busch, C. (2017). On
the vulnerability of face recognition systems towards
morphed face attacks. In 2017 5th International Work-
shop on Biometrics and Forensics (IWBF), pages 1–6.
Scherhag, U., Rathgeb, C., and Busch, C. (2018).
Morph Deterction from Single Face Image: a Multi-
Algorithm Fusion Approach. In Proceedings of the
2018 2nd International Conference on Biometric En-
gineering and Applications, pages 6–12.
Scherhag, U., Rathgeb, C., Merkle, J., and Busch, C.
(2020). Deep Face Representations for Differential
Morphing Attack Detection. IEEE Transactions on
Information Forensics and Security, 15:3625–3639.
School of Natural Sciences University of Stirling
(1998). Psychological Image Collection of Stir-
ling. http://pics.stir.ac.uk. (accessed: September 1,
2022).
Schroff, F., Kalenichenko, D., and Philbin, J. (2015).
FaceNet: A unified embedding for face recognition
and clustering. In 2015 IEEE Conference CVPR,
pages 815–823.
Seibold, C., Samek, W., Hilsmann, A., and Eisert, P. (2017).
Detection of Face Morphing Attacks by Deep Learn-
ing. In Proceedings of Digital Forensics and Water-
marking: 16th International Workshop, IWDW 2017,
pages 107–120.
Shi, Y. and Jain, A. (2019). Probabilistic Face Embeddings.
In 2019 IEEE/CVF International Conference on Com-
puter Vision (ICCV), pages 6901–6910.
Shi, Y., Yu, X., Sohn, K., Chandraker, M., and Jain, A.
(2020). Towards Universal Representation Learning
for Deep Face Recognition. In Proceedings of 2020
IEEE/CVF Conference on Computer Vision and Pat-
tern Recognition (CVPR), pages 6816–6825.
Sun, J., Yang, W., Xue, J., and Liao, Q. (2020). An Equal-
ized Margin Loss for Face Recognition. IEEE Trans-
actions on Multimedia, pages 1–1.
Sun, Y., Chen, Y., Wang, X., and Tang, X. (2014). Deep
Learning Face Representation by Joint Identification-
Verification. In NIPS.
Sun, Y., Wang, X., and Tang, X. (2014). Deep Learning
Face Representation from Predicting 10,000 Classes.
In 2014 IEEE Conference on Computer Vision and
Pattern Recognition, pages 1891–1898.
Sun, Y., Wang, X., and Tang, X. (2015). Deeply learned
face representations are sparse, selective, and robust.
MorDeephy: Face Morphing Detection via Fused Classification
203