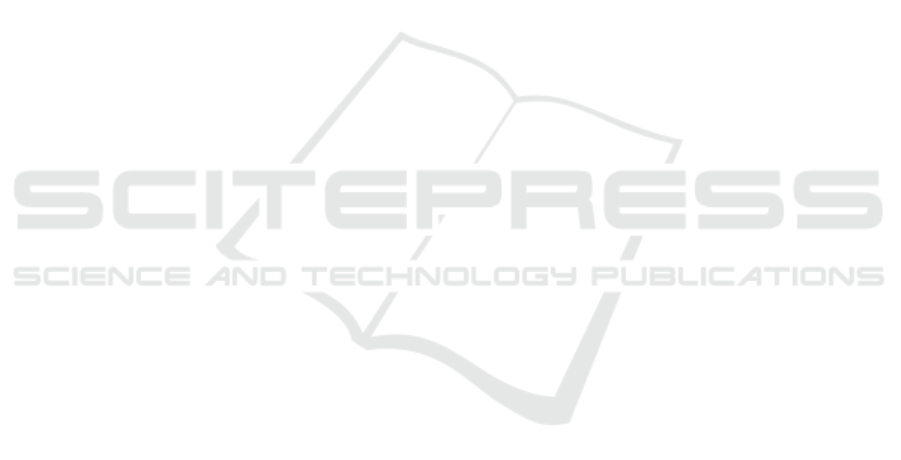
towards gaze-based models of attention during learn-
ing with technology in the classroom. In Proceedings
of the 25th Conference on User Modeling, Adaptation
and Personalization, pages 94–103.
Hwang, Y. M. and Lee, K. C. (2020). An eye-tracking
paradigm to explore the effect of online consumers’
emotion on their visual behaviour between desktop
screen and mobile screen. Behaviour & Information
Technology, pages 1–12.
Jarodzka, H., Skuballa, I., and Gruber, H. (2020). Eye-
tracking in educational practice: Investigating visual
perception underlying teaching and learning in the
classroom. Educational Psychology Review, pages 1–
10.
Jenke, L., Bansak, K., Hainmueller, J., and Hangartner, D.
(2021). Using eye-tracking to understand decision-
making in conjoint experiments. Political Analysis,
29(1):75–101.
Jiang, H. and Learned-Miller, E. (2017). Face detection
with the faster r-cnn. In 2017 12th IEEE international
conference on automatic face & gesture recognition
(FG 2017), pages 650–657. IEEE.
J
¨
onsson, E. (2005). If looks could kill–an evaluation of eye
tracking in computer games. Unpublished Master’s
Thesis, Royal Institute of Technology (KTH), Stock-
holm, Sweden.
Judd, T., Ehinger, K., Durand, F., and Torralba, A. (2009).
Learning to predict where humans look. In 2009
IEEE 12th international conference on computer vi-
sion, pages 2106–2113. IEEE.
Katsini, C., Abdrabou, Y., Raptis, G. E., Khamis, M., and
Alt, F. (2020). The role of eye gaze in security and
privacy applications: Survey and future hci research
directions. In Proceedings of the 2020 CHI Confer-
ence on Human Factors in Computing Systems, pages
1–21.
Kellnhofer, P., Recasens, A., Stent, S., Matusik, W., and
Torralba, A. (2019). Gaze360: Physically uncon-
strained gaze estimation in the wild. In Proceedings of
the IEEE/CVF International Conference on Computer
Vision, pages 6912–6921.
King, D. E. (2009). Dlib-ml: A machine learning toolkit.
The Journal of Machine Learning Research, 10:1755–
1758.
King, D. E. (2015). Max-margin object detection. arXiv
preprint arXiv:1502.00046.
Korbach, A., Ginns, P., Br
¨
unken, R., and Park, B. (2020).
Should learners use their hands for learning? results
from an eye-tracking study. Journal of Computer As-
sisted Learning, 36(1):102–113.
Krafka, K., Khosla, A., Kellnhofer, P., Kannan, H., Bhan-
darkar, S., Matusik, W., and Torralba, A. (2016). Eye
tracking for everyone. In Proceedings of the IEEE
conference on computer vision and pattern recogni-
tion, pages 2176–2184.
Kredel, R., Vater, C., Klostermann, A., and Hossner, E.-
J. (2017). Eye-tracking technology and the dynamics
of natural gaze behavior in sports: A systematic re-
view of 40 years of research. Frontiers in psychology,
8:1845.
Li, Z., Tang, X., Han, J., Liu, J., and He, R. (2019). Pyra-
midbox++: high performance detector for finding tiny
face. arXiv preprint arXiv:1904.00386.
Liu, W., Anguelov, D., Erhan, D., Szegedy, C., Reed, S.,
Fu, C.-Y., and Berg, A. C. (2016). Ssd: Single shot
multibox detector. In European conference on com-
puter vision, pages 21–37. Springer.
Lu, F., Sugano, Y., Okabe, T., and Sato, Y. (2014). Adap-
tive linear regression for appearance-based gaze esti-
mation. IEEE transactions on pattern analysis and
machine intelligence, 36(10):2033–2046.
Manning, D., Ethell, S. C., and Crawford, T. (2003). Eye-
tracking afroc study of the influence of experience
and training on chest x-ray interpretation. In Medi-
cal Imaging 2003: Image Perception, Observer Per-
formance, and Technology Assessment, volume 5034,
pages 257–266. International Society for Optics and
Photonics.
Marin-Jimenez, M. J., Zisserman, A., Eichner, M., and Fer-
rari, V. (2014). Detecting people looking at each other
in videos. International Journal of Computer Vision,
106(3):282–296.
Matsumoto, H., Terao, Y., Yugeta, A., Fukuda, H., Emoto,
M., Furubayashi, T., Okano, T., Hanajima, R., and
Ugawa, Y. (2011). Where do neurologists look when
viewing brain ct images? an eye-tracking study in-
volving stroke cases. PloS one, 6(12):e28928.
Maurage, P., Masson, N., Bollen, Z., and D’Hondt, F.
(2020). Eye tracking correlates of acute alcohol con-
sumption: A systematic and critical review. Neuro-
science & Biobehavioral Reviews, 108:400–422.
McIntyre, N. A. and Foulsham, T. (2018). Scanpath anal-
ysis of expertise and culture in teacher gaze in real-
world classrooms. Instructional Science, 46(3):435–
455.
McParland, A., Gallagher, S., and Keenan, M. (2021).
Investigating gaze behaviour of children diagnosed
with autism spectrum disorders in a classroom set-
ting. Journal of Autism and Developmental Disorders,
pages 1–16.
Meng, X., Du, R., Zwicker, M., and Varshney, A. (2018).
Kernel foveated rendering. Proceedings of the ACM
on Computer Graphics and Interactive Techniques,
1(1):1–20.
Mills, C., Bosch, N., Graesser, A., and D’Mello, S. (2014).
To quit or not to quit: predicting future behavioral dis-
engagement from reading patterns. In International
Conference on Intelligent Tutoring Systems, pages 19–
28. Springer.
Mukherjee, S. S. and Robertson, N. M. (2015). Deep head
pose: Gaze-direction estimation in multimodal video.
IEEE Transactions on Multimedia, 17(11):2094–
2107.
Oldham, J. R., Master, C. L., Walker, G. A., Meehan III,
W. P., and Howell, D. R. (2021). The association be-
tween baseline eye tracking performance and concus-
sion assessments in high school football players. Op-
tometry and Vision Science, 98(7):826–832.
Palinko, O., Rea, F., Sandini, G., and Sciutti, A. (2016).
Robot reading human gaze: Why eye tracking is bet-
GroupGazer: A Tool to Compute the Gaze per Participant in Groups with Integrated Calibration to Map the Gaze Online to a Screen or
Beamer Projection
115