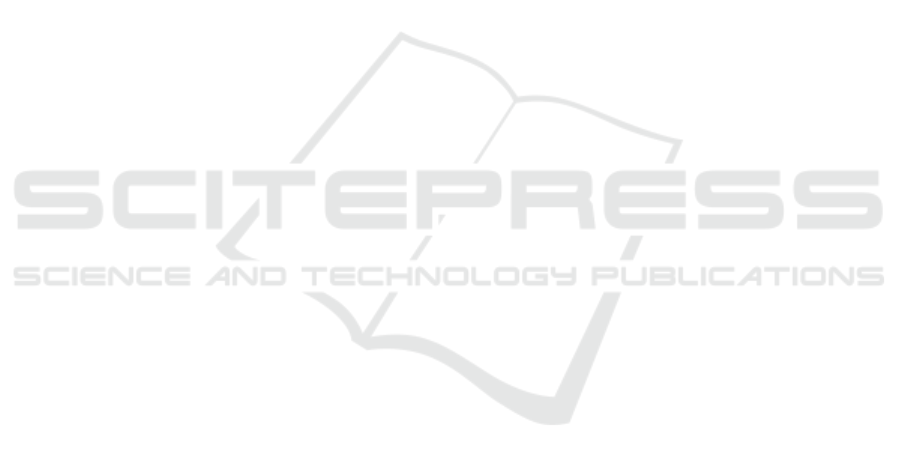
Ladicky, L., Torr, P. H., and Zisserman, A. (2013). Human
pose estimation using a joint pixel-wise and part-wise
formulation. In Conference on Computer Vision and
Pattern Recognition (CVPR). 3
Li, J., Raventos, A., Bhargava, A., Tagawa, T., and Gaidon,
A. (2018a). Learning to fuse things and stuff. arXiv
preprint arXiv:1812.01192. 3
Li, P., Xu, Y., Wei, Y., and Yang, Y. (2020a). Self-correction
for human parsing. Transactions on Pattern Analysis
and Machine Intelligence (T-PAMI). 3
Li, Q., Arnab, A., and Torr, P. H. (2017a). Holistic,
instance-level human parsing. British Machine Vision
Conference (BMVC). 1, 3
Li, Q., Arnab, A., and Torr, P. H. (2018b). Weakly-and
semi-supervised panoptic segmentation. In European
conference on computer vision (ECCV). 3
Li, Q., Qi, X., and Torr, P. H. (2020b). Unifying training and
inference for panoptic segmentation. In Conference on
Computer Vision and Pattern Recognition (CVPR). 1
Li, X., Xu, S., Yang, Y., Cheng, G., Tong, Y., and Tao,
D. (2022). Panoptic-PartFormer: Learning a Unified
Model for Panoptic Part Segmentation. In European
Conference on Computer Vision (ECCV). 1
Li, Y., Qi, H., Dai, J., Ji, X., and Wei, Y. (2017b). Fully con-
volutional instance-aware semantic segmentation. In
Conference on Computer Vision and Pattern Recogni-
tion (CVPR). 3
Liang, X., Gong, K., Shen, X., and Lin, L. (2018). Look
into person: Joint body parsing & pose estimation net-
work and a new benchmark. Transactions on Pattern
Analysis and Machine Intelligence (T-PAMI). 3
Lin, K., Wang, L., Luo, K., Chen, Y., Liu, Z., and Sun,
M.-T. (2020). Cross-domain complementary learning
using pose for multi-person part segmentation. Trans-
actions on Circuits and Systems for Video Technology
(T-CSVT). 3
Liu, H., Peng, C., Yu, C., Wang, J., Liu, X., Yu, G., and
Jiang, W. (2019). An end-to-end network for panoptic
segmentation. In Conference on Computer Vision and
Pattern Recognition (CVPR). 3
Liu, S., Sun, Y., Zhu, D., Ren, G., Chen, Y., Feng, J., and
Han, J. (2018). Cross-domain human parsing via ad-
versarial feature and label adaptation. In Conference
On Artificial Intelligence (AAAI). 1, 3
Luo, P., Wang, X., and Tang, X. (2013). Pedestrian parsing
via deep decompositional network. In International
Conference on Computer Vision (ICCV). 1
Luo, X., Su, Z., Guo, J., Zhang, G., and He, X. (2018).
Trusted guidance pyramid network for human pars-
ing. In ACM International Conference on Multimedia
(ACM-MM). 3
Meletis, P., Wen, X., Lu, C., de Geus, D., and Dubbelman,
G. (2020). Cityscapes-panoptic-parts and pascal-
panoptic-parts datasets for scene understanding. arXiv
preprint arXiv:2004.07944. 2, 3, 5, 6, 7, 8, 11, 12
Michieli, U., Borsato, E., Rossi, L., and Zanuttigh, P.
(2020). Gmnet: Graph matching network for large
scale part semantic segmentation in the wild. In Eu-
ropean Conference on Computer Vision (ECCV). 1, 3,
6
Mohan, R. and Valada, A. (2021). EfficientPS: Effi-
cient Panoptic Segmentation. International Journal
of Computer Vision (IJCV). 1, 3, 4, 5, 6
O Pinheiro, P. O., Collobert, R., and Doll
´
ar, P. (2015).
Learning to segment object candidates. Advances in
Neural Information Processing Systems (NeurIPS). 3
Pont-Tuset, J., Arbelaez, P., Barron, J. T., Marques, F., and
Malik, J. (2016). Multiscale combinatorial grouping
for image segmentation and object proposal genera-
tion. Transactions on Pattern Analysis and Machine
Intelligence (T-PAMI). 2
Porzi, L., Bulo, S. R., Colovic, A., and Kontschieder, P.
(2019). Seamless scene segmentation. In Conference
on Computer Vision and Pattern Recognition (CVPR).
1, 3
Qiao, S., Chen, L.-C., and Yuille, A. (2020). Detec-
tors: Detecting objects with recursive feature pyra-
mid and switchable atrous convolution. arXiv preprint
arXiv:2006.02334. 6
Ren, S., He, K., Girshick, R. B., and Sun, J. (2015). Faster
R-CNN: towards real-time object detection with re-
gion proposal networks. Advances in Neural Informa-
tion Processing Systems (NeurIPS). 3
Ruan, T., Liu, T., Huang, Z., Wei, Y., Wei, S., and Zhao, Y.
(2019). Devil in the details: Towards accurate single
and multiple human parsing. In Conference on Artifi-
cial Intelligence (AAAI). 3
Sofiiuk, K., Barinova, O., and Konushin, A. (2019). Adap-
tis: Adaptive instance selection network. In Interna-
tional Conference on Computer Vision (ICCV). 3
Tan, M. and Le, Q. (2019). Efficientnet: Rethinking model
scaling for convolutional neural networks. In Interna-
tional Conference on Machine Learning (ICML). 3
Tian, Z., He, T., Shen, C., and Yan, Y. (2019). Decoders
matter for semantic segmentation: Data-dependent
decoding enables flexible feature aggregation. In Con-
ference on Computer Vision and Pattern Recognition
(CVPR). 2
Valada, A., Mohan, R., and Burgard, W. (2018). Self-
supervised model adaptation for multimodal seman-
tic segmentation. International Journal of Computer
Vision (IJCV). 2
Xiong, Y., Liao, R., Zhao, H., Hu, R., Bai, M., Yumer, E.,
and Urtasun, R. (2019). Upsnet: A unified panoptic
segmentation network. In Conference on Computer
Vision and Pattern Recognition (CVPR). 1, 3
Yang, L., Song, Q., Wang, Z., and Jiang, M. (2019a). Pars-
ing r-cnn for instance-level human analysis. In Con-
ference on Computer Vision and Pattern Recognition
(CVPR). 3
Yang, T., Collins, M. D., Zhu, Y., Hwang, J., Liu, T., Zhang,
X., Sze, V., Papandreou, G., and Chen, L. (2019b).
Deeperlab: Single-shot image parser. arXiv preprint
arXiv:1902.05093. 3
Zhang, H., Wu, C., Zhang, Z., Zhu, Y., Lin, H., Zhang,
Z., Sun, Y., He, T., Mueller, J., Manmatha, R., et al.
(2022). Resnest: Split-attention networks. In Con-
ference on Computer Vision and Pattern Recognition
(CVPR). 6
ICPRAM 2023 - 12th International Conference on Pattern Recognition Applications and Methods
24