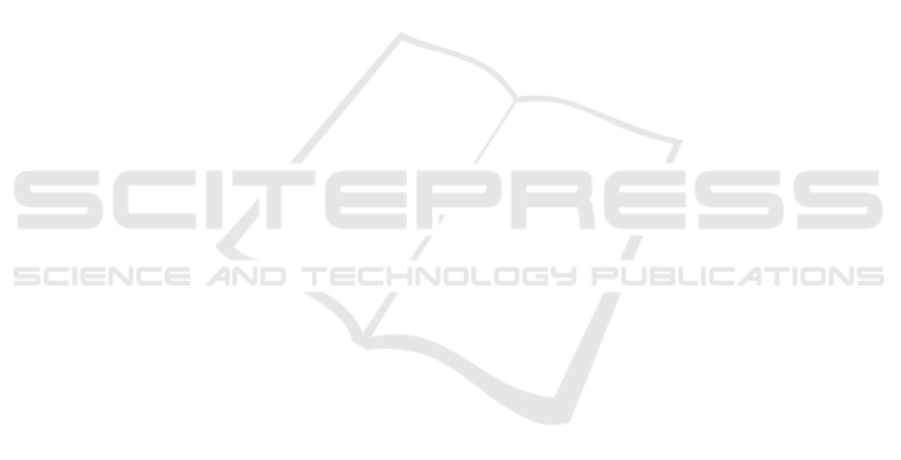
5 CONCLUSION
In this work, we proposed a domain generalization
method applicable to any semantic segmentation net-
work using monocular depth estimation, in particu-
lar reducing non-detected segments. We inferred a
depth heatmap via a modified segmentation network
that predicts foreground-background masks in paral-
lel to a semantic segmentation network. Aggregat-
ing both predictions in an uncertainty-aware manner
with a focus on important classes, false negative seg-
ments were successfully reduced. Our experiments
suggest that also in a single-sensor setup, the informa-
tion about spatial structure from pre-trained monocu-
lar depth estimators can be utilized well to improve
the robustness of off-the-shelf segmentation networks
under domain shift in various settings.
ACKNOWLEDGEMENTS
We thank M. K. Neugebauer for support in data han-
dling and programming. This work is supported by
the Ministry of Culture and Science of the German
state of North Rhine-Westphalia as part of the KI-
Starter research funding program.
REFERENCES
Adelson, E. H. (2001). On seeing stuff: the perception of
materials by humans and machines. In IS&T/SPIE
Electronic Imaging. 1
Cao, Y., Shen, C., and Shen, H. T. (2017). Exploiting
depth from single monocular images for object detec-
tion and semantic segmentation. IEEE Transactions
on Image Processing. 4
Cardace, A., Luigi, L., Zama Ramirez, P., Salti, S., and
Di Stefano, L. (2022). Plugging self-supervised
monocular depth into unsupervised domain adaptation
for semantic segmentation. 3
Chan, R., Rottmann, M., H
¨
uger, F., Schlicht, P., and
Gottschalk, H. (2020). Metafusion: Controlled false-
negative reduction of minority classes in semantic seg-
mentation. IEEE International Joint Conference on
Neural Networks (IJCNN). 3
Chen, L.-C., Zhu, Y., Papandreou, G., Schroff, F., and
Adam, H. (2018). Encoder-decoder with atrous sep-
arable convolution for semantic image segmentation.
In European Conference on Computer Vision (ECCV).
1, 2, 5
Chen, P.-Y., Liu, A. H., Liu, Y.-C., and Wang, Y.-C. F.
(2019). Towards scene understanding: Unsupervised
monocular depth estimation with semantic-aware rep-
resentation. In IEEE/CVF Conference on Computer
Vision and Pattern Recognition (CVPR). 3
Chen, W., Yu, Z., Wang, Z., and Anandkumar, A. (2020).
Automated synthetic-to-real generalization. In Inter-
national Conference on Machine Learning (ICML). 3
Choi, S., Jung, S., Yun, H., Kim, J. T., Kim, S., et al.
(2021). Robustnet: Improving domain generalization
in urban-scene segmentation via instance selective
whitening. IEEE/CVF Conference on Computer Vi-
sion and Pattern Recognition (CVPR), pages 11575–
11585. 3
Cordts, M., Omran, M., Ramos, S., Rehfeld, T., Enzweiler,
M., et al. (2016). The cityscapes dataset for semantic
urban scene understanding. In IEEE Conference on
Computer Vision and Pattern Recognition (CVPR). 1,
2, 5, 12
Friedman, J. H. (2002). Stochastic gradient boosting. Com-
put. Stat. Data Anal. 5
Geiger, A., Lenz, P., Stiller, C., and Urtasun, R. (2013).
Vision meets robotics: The kitti dataset. The Interna-
tional Journal of Robotics Research. 5
Geyer, J., Kassahun, Y., Mahmudi, M., Ricou, X., Durgesh,
R., et al. (2020). A2D2: Audi Autonomous Driving
Dataset. 2, 5, 12
Godard, C., Mac Aodha, O., Firman, M., and Brostow, G. J.
(2019). Digging into self-supervised monocular depth
prediction. 2, 5
Hazirbas, C., Ma, L., Domokos, C., and Cremers, D.
(2016). Fusenet: Incorporating depth into semantic
segmentation via fusion-based cnn architecture. In
Asian Conference on Computer Vision (ACCV). 3
He, K., Zhang, X., Ren, S., and Sun, J. (2016). Deep resid-
ual learning for image recognition. 5
Hoyer, L., Dai, D., Chen, Y., Koring, A., Saha, S., et al.
(2021). Three ways to improve semantic segmentation
with self-supervised depth estimation. 4
Huang, G., Liu, Z., and Weinberger, K. Q. (2017). Densely
connected convolutional networks. IEEE Conference
on Computer Vision and Pattern Recognition (CVPR).
5
Jaccard, P. (1912). The distribution of the flora in the alpine
zone. New Phytologist. 5
Jiang, H., Larsson, G., Maire, M., Shakhnarovich, G., and
Learned-Miller, E. G. (2018). Self-supervised relative
depth learning for urban scene understanding. In Eu-
ropean Conference on Computer Vision (ECCV). 4
Jiao, J., Cao, Y., Song, Y., and Lau, R. (2018). Look deeper
into depth: Monocular depth estimation with semantic
booster and attention-driven loss. In European Con-
ference on Computer Vision (ECCV). 3
Kim, N., Son, T., Lan, C., Zeng, W., and Kwak, S. (2021).
Wedge: Web-image assisted domain generalization
for semantic segmentation. 3
Kirillov, A., He, K., Girshick, R., Rother, C., and Dollar, P.
(2019). Panoptic segmentation. In IEEE/CVF Con-
ference on Computer Vision and Pattern Recognition
(CVPR). 4
Lee, J. H., Han, M.-K., Ko, D. W., and Suh, I. H. (2019).
From big to small: Multi-scale local planar guidance
for monocular depth estimation. 2, 5
Lee, S., Seong, H., Lee, S., and Kim, E. (2022). Wildnet:
False Negative Reduction in Semantic Segmentation Under Domain Shift Using Depth Estimation
405