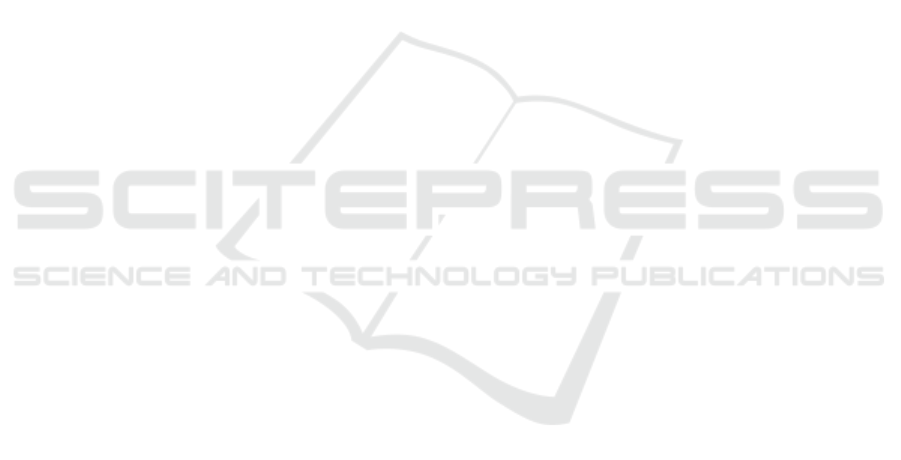
ACKNOWLEDGEMENTS
The authors would like to acknowledge the American
University of Beirut (AUB) and the National Coun-
cil for Scientific Research of Lebanon (CNRS-L) for
granting a doctoral fellowship to Maya Antoun.
REFERENCES
Baldassarre, F., Smith, K., Sullivan, J., and Azizpour, H.
(2020). Explanation-based weakly-supervised learn-
ing of visual relations with graph networks. arXiv
preprint arXiv:2006.09562.
Bansal, A., Rambhatla, S. S., Shrivastava, A., and Chel-
lappa, R. (2020a). Detecting human-object interac-
tions via functional generalization. In AAAI, pages
10460–10469.
Bansal, A., Rambhatla, S. S., Shrivastava, A., and
Chellappa, R. (2020b). Spatial priming for de-
tecting human-object interactions. arXiv preprint
arXiv:2004.04851.
Bub, D. and Masson, M. (2006). Gestural knowledge
evoked by objects as part of conceptual representa-
tions. Aphasiology, 20(9):1112–1124.
Chao, Y.-W., Liu, Y., Liu, X., Zeng, H., and Deng, J. (2018).
Learning to detect human-object interactions. In 2018
ieee winter conference on applications of computer vi-
sion (wacv), pages 381–389. IEEE.
Chen, M., Liao, Y., Liu, S., Chen, Z., Wang, F., and Qian,
C. (2021). Reformulating hoi detection as adaptive set
prediction. arXiv preprint arXiv:2103.05983.
Devlin, J., Chang, M.-W., Lee, K., and Toutanova, K.
(2018). Bert: Pre-training of deep bidirectional trans-
formers for language understanding. arXiv preprint
arXiv:1810.04805.
Fang, H.-S., Xie, S., Tai, Y.-W., and Lu, C. (2017). Rmpe:
Regional multi-person pose estimation. In Proceed-
ings of the IEEE international conference on com-
puter vision, pages 2334–2343.
Gallese, V., Fadiga, L., Fogassi, L., and Rizzolatti, G.
(1996). Action recognition in the premotor cortex.
Brain, 119(2):593–609.
Gao, C., Xu, J., Zou, Y., and Huang, J.-B. (2020). Drg: Dual
relation graph for human-object interaction detection.
In European Conference on Computer Vision, pages
696–712. Springer.
Gao, C., Zou, Y., and Huang, J.-B. (2018). ican: Instance-
centric attention network for human-object interaction
detection. arXiv preprint arXiv:1808.10437.
Gkioxari, G., Girshick, R., Doll
´
ar, P., and He, K. (2018).
Detecting and recognizing human-object interactions.
In Proceedings of the IEEE Conference on Computer
Vision and Pattern Recognition, pages 8359–8367.
He, K., Zhang, X., Ren, S., and Sun, J. (2016). Deep resid-
ual learning for image recognition. In Proceedings of
the IEEE conference on computer vision and pattern
recognition, pages 770–778.
Hou, Z., Peng, X., Qiao, Y., and Tao, D. (2020). Visual
compositional learning for human-object interaction
detection. arXiv preprint arXiv:2007.12407.
Hou, Z., Yu, B., Qiao, Y., Peng, X., and Tao, D. (2021).
Affordance transfer learning for human-object inter-
action detection. arXiv preprint arXiv:2104.02867.
Kim, B., Choi, T., Kang, J., and Kim, H. J. (2020a). Union-
det: Union-level detector towards real-time human-
object interaction detection. In European Conference
on Computer Vision, pages 498–514. Springer.
Kim, B., Lee, J., Kang, J., Kim, E.-S., and Kim, H. J.
(2021). Hotr: End-to-end human-object interac-
tion detection with transformers. arXiv preprint
arXiv:2104.13682.
Kim, D., Lee, G., Jeong, J., and Kwak, N. (2020b). Tell
me what they’re holding: Weakly-supervised object
detection with transferable knowledge from human-
object interaction. In AAAI, pages 11246–11253.
Kipf, T. N. and Welling, M. (2016). Semi-supervised clas-
sification with graph convolutional networks. arXiv
preprint arXiv:1609.02907.
Li, Y.-L., Liu, X., Wu, X., Li, Y., and Lu, C. (2020a). Hoi
analysis: Integrating and decomposing human-object
interaction. Advances in Neural Information Process-
ing Systems, 33.
Li, Y.-L., Xu, L., Liu, X., Huang, X., Xu, Y., Wang, S.,
Fang, H.-S., Ma, Z., Chen, M., and Lu, C. (2020b).
Pastanet: Toward human activity knowledge engine.
In Proceedings of the IEEE/CVF Conference on Com-
puter Vision and Pattern Recognition, pages 382–391.
Li, Y.-L., Zhou, S., Huang, X., Xu, L., Ma, Z., Fang, H.-
S., Wang, Y., and Lu, C. (2019). Transferable in-
teractiveness knowledge for human-object interaction
detection. In Proceedings of the IEEE Conference
on Computer Vision and Pattern Recognition, pages
3585–3594.
Liang, Z., Guan, Y., and Rojas, J. (2020). Visual-semantic
graph attention network for human-object interaction
detection. arXiv preprint arXiv:2001.02302.
Liao, Y., Liu, S., Wang, F., Chen, Y., Qian, C., and Feng,
J. (2020). Ppdm: Parallel point detection and match-
ing for real-time human-object interaction detection.
In Proceedings of the IEEE/CVF Conference on Com-
puter Vision and Pattern Recognition, pages 482–490.
Lin, T.-Y., Maire, M., Belongie, S., Hays, J., Perona, P.,
Ramanan, D., Doll
´
ar, P., and Zitnick, C. L. (2014).
Microsoft coco: Common objects in context. In Euro-
pean conference on computer vision, pages 740–755.
Springer.
Liu, Y., Yuan, J., and Chen, C. W. (2020). Consnet: Learn-
ing consistency graph for zero-shot human-object in-
teraction detection. In Proceedings of the 28th ACM
International Conference on Multimedia, pages 4235–
4243.
Maaten, L. v. d. and Hinton, G. (2008). Visualizing data
using t-sne. Journal of machine learning research,
9(Nov):2579–2605.
Nelissen, K., Luppino, G., Vanduffel, W., Rizzolatti, G.,
and Orban, G. A. (2005). Observing others: multi-
Human Object Interaction Detection Primed with Context
67