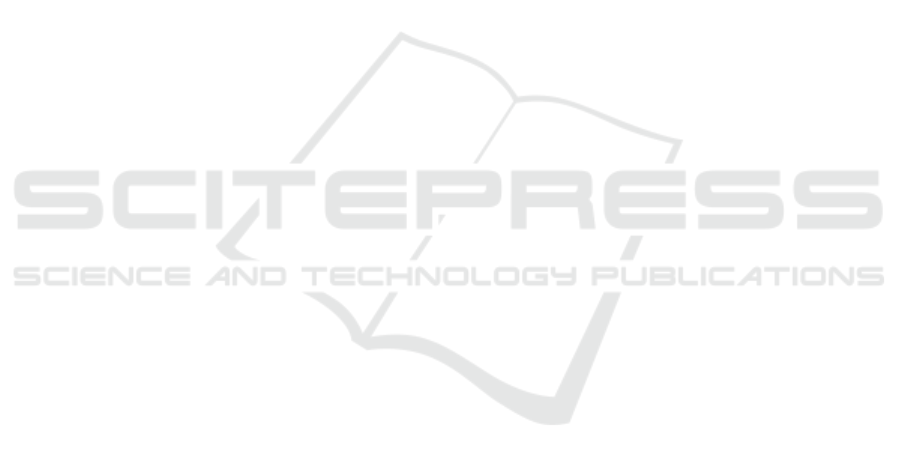
non-negative side. By intersecting the non-negative
half-space of h with n-dimensional space, a convex
feasible region is created which contains the solutions
for the conjunct (this holds because the intersection of
convex sets is a convex set). Applying this operation
for each conjunct in th CNF sentence results in a
convex feasible region which must contain solutions
to the CNF sentence, if any.
Our position is that Chop-SAT offers the possibil-
ity to find efficient and effective ways to solve SAT.
Chop-SAT produces a feasible region as follows:
• First, a knowledge base (KB) is defined as a CNF
sentence, and each conjunct is given a probability
(for a SAT problem, this will be 1 for each con-
junct).
• Next, the Chop-SAT method is used to produce
a set of hyperplanes such that the intersection of
their non-negative half-spaces determines the fea-
sible region.
• The resulting feasible region is convex.
The feasible region represents the solution space for
the KB. If any of the original hypercube corners exist
in the feasible region, the KB is satisfiable, otherwise
it is unsatisfiable.
2 BACKGROUND
Gomory (Gomory, 1958) introduced the cutting plane
method as an extension to Dantzig’s linear pro-
gramming approach to solving discrete variable ex-
tremum problems (Dantzig, 1957). Gomory provided
a method so that when the objective function was
maximized (e.g., using the simplex method), if the
result was a non-integral solution, then a new con-
straint plane was found algorithmically to separate
(cut) the non-integer solution from the integer solu-
tions. Chvatal (Chvatal, 1973; Chvatal, 1985) de-
veloped this method further, proved supporting the-
orems for bounded polytopes, and applied the results
to solve combinatorial problems. Note that integer
programming is in NP (Papadimitriou, 1981).
In terms of solving SAT, Cook et al. (Cook
et al., 1987) examined the complexity of cutting plane
proofs, and in particular, those for the unsatisfiability
of formulae in propositional calculus. This was done
in terms of resolution theorem proving using the cut-
ting planes method. Note that it is straightforward
to produce an equation from a disjunction; e.g., con-
sider (a ∨a) which results in the equation a + b ≥ 1.
Each conjunct gives rise to an equation, and the set
forms an integer programming problem where a and
b take on 0-1 values. Note that linear programming
relaxation can be used to allow the a and b to take
on values in the interval [0,1] – i.e., relaxing the 0-1
requirement. Hooker (Hooker, 1988) provided a way
to handle generalized resolution by observing that the
resolvent of two clauses corresponds to a certain cut-
ting plane in integer programming. Buss et al. (Buss
and Clote, 1996) describe an extension to Cook’s cut-
ting plane refutation approach that applies to thresh-
old logic analysis. For some recent work using cut-
ting planes, see Devriendt et al. (Devriendt et al.,
2021) who use the method to improve state-of-the-art
pseudo-Boolean optimization. All these methods are
still exponential time complexity.
3 THE Chop-SAT METHOD
We look at the SAT problem geometrically: for an n-
variable problem, the corners of the hypercube are the
only possible SAT solutions, and the interior points
are possible atom probabilities assignments. Given
a Conjunctive Normal Form (CNF) sentence, S =
C
1
∧C
2
∧. .. ∧C
m
, over a set of Boolean variables
A = {a
1
,a
2
,...,a
n
}, each conjunct, C
i
, has at least one
truth assignment that makes it false (note that a ∨¬a
is not allowed). If there are k
i
literals in conjunct C
i
,
then there are 2
k
truth assignments that make it false,
where k = n −k
i
. A hyperplane can then be found for
each conjunct which separates the solutions from the
non-solutions. Moreover, this hyperplane can be po-
sitioned so as to cut the edges between non-solution
corners and their feasible region neighbors anywhere
in the range 0 < x ≤ 1 along those edges. The in-
tersection of the non-negative side of the hyperplane
with the initial feasible region (H
n
or ℜ
n
) produces
a convex feasible region. Continuing this process for
each conjunct, the result is a convex feasible region
which may or may not contain a corner of H
n
– i.e., a
solution for the CNF sentence.
Suppose A = {a
1
,a
2
,...,a
n
} is a set of Boolean
variables, and the set of A -formulas is the inductive
set of propositional well-formed formulas over A. A
Conjunctive Normal Form (CNF) sentence over A is
defined as a conjunction of a set of disjunctions of
literals.
S = C
1
∧C
2
∧. .. ∧C
m
C
i
= L
i
1
∨L
i
2
∨. .. ∨L
i
k
i
L
i
j
= a
i
j
or L
i
j
= ¬a
i
j
The Satisfiability Problem is defined as (Davis
et al., 1994):
Find an efficient algorithm for testing an A-
formula in CNF to determine whether it is
truth-functional satisfiable.
Chop-SAT: A New Approach to Solving SAT and Probabilistic SAT for Agent Knowledge Bases
49