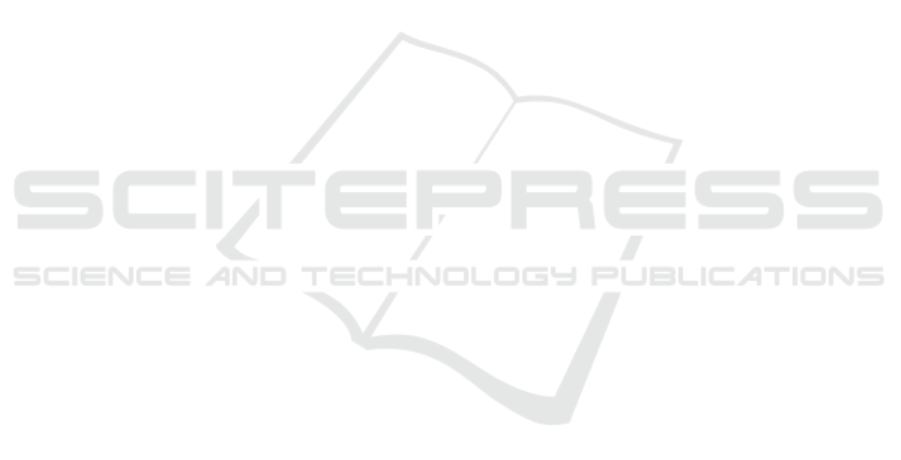
representations. In Int. conference on machine learn-
ing, pages 1597–1607. PMLR.
Chen, Y., Zhou, X., Xing, Z., Liu, Z., and Xu, M. (2022).
Cass: A channel-aware self-supervised representation
learning framework for multivariate time series classi-
fication. In Int. Conference on Database Systems for
Advanced Applications, pages 375–390. Springer.
Dau, H. A., Bagnall, A., Kamgar, K., Yeh, C.-C. M., Zhu,
Y., Gharghabi, S., Ratanamahatana, C. A., and Keogh,
E. (2019). The ucr time series archive. IEEE/CAA
Journal of Automatica Sinica, 6(6):1293–1305.
Deng, J., Dong, W., Socher, R., Li, L.-J., Li, K., and Fei-
Fei, L. (2009). Imagenet: A large-scale hierarchical
image database. In 2009 IEEE conference on com-
puter vision and pattern recognition, pages 248–255.
Ieee.
Eldele, E., Ragab, M., Chen, Z., Wu, M., Kwoh, C. K.,
Li, X., and Guan, C. (2021). Time-series representa-
tion learning via temporal and contextual contrasting.
arXiv preprint arXiv:2106.14112.
Fawaz, H. I., Forestier, G., Weber, J., Idoumghar, L., and
Muller, P.-A. (2018). Data augmentation using syn-
thetic data for time series classification with deep
residual networks. arXiv preprint arXiv:1808.02455.
Franceschi, J.-Y., Dieuleveut, A., and Jaggi, M. (2019). Un-
supervised scalable representation learning for multi-
variate time series. Advances in neural information
processing systems, 32.
Garg, Y. (2021). Retrim: Reconstructive triplet loss for
learning reduced embeddings for multi-variate time
series. In 2021 Int. Conference on Data Mining Work-
shops (ICDMW), pages 460–465. IEEE.
Grill, J.-B., Strub, F., Altch
´
e, F., Tallec, C., Richemond, P.,
Buchatskaya, E., Doersch, C., Avila Pires, B., Guo,
Z., Gheshlaghi Azar, M., et al. (2020). Bootstrap your
own latent-a new approach to self-supervised learn-
ing. Advances in Neural Information Processing Sys-
tems, 33:21271–21284.
He, K., Zhang, X., Ren, S., and Sun, J. (2016). Deep resid-
ual learning for image recognition. In Proceedings of
the IEEE conference on computer vision and pattern
recognition, pages 770–778.
Hinton, G., Vinyals, O., Dean, J., et al. (2015). Distilling
the knowledge in a neural network.
Ismail Fawaz, H., Forestier, G., Weber, J., Idoumghar, L.,
and Muller, P.-A. (2019). Deep learning for time series
classification: a review. Data mining and knowledge
discovery, 33(4):917–963.
Ismail Fawaz, H., Lucas, B., Forestier, G., Pelletier, C.,
Schmidt, D. F., Weber, J., Webb, G. I., Idoumghar, L.,
Muller, P.-A., and Petitjean, F. (2020). Inceptiontime:
Finding alexnet for time series classification. Data
Mining and Knowledge Discovery, 34(6):1936–1962.
Kavran, D., Zalik, B., and Lukac, N. (2022). Time series
augmentation based on beta-vae to improve classifica-
tion performance. In ICAART (2), pages 15–23.
Lafabregue, B., Weber, J., Ganc¸arski, P., and Forestier, G.
(2022). End-to-end deep representation learning for
time series clustering: a comparative study. Data Min-
ing and Knowledge Discovery, 36(1):29–81.
LeCun, Y., Bottou, L., Bengio, Y., and Haffner, P. (1998).
Gradient-based learning applied to document recogni-
tion. Proceedings of the IEEE, 86(11):2278–2324.
Liang, Y., Pan, Y., Lai, H., Liu, W., and Yin, J. (2021).
Deep listwise triplet hashing for fine-grained image
retrieval. IEEE Transactions on Image Processing.
Lin, L., Song, S., Yang, W., and Liu, J. (2020). Ms2l: Multi-
task self-supervised learning for skeleton based action
recognition. In Proceedings of the 28th ACM Int. Con-
ference on Multimedia, pages 2490–2498.
Mercier, D., Bhatt, J., Dengel, A., and Ahmed, S. (2022).
Time to focus: A comprehensive benchmark us-
ing time series attribution methods. arXiv preprint
arXiv:2202.03759.
Mohsenvand, M. N., Izadi, M. R., and Maes, P. (2020).
Contrastive representation learning for electroen-
cephalogram classification. In Machine Learning for
Health, pages 238–253. PMLR.
Oki, H., Abe, M., Miyao, J., and Kurita, T. (2020). Triplet
loss for knowledge distillation. In 2020 Int. Joint
Conference on Neural Networks (IJCNN), pages 1–7.
IEEE.
Peng, C. and Cheng, Q. (2020). Discriminative ridge ma-
chine: A classifier for high-dimensional data or imbal-
anced data. IEEE Transactions on Neural Networks
and Learning Systems, 32(6):2595–2609.
Pialla, G., Devanne, M., Weber, J., Idoumghar, L., and
Forestier, G. (2022a). Data augmentation for time
series classification with deep learning models. In
Advanced Analytics and Learning on Temporal Data
(AALTD), page undefined. undefined.
Pialla, G., Fawaz, H. I., Devanne, M., Weber, J., Idoumghar,
L., Muller, P.-A., Bergmeir, C., Schmidt, D., Webb,
G., and Forestier, G. (2022b). Smooth perturbations
for time series adversarial attacks. In Pacific-Asia
Conference on Knowledge Discovery and Data Min-
ing, pages 485–496. Springer.
Schroff, F., Kalenichenko, D., and Philbin, J. (2015).
Facenet: A unified embedding for face recognition
and clustering. In Proceedings of the IEEE conference
on computer vision and pattern recognition, pages
815–823.
Terefe, T., Devanne, M., Weber, J., Hailemariam, D., and
Forestier, G. (2020). Time series averaging using
multi-tasking autoencoder. In 2020 IEEE 32nd Int.
Conference on Tools with Artificial Intelligence (IC-
TAI), pages 1065–1072. IEEE.
Van der Maaten, L. and Hinton, G. (2008). Visualizing data
using t-sne. Journal of machine learning research,
9(11).
Wang, Z., Yan, W., and Oates, T. (2017). Time series clas-
sification from scratch with deep neural networks: A
strong baseline. In 2017 Int. joint conference on neu-
ral networks (IJCNN), pages 1578–1585. IEEE.
Wickstrøm, K., Kampffmeyer, M., Mikalsen, K. Ø., and
Jenssen, R. (2022). Mixing up contrastive learning:
Self-supervised representation learning for time se-
ries. Pattern Recognition Letters, 155:54–61.
Yang, X., Zhang, Z., and Cui, R. (2022). Timeclr: A self-
supervised contrastive learning framework for univari-
ate time series representation. Knowledge-Based Sys-
tems, page 108606.
Enhancing Time Series Classification with Self-Supervised Learning
47