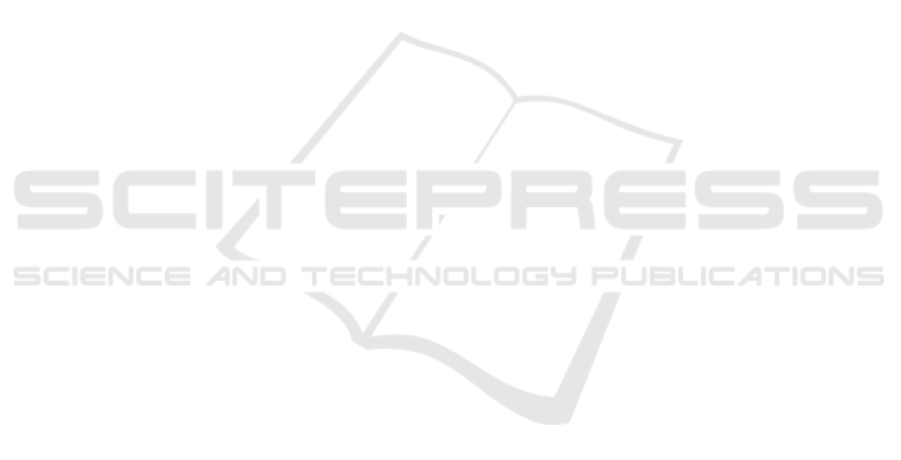
laborative filtering. Expert Systems with Applications,
83:30–39.
Lee, D., Kim, B. H., and Kim, K. J. (2010). Detecting
method on illegal use using pca under her environment.
In 2010 International Conference on Information Sci-
ence and Applications, pages 1–6. IEEE.
Liu, S., Maljovec, D., Wang, B., Bremer, P.-T., and Pas-
cucci, V. (2016). Visualizing high-dimensional data:
Advances in the past decade. IEEE transactions on vi-
sualization and computer graphics, 23(3):1249–1268.
Lu, L. and Vidal, R. (2006). Combined central and subspace
clustering for computer vision applications. pages 593–
600.
McInnes, L., Healy, J., and Melville, J. (2018). Umap:
Uniform manifold approximation and projection for di-
mension reduction. arXiv preprint arXiv:1802.03426.
Mevel, L., Hermans, L., and Van der Auweraer, H. (1999).
Application of a subspace-based fault detection method
to industrial structures. Mechanical Systems and Signal
Processing, 13(6):823–838.
Nickel, M. and Kiela, D. (2017). Poincaré embeddings
for learning hierarchical representations. Advances in
neural information processing systems, 30:6338–6347.
Novembre, J., Johnson, T., Bryc, K., Kutalik, Z., Boyko,
A. R., Auton, A., Indap, A., King, K. S., Bergmann, S.,
Nelson, M. R., et al. (2008). Genes mirror geography
within europe. Nature, 456(7218):98–101.
Parsons, L., Haque, E., and Liu, H. (2004). Subspace clus-
tering for high dimensional data: a review. Acm sigkdd
explorations newsletter, 6(1):90–105.
Recht, B. (2011). A simpler approach to matrix completion.
Journal of Machine Learning Research, 12(12).
Shaham, U. and Steinerberger, S. (2017). Stochastic neigh-
bor embedding separates well-separated clusters. arXiv
preprint arXiv:1702.02670.
Song, Y., Westerhuis, J. A., Aben, N., Michaut, M., Wessels,
L. F., and Smilde, A. K. (2019). Principal compo-
nent analysis of binary genomics data. Briefings in
bioinformatics, 20(1):317–329.
Stewart, G. W. (1998). An updating algorithm for subspace
tracking. Technical report.
Sun, W., Zhang, L., Du, B., Li, W., and Lai, Y. M. (2015).
Band selection using improved sparse subspace clus-
tering for hyperspectral imagery classification. IEEE
Journal of Selected Topics in Applied Earth Observa-
tions and Remote Sensing, 8(6):2784–2797.
Tang, J., Liu, J., Zhang, M., and Mei, Q. (2016). Visualizing
large-scale and high-dimensional data. In Proceedings
of the 25th international conference on world wide
web, pages 287–297.
Tenenbaum, J. B., Silva, V. d., and Langford, J. C. (2000). A
global geometric framework for nonlinear dimension-
ality reduction. science, 290(5500):2319–2323.
Tomasi, C. and Kanade, T. (1992). Shape and motion
from image streams under orthography: a factoriza-
tion method. International journal of computer vision,
9(2):137–154.
Tron, R. and Vidal, R. (2007). A benchmark for the com-
parison of 3-d motion segmentation algorithms. In
2007 IEEE conference on computer vision and pattern
recognition, pages 1–8. IEEE.
Ullah, F., Sarwar, G., and Lee, S. (2014). N-screen aware
multicriteria hybrid recommender system using weight
based subspace clustering. The Scientific World Jour-
nal, 2014.
Van der Maaten, L. and Hinton, G. (2008). Visualizing data
using t-sne. Journal of machine learning research,
9(11).
Van Overschee, P. (1997). Subspace identification: Theory,
implementation, application.
Vaswani, N., Bouwmans, T., Javed, S., and Narayanamurthy,
P. (2018). Robust subspace learning: Robust pca, ro-
bust subspace tracking, and robust subspace recovery.
IEEE signal processing magazine, 35(4):32–55.
Vidal, R. and Favaro, P. (2014). Low rank subspace cluster-
ing (lrsc). Pattern Recognition Letters, 43:47–61.
Vidal, R., Tron, R., and Hartley, R. (2008). Multiframe mo-
tion segmentation with missing data using powerfactor-
ization and gpca. International Journal of Computer
Vision, 79(1):85–105.
Wu, J. and Zhang, X. (2001). A pca classifier and its ap-
plication in vehicle detection. In IJCNN’01. Interna-
tional Joint Conference on Neural Networks. Proceed-
ings (Cat. No. 01CH37222), volume 1, pages 600–604.
IEEE.
Wu, J., Zhang, X., and Zhou, J. (2001). Vehicle detec-
tion in static road images with pca-and-wavelet-based
classifier. In ITSC 2001. 2001 IEEE Intelligent Trans-
portation Systems. Proceedings (Cat. No. 01TH8585),
pages 740–744. IEEE.
Xia, C.-Q., Han, K., Qi, Y., Zhang, Y., and Yu, D.-J. (2017).
A self-training subspace clustering algorithm under
low-rank representation for cancer classification on
gene expression data. IEEE/ACM transactions on
computational biology and bioinformatics, 15(4):1315–
1324.
Xu, J., Ithapu, V. K., Mukherjee, L., Rehg, J. M., and Singh,
V. (2013). Gosus: Grassmannian online subspace
updates with structured-sparsity. In Proceedings of
the IEEE international conference on computer vision,
pages 3376–3383.
Yang, A. Y., Wright, J., Ma, Y., and Sastry, S. S. (2008).
Unsupervised segmentation of natural images via lossy
data compression. Computer Vision and Image Under-
standing, 110(2):212–225.
Zhang, H., Ericksen, S. S., Lee, C.-p., Ananiev, G. E., Wlo-
darchak, N., Yu, P., Mitchell, J. C., Gitter, A., Wright,
S. J., Hoffmann, F. M., et al. (2019). Predicting kinase
inhibitors using bioactivity matrix derived informer
sets. PLoS computational biology, 15(8):e1006813.
Zhang, W., Wang, Q., Yoshida, T., and Li, J. (2021). Rp-
lgmc: rating prediction based on local and global in-
formation with matrix clustering. Computers & Oper-
ations Research, 129:105228.
Visualizing Grassmannians via Poincare Embeddings
37