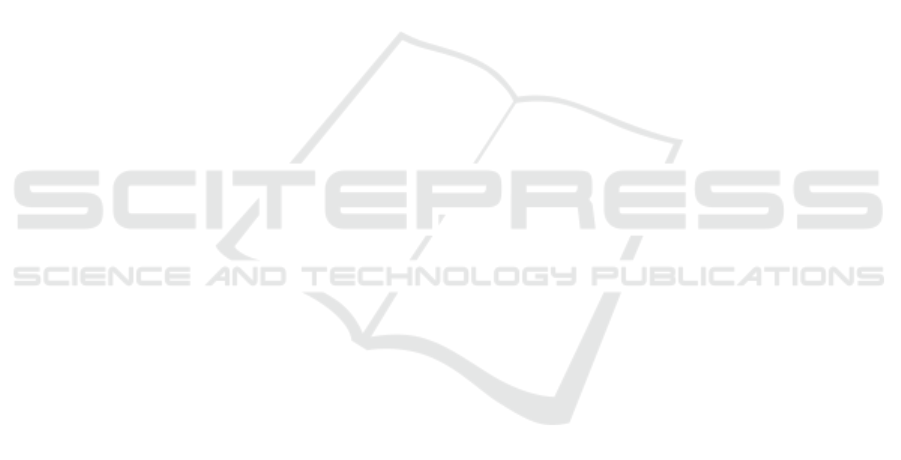
Kacem, A., Hammal, Z., Daoudi, M., and Cohn, J. (2018).
Detecting depression severity by interpretable repre-
sentations of motion dynamics. In 13th IEEE Inter-
national Conference on Automatic Face and Gesture
Recognition, FG 2018, pages 739–745.
Kapur, A., Kapur, A., Virji-Babul, N., Tzanetakis, G., and
Driessen, P. (2005). Gesture-based affective comput-
ing on motion capture data. In International Confer-
ence on Affective Computing and Intelligent Interac-
tion, ACII, volume 3784, pages 1–7.
Kico, I. and Liarokapis, F. (2020). Investigating the learn-
ing process of folk dances using mobile augmented
reality. Applied Sciences, 10(2):599.
Kora, T., Soga, M., and Taki, H. (2015). Golf learning envi-
ronment enabling overlaid display of expert’s model
motion and learner’s motion using kinect. Proce-
dia Computer Science, 60:1559–1565. Knowledge-
Based and Intelligent Information & Engineering Sys-
tems 19th Annual Conference, KES-2015, Singapore,
September 2015 Proceedings.
Larboulette, C. and Gibet, S. (2015). A review of com-
putable expressive descriptors of human motion. In
Proceedings of the 2nd International Workshop on
Movement and Computing, MOCO ’15, page 21–28.
Association for Computing Machinery.
Le Naour, T., Hamon, L., and Bresciani, J.-P. (2019). Super-
imposing 3d virtual self + expert modeling for motor
learning: Application to the throw in american foot-
ball. Frontiers in ICT, 6:16.
L
´
ecuyer, F., Gouranton, V., Reuzeau, A., Gaugne, R., and
Arnaldi, B. (2020). Action sequencing in VR, a no-
code approach. LNCS Transactions on Computational
Science, pages 57–76.
Liu, J., Zheng, Y., Wang, K., Bian, Y., Gai, W., and Gao, D.
(2020). A real-time interactive tai chi learning system
based on vr and motion capture technology. Procedia
Computer Science, 174:712–719. 2019 International
Conference on Identification, Information and Knowl-
edge in the Internet of Things.
Moore, A. G., McMahan, R. P., Dong, H., and Ruozzi, N.
(2020). Extracting velocity-based user-tracking fea-
tures to predict learning gains in a virtual reality train-
ing application. In 2020 IEEE International Sym-
posium on Mixed and Augmented Reality (ISMAR),
pages 694–703.
Morel, M. (2017). Multidimensional time-series averag-
ing: Application to automatic and generic evaluation
of sport gestures. Theses, Universit
´
e Pierre et Marie
Curie - Paris VI.
Morel, M., Achard, C., Kulpa, R., and Dubuisson, S.
(2017). Automatic evaluation of sports motion: A
generic computation of spatial and temporal errors.
Image and Vision Computing, 64:67–78.
Naser, M. and Alavi, A. (2020). Insights into performance
fitness and error metrics for machine learning. Re-
search Gate.
Park, D.-S., Lee, D.-G., Lee, K., and Lee, G. (2017). Ef-
fects of virtual reality training using xbox kinect on
motor function in stroke survivors: A preliminary
study. Journal of Stroke and Cerebrovascular Dis-
eases, 26(10):2313–2319.
Pilati, F., Faccio, M., Gamberi, M., and Regattieri, A.
(2020). Learning manual assembly through real-time
motion capture for operator training with augmented
reality. Procedia Manufacturing, 45:189–195. Learn-
ing Factories across the value chain – from innovation
to service – The 10th Conference on Learning Facto-
ries 2020.
Rold
´
an, J. J., Crespo, E., Mart
´
ın-Barrio, A., Pe
˜
na-Tapia,
E., and Barrientos, A. (2019). A training system for
industry 4.0 operators in complex assemblies based
on virtual reality and process mining. Robotics and
computer-integrated manufacturing, 59:305–316.
Savitzky, A. and Golay, M. (1964). Smoothing and differen-
tiation of data by simplified least squares procedures.
Analytical Chemistry, 36:1627–1639.
Schafer, R. W. (2011). What is a savitzky-golay filter?
IEEE Signal Processing Magazine, 28:111 – 117.
Sror, L., Vered, M., Treger, I., Levy-Tzedek, S., Levin,
M. F., and Berman, S. (2019). A virtual reality-based
training system for error-augmented treatment in pa-
tients with stroke. In 2019 International Conference
on Virtual Rehabilitation (ICVR), pages 1–2.
Swee, S. K., You, L. Z., Hang, B. W. W., and Kiang, D. K. T.
(2017). Development of rehabilitation system using
virtual reality. In 2017 International Conference on
Robotics, Automation and Sciences (ICORAS), pages
1–6.
Thomas, S., G.N., P., and Kirat, P. (2017). Prediction of
peak ground acceleration using e-svr, v-svr and ls-
svr algorithm. Geomatics Natural Hazards and Risk,
8:177–193.
Vabalas, A., Gowen, E., Poliakoff, E., and Casson, A. J.
(2020). Applying machine learning to kinematic and
eye movement features of a movement imitation task
to predict autism diagnosis. Scientific Reports.
Wei, W., Lu, Y., Printz, C. D., and Dey, S. (2015). Motion
data alignment and real-time guidance in cloud-based
virtual training system. In Proceedings of the Con-
ference on Wireless Health, WH ’15, New York, NY,
USA. Association for Computing Machinery.
Winkler-Schwartz, A., Yilmaz, R., Mirchi, N., Bissonnette,
V., Ledwos, N., Siyar, S., Azarnoush, H., Karlik, B.,
and Del Maestro, R. (2019). Machine Learning Identi-
fication of Surgical and Operative Factors Associated
With Surgical Expertise in Virtual Reality Simulation.
JAMA Network Open, 2(8):e198363–e198363.
Automatic Prediction of 3D Checkpoints for Technical Gesture Learning in Virtual Environments
51