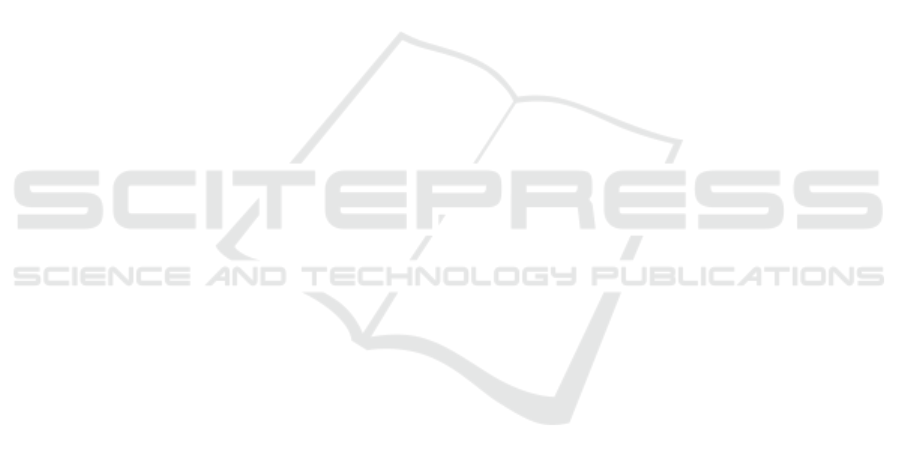
by the five-trials. Those trials were conducted by the
five-set of the training set and validation set, each con-
taining the no duplicated WSIs for each trials.
4.3 Implementation Details
In a comparison experiment with the single-scale
methods, we used the same training data style as
that of the DA-MIL network paper(Hashimoto et al.,
2020). Additionally, we applied the model structure
of the DA-MIL network in the same setting as that of
the DA-MIL network paper too. Therefore, we hired
the feature extractor composed of VGG16(Simonyan
and Zisserman, 2015) and two linear layers. Then,
we applied VGG16 obtained from trained DA-MIL
network as the feature extractors of the MSAA-Net,
DSMIL, and MS-DA-MIL network.
In a comparison experiment with the multi-scale
methods, we applied the model structure of the MS-
DA-MIL network in the same setting as that of the
MS-DA-MIL network paper(Hashimoto et al., 2020).
Then, except for the feature extractor of the DSMIL,
we used the original model structure to the DSMIL(Li
et al., 2021a).
In MSAA-Net, we used the same feature extrac-
tor F
(s
j
)
(·) structure as the DA-MIL. In addition, the
structure of the region aggregator for each scale and
the scale aggregator are the same. Those aggregators
are composed of the linear layer, Tanh activation, lin-
ear layer, and softmax function serially and calculate
the attention weights. Finally, we used the single lin-
ear layer as the classifier P(·).
We trained all model with the automatic mixed
precision, gradient accumulation, and Adam opti-
mizer. We set 16 to the mini-batch size substantially.
The number of training epochs is set to 50 and 100 for
the comparison experiment in the single-scale meth-
ods and multi-scale methods, respectively.
4.4 Results
Table 2 lists the classification results of the single-
scale method and the proposed method. The proposed
method performed equal or better in each metric than
that of the conventional method in both datasets. In
particular, the proposed method exhibited an 18.5%
higher F1 score than that of the DA-MIL network with
20x in the private-LUAD dataset. Thus, we confirmed
that the multi-scale WSIs could provide high cancer
detection ability.
Table 3 lists the averages and standard deviations
of metrics by the five-trials as the evaluation results
obtained by the proposed method and the conven-
tional methods with the multi-scale approach. The
results of the TCGA-LUAD dataset, the average num-
ber of misclassified WSIs, are 4.8, 5.6, and 5.2
at DSMIL, MS-DA-MIL, and MSAA, respectively.
That difference between the method is under one.
Therefore, although slight differences were observed,
all methods accurately classified the TCGA-LUAD
dataset.
In contrast, in the private-LUAD dataset, the F1
score of the proposed method was higher than that of
the conventional methods. In particular, the F1 score
of the proposed method was 10.7% higher than that
of the DSMIL. Furthermore, the MSAA-Net consid-
erably improved the recall performance, which was
20% higher than that of the DSMIL and 6.3% higher
than that of the MS-DA-MIL. The classification of
the WSIs in the private-LUAD dataset is more diffi-
cult than that of the TCGA-LUAD dataset because the
cancerous regions in the WSIs in the private-LUAD
dataset are small as shown in Figure 3. However, the
proposed method performed higher than the conven-
tional method.
According to these results, the proposed method
diagnosed with fewer overlooks than that of the
conventional methods. Consequently, the proposed
method achieves a high cancer diagnosis performance
because of the feature aggregation mechanism consid-
ering the multi-scale structures.
5 DISCUSSION
Figure 4 shows the WSIs contained in the test set of
the private-LUAD dataset and attention maps of the
attention weights for the corresponding regions. The
ground truth images show the WSIs corresponding
to the test data that all methods predicted as cancer.
In the images, the green regions enclosed by dotted
lines indicate the cancer regions diagnosed by pathol-
ogists. Moreover, the remaining images are the at-
tention maps produced by the DSMIL, MS-DA-MIL
network, and MSAA-Net. The attention maps imply
that the brighter the regions, the higher is the cancer
probability. Note that, because of the difference in
the feature aggregation mechanism of each method,
the DSMIL shows the attention map per region, and
the MS-DA-MIL network and the MSAA-Net show
the attention maps per region for each scale.
The attention maps are significantly different al-
though all methods predict correctly. The attention
maps of the DSMIL and MS-DA-MIL network at the
10x magnification show the cancer regions as the high
attention weights. The attention weights of the pro-
posed network were assigned to different regions de-
pending on the scales. In particular, the high values on
Multi-Scale Feature Aggregation Based Multiple Instance Learning for Pathological Image Classification
625