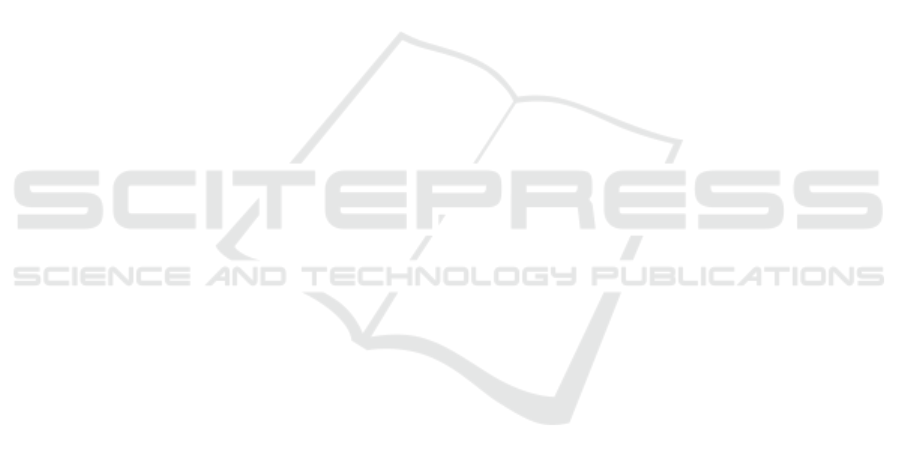
Hochreiter, S. and Schmidhuber, J. (1997). Long short-term
memory. Neural computation, 9(8):1735–1780.
Keogh, E. and Ratanamahatana, C. A. (2005). Exact index-
ing of dynamic time warping. Knowledge and infor-
mation systems, 7(3):358–386.
Kim, D. (2018). Effect of musculoskeletal pain of care
workers on job satisfaction. Journal of Physical Ther-
apy Science, 30:164–168.
Kingma, D. P. and Ba, J. (2015). Adam: A method for
stochastic optimization. ICLR.
Kok, J., Vroonhof, P., Snijders, J., Roullis, G., Clarke,
M., Peereboom, K., Dorst, P., Isusi, I., and European
Agency for Safety and Health at Work (2020). Work-
related musculoskeletal disorders : prevalence, costs
and demographics in the EU. Publications Office.
Lai, G., Chang, W.-C., Yang, Y., and Liu, H. (2018). Mod-
eling long-and short-term temporal patterns with deep
neural networks. In The 41st International ACM SI-
GIR Conference on Research & Development in In-
formation Retrieval, pages 95–104.
Lalloo, C., Jibb, L. A., Rivera, J., Agarwal, A., and Stinson,
J. N. (2015). There’s a pain app for that. The Clinical
journal of pain, 31(6):557–563.
Laptev, N., Yosinski, J., Li, L. E., and Smyl, S. (2017).
Time-series extreme event forecasting with neural net-
works at uber. In International Conference on Ma-
chine Learning, volume 34, pages 1–5.
Lee, J., Mawla, I., Kim, J., Loggia, M. L., Ortiz, A., Jung,
C., Chan, S.-T., Gerber, J., Schmithorst, V. J., Ed-
wards, R. R., et al. (2019). Machine learning-based
prediction of clinical pain using multimodal neu-
roimaging and autonomic metrics. Pain, 160(3):550.
Li, K., Liu, C., Zhu, T., Herrero, P., and Georgiou, P. (2019).
GluNet: A deep learning framework for accurate glu-
cose forecasting. IEEE journal of biomedical and
health informatics, 24(2):414–423.
Lipton, Z. C., Kale, D., and Wetzel, R. (2016). Directly
modeling missing data in sequences with RNNs: Im-
proved classification of clinical time series. In Ma-
chine Learning for Healthcare Conference, pages
253–270.
Liu, M., Zeng, A., Xu, Z., Lai, Q., and Xu, Q. (2021).
Time series is a special sequence: Forecasting with
sample convolution and interaction. arXiv preprint
arXiv:2106.09305.
L
¨
otsch, J. and Ultsch, A. (2018). Machine learning in pain
research. Pain, 159(4):623.
Makridakis, S., Spiliotis, E., and Assimakopoulos, V.
(2018). Statistical and machine learning forecasting
methods: Concerns and ways forward. PloS one,
13(3):e0194889.
McCracken, L. M. and Eccleston, C. (2005). A prospective
study of acceptance of pain and patient functioning
with chronic pain. Pain, 118(1-2):164–169.
Morlion, B., Coluzzi, F., Aldington, D., Kocot-Kepska, M.,
Pergolizzi, J., Mangas, A. C., Ahlbeck, K., and Kalso,
E. (2018). Pain chronification: what should a non-pain
medicine specialist know? Current Medical Research
and Opinion, 34(7):1169–1178.
Moseley, G. L. and Arntz, A. (2007). The context of a
noxious stimulus affects the pain it evokes. PAIN®,
133(1-3):64–71.
Nijs, J., Van Wilgen, C. P., Van Oosterwijck, J., van Itter-
sum, M., and Meeus, M. (2011). How to explain cen-
tral sensitization to patients with ‘unexplained’chronic
musculoskeletal pain: practice guidelines. Manual
therapy, 16(5):413–418.
Puri, C., Kooijman, G., Masculo, F., Van Sambeek, S.,
Den Boer, S., Luca, S., and Vanrumste, B. (2019).
PREgDICT: Early prediction of gestational weight
gain for pregnancy care. In 2019 41st Annual In-
ternational Conference of the IEEE Engineering in
Medicine and Biology Society (EMBC), pages 4274–
4278. IEEE.
Rasmussen, C. E. (2004). Gaussian processes in machine
learning. In Advanced lectures on machine learning,
pages 63–71. Springer.
Shibata, R. (1976). Selection of the order of an au-
toregressive model by akaike’s information criterion.
Biometrika, 63(1):117–126.
Shumway, R. H. and Stoffer, D. S. (2017). Time series anal-
ysis and its applications: with R examples. Springer.
Strodthoff, N. and Wagner, P. e. (2020). Deep learning for
ECG analysis: Benchmarks and insights from PTB-
XL. IEEE Journal of Biomedical and Health Infor-
matics, 25(5):1519–1528.
Tighe, P. J., Harle, C. A., Hurley, R. W., Aytug, H.,
Boezaart, A. P., and Fillingim, R. B. (2015). Teach-
ing a machine to feel postoperative pain: combining
high-dimensional clinical data with machine learning
algorithms to forecast acute postoperative pain. Pain
Medicine, 16(7):1386–1401.
Vlaeyen, J. W. and Linton, S. J. (2000). Fear-avoidance and
its consequences in chronic musculoskeletal pain: a
state of the art. Pain, 85(3):317–332.
Daily Pain Prediction in Workplace Using Gaussian Processes
247