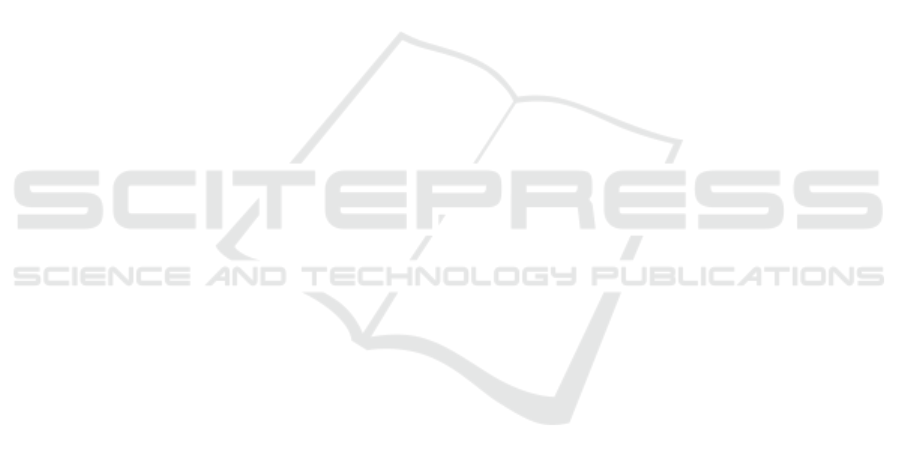
time based on the knowledge of seen source datasets.
CoDA-Few was shown to be a useful Domain
Adaptation method that could learn a single model
that performs satisfactory predictions for several dif-
ferent unseen target datasets in a domain, even when
the visual patterns of these data were different. The
proposed method was able to gather both labeled and
unlabeled data in the inference process, making it
highly adaptable to a wide variety of data scarcity sce-
narios.
CoDA-Few reached results in Few-Shot DA that
are comparable to DA methods that do have access to
the target data distribution. Furthermore, it presented
better Jaccard values in most experiments where la-
beled data was scarce, such as in heart segmentation
where only JSRT provided labeled training data. The
method also presented good performance in Few-Shot
DA tasks, even for highly imbalanced classes, such as
in the case of heart segmentation, wherein the region
of interest in images represented only a very small
slice of the number of pixels.
One should notice that CoDA-Few is conceptually
not limited to 2D dense labeling tasks or biomedical
images, despite being tested only for non-volumetric
segmentation tasks in this paper. Future works will
investigate Few-Shot DA in the segmentation of vol-
umetric images, such as Computed Tomography (CT)
scans, Positron Emission Tomography (PET scans),
and Magnetic Resonance Imaging (MRI). We also
plan to test CoDA-Few in other image domains, such
as traditional Computer Vision datasets and Remote
Sensing data.
ACKNOWLEDGEMENTS
The authors would like to thank CAPES, CNPq
(424700/2018-2 and 306955/2021-0), FAPEMIG
(APQ-00449-17 and APQ-00519-20), FAPESP (grant
#2020/06744-5), and Serrapilheira Institute (grant
#R-2011-37776) for their financial support to this re-
search project.
REFERENCES
Bustos, A., Pertusa, A., Salinas, J.-M., and de la Iglesia-
Vay
´
a, M. (2020). Padchest: A large chest x-ray image
dataset with multi-label annotated reports. Medical
Image Analysis, 66:101797.
Demner-Fushman, D., Kohli, M. D., Rosenman, M. B.,
Shooshan, S. E., Rodriguez, L., Antani, S., Thoma,
G. R., and McDonald, C. J. (2016). Preparing a col-
lection of radiology examinations for distribution and
retrieval. Journal of the American Medical Informat-
ics Association, 23(2):304–310.
Goodfellow, I. J., Pouget-Abadie, J., Mirza, M., Xu, B.,
Warde-Farley, D., Ozair, S., Courville, A. C., and Ben-
gio, Y. (2014). Generative adversarial nets. In NIPS.
Hoffman, J., Tzeng, E., Park, T., Zhu, J.-Y., Isola, P.,
Saenko, K., Efros, A., and Darrell, T. (2018). Cy-
cada: Cycle-consistent adversarial domain adaptation.
In ICML, pages 1989–1998. PMLR.
Huang, X., Liu, M.-Y., Belongie, S., and Kautz, J. (2018).
Multimodal unsupervised image-to-image translation.
In Proceedings of the European conference on com-
puter vision (ECCV), pages 172–189.
Isola, P., Zhu, J.-Y., Zhou, T., and Efros, A. A. (2017).
Image-to-image translation with conditional adversar-
ial networks. In Proceedings of the IEEE conference
on computer vision and pattern recognition, pages
1125–1134.
Jaeger, S., Candemir, S., Antani, S., W
´
ang, Y.-X. J., Lu,
P.-X., and Thoma, G. (2014). Two public chest
x-ray datasets for computer-aided screening of pul-
monary diseases. Quantitative Imaging in Medicine
and Surgery, 4(6):475.
Karras, T., Aila, T., Laine, S., and Lehtinen, J. (2017). Pro-
gressive growing of gans for improved quality, stabil-
ity, and variation. arXiv preprint arXiv:1710.10196.
Krizhevsky, A., Sutskever, I., and Hinton, G. E. (2012). Im-
ageNet classification with deep convolutional neural
networks. NIPS, 25:1097–1105.
Liu, M.-Y., Breuel, T., and Kautz, J. (2017). Unsupervised
image-to-image translation networks. In Proceedings
of the 31st International Conference on Neural Infor-
mation Processing Systems, pages 700–708.
Liu, M.-Y., Huang, X., Mallya, A., Karras, T., Aila, T.,
Lehtinen, J., and Kautz, J. (2019). Few-shot unsu-
pervised image-to-image translation. In Proceedings
of the IEEE/CVF International Conference on Com-
puter Vision, pages 10551–10560.
Murez, Z., Kolouri, S., Kriegman, D., Ramamoorthi, R.,
and Kim, K. (2018). Image to image translation for
domain adaptation. In Proceedings of the IEEE Con-
ference on Computer Vision and Pattern Recognition,
pages 4500–4509.
Oliveira, H. N., Ferreira, E., and Dos Santos, J. A.
(2020). Truly generalizable radiograph segmentation
with conditional domain adaptation. IEEE Access,
8:84037–84062.
Ronneberger, O., Fischer, P., and Brox, T. (2015). U-net:
Convolutional networks for biomedical image seg-
mentation. In International Conference on Medical
image computing and computer-assisted intervention,
pages 234–241. Springer.
Salimans, T., Goodfellow, I., Zaremba, W., Cheung, V.,
Radford, A., and Chen, X. (2016). Improved tech-
niques for training gans. Advances in neural informa-
tion processing systems, 29:2234–2242.
Shiraishi, J., Katsuragawa, S., Ikezoe, J., Matsumoto, T.,
Kobayashi, T., Komatsu, K.-i., Matsui, M., Fujita,
H., Kodera, Y., and Doi, K. (2000). Development
of a digital image database for chest radiographs with
CoDA-Few: Few Shot Domain Adaptation for Medical Image Semantic Segmentation
725