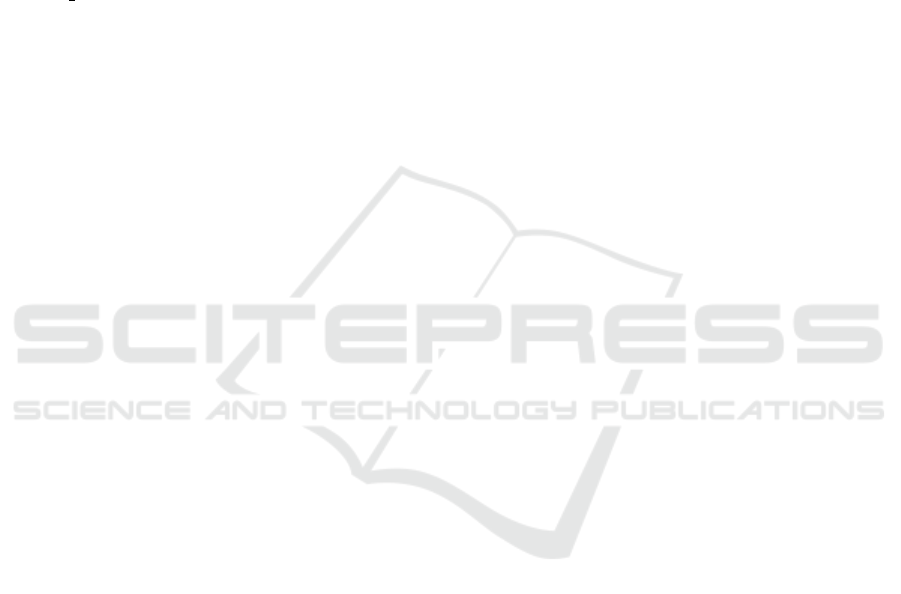
REFERENCES
Albert, J. (2007). Implementation of the Random For-
est Method for the Imaging Atmospheric Cherenkov
Telescope MAGIC. Publisher: arXiv Version Num-
ber: 2.
Becherini, Y., Djannati-Ata
¨
ı, A., Marandon, V., Punch, M.,
and Pita, S. (2011). A new analysis strategy for de-
tection of faint gamma-ray sources with Imaging At-
mospheric Cherenkov Telescopes. Publisher: arXiv
Version Number: 1.
Bernlohr, K. (2008). Simulation of Imaging Atmo-
spheric Cherenkov Telescopes with CORSIKA and
sim telarray. Publisher: arXiv Version Number: 1.
Brill, A., Feng, Q., Humensky, T. B., Kim, B., Nieto, D.,
and Miener, T. (2019). Investigating a Deep Learning
Method to Analyze Images from Multiple Gamma-ray
Telescopes. In 2019 New York Scientific Data Summit
(NYSDS), pages 1–4. arXiv:2001.03602 [astro-ph].
Chapelle, O., Scholkopf, B., and Zien, Eds., A. (2009).
Semi-Supervised Learning (Chapelle, O. et al., Eds.;
2006) [Book reviews]. IEEE Transactions on Neural
Networks, 20(3):542–542.
Developers, T. (2022). TensorFlow.
Drew, R. D. (2021). Deep unsupervised domain adaptation
for gamma-hadron separation.
Grespan, P., Jacquemont, M., L
´
opez-Coto, R., Miener, T.,
Nieto-Casta
˜
no, D., and Vuillaume, T. (2021). Deep-
learning-driven event reconstruction applied to sim-
ulated data from a single Large-Sized Telescope of
CTA. arXiv:2109.14262 [astro-ph].
Heck, D., Knapp, J., Capdevielle, J. N., Schatz, G., and
Thouw, T. (1998). CORSIKA: a Monte Carlo code
to simulate extensive air showers. Publication Title:
CORSIKA: a Monte Carlo code to simulate extensive
air showers ADS Bibcode: 1998cmcc.book.....H.
Hillas, A. M. (1985). Cerenkov light images of EAS pro-
duced by primary gamma. 3(OG-9.5-3):4.
Jacquemont, M., Vuillaume, T., Benoit, A., Maurin, G.,
Lambert, P., and Lamanna, G. (2021). First Full-
Event Reconstruction from Imaging Atmospheric
Cherenkov Telescope Real Data with Deep Learn-
ing. In 2021 International Conference on Content-
Based Multimedia Indexing (CBMI), pages 1–6, Lille,
France. IEEE.
Jacquemont, M., Vuillaume, T., Benoit, A., Maurin, G.,
Lambert, P., Lamanna, G., and Brill, A. (2019). Gam-
maLearn: A Deep Learning Framework for IACT
Data. In Proceedings of 36th International Cosmic
Ray Conference — PoS(ICRC2019), page 705, Madi-
son, WI, U.S.A. Sissa Medialab.
Jury
ˇ
sek, J., Lyard, E., and Walter, R. (2021). Full LST-1
data reconstruction with the use of convolutional neu-
ral networks. arXiv:2111.14478 [astro-ph].
Krause, M., Pueschel, E., and Maier, G. (2017). Improved
$\gamma$/hadron separation for the detection of faint
gamma-ray sources using boosted decision trees. As-
troparticle Physics, 89:1–9. arXiv:1701.06928 [astro-
ph].
Laurens Van der Maaten and Hinton, G. (2008). Visualizing
data using t-SNE. 9(11).
Lee, D.-H. (2013). Pseudo-Label : The Simple and Efficient
Semi-Supervised Learning Method for Deep Neural
Networks. Technical report.
Lu, C.-C. (2013). Improving the H.E.S.S. angular resolution
using the Disp method. arXiv:1310.1200 [astro-ph].
Mangano, S., Delgado, C., Bernardos, M., Lallena, M., and
V
´
azquez, J. J. R. (2018). Extracting gamma-ray in-
formation from images with convolutional neural net-
work methods on simulated Cherenkov Telescope Ar-
ray data, volume 11081. arXiv:1810.00592 [astro-
ph].
McInnes, L., Healy, J., and Melville, J. (2020). UMAP:
Uniform Manifold Approximation and Projection for
Dimension Reduction. arXiv:1802.03426 [cs, stat].
Miener, T., L
´
opez-Coto, R., Contreras, J. L., Green, J. G.,
Green, D., Mariotti, E., Nieto, D., Romanato, L., and
Yadav, S. (2021a). IACT event analysis with the
MAGIC telescopes using deep convolutional neural
networks with CTLearn. arXiv:2112.01828 [astro-
ph].
Miener, T., Nieto, D., Brill, A., Spencer, S., and Con-
treras, J. L. (2021b). Reconstruction of stereo-
scopic CTA events using deep learning with CTLearn.
arXiv:2109.05809 [astro-ph].
Nieto, D., Brill, A., Feng, Q., Humensky, T. B., Kim, B.,
Miener, T., Mukherjee, R., and Sevilla, J. (2019).
CTLearn: Deep Learning for Gamma-ray Astronomy.
arXiv:1912.09877 [astro-ph].
Nieto, D., Brill, A., Kim, B., and Humensky, T. B.
(2017). Exploring deep learning as an event classi-
fication method for the Cherenkov Telescope Array.
arXiv:1709.05889 [astro-ph].
Ohm, S., van Eldik, C., and Egberts, K. (2009). Gamma-
Hadron Separation in Very-High-Energy gamma-ray
astronomy using a multivariate analysis method. Pub-
lisher: arXiv Version Number: 1.
Shilon, I., Kraus, M., B
¨
uchele, M., Egberts, K., Fischer, T.,
Holch, T., Lohse, T., Schwanke, U., Steppa, C., and
Funk, S. (2019). Application of deep learning meth-
ods to analysis of imaging atmospheric Cherenkov
telescopes data. Astroparticle Physics, 105:44–53.
Smith, L. N. (2017). Cyclical Learning Rates for Training
Neural Networks. In 2017 IEEE Winter Conference
on Applications of Computer Vision (WACV), pages
464–472, Santa Rosa, CA, USA. IEEE.
The CTA Consortium (2019). Science with the Cherenkov
Telescope Array. WORLD SCIENTIFIC.
Vuillaume, T., Jacquemont, M., de Lavergne, M. d. B.,
Sanchez, D. A., Poireau, V., Maurin, G., Benoit,
A., Lambert, P., Lamanna, G., and Project, C.-L.
(2021). Analysis of the Cherenkov Telescope Array
first Large-Sized Telescope real data using convolu-
tional neural networks. arXiv:2108.04130 [astro-ph].
Zeugmann, T., Poupart, P., Kennedy, J., Jin, X., Han,
J., Saitta, L., Sebag, M., Peters, J., Bagnell, J. A.,
Daelemans, W., Webb, G. I., Ting, K. M., Ting,
K. M., Webb, G. I., Shirabad, J. S., F
¨
urnkranz, J.,
H
¨
ullermeier, E., Matwin, S., Sakakibara, Y., Flener,
Deep Learning Semi-Supervised Strategy for Gamma/Hadron Classification of Imaging Atmospheric Cherenkov Telescope Events
731