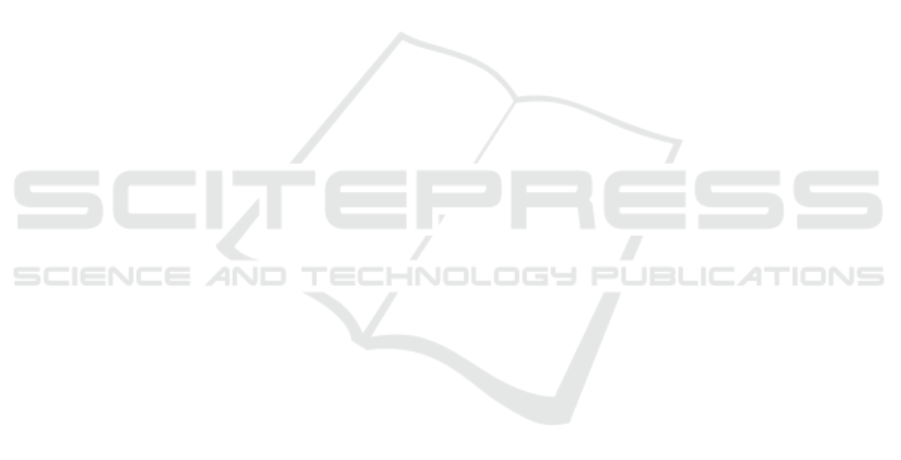
Chen, S., Webb, G. I., Liu, L., and Ma, X. (2020). A novel
selective na
¨
ıve bayes algorithm. Knowledge-Based
Systems, 192:106361.
Christiani, N. and Shawe-Taylor, J. (2000). An introduc-
tion to support vector machines. Cambridge Univer-
sity Press.
Dietterich, T. G. (1998). Approximate statistical tests
for comparing supervised classification learning algo-
rithms. Neural computation, 10(7):1895–1923.
Dixon, J., Moran, L., Rodriguez, J., and Domke, R. (2005).
Reactive power compensation technologies: State-of-
the-art review. Proceedings of the IEEE, 93(12):2144–
2164.
Feurer, M. and Hutter, F. (2019). Hyperparameter optimiza-
tion. In Automated machine learning, pages 3–33.
Springer, Cham.
Freund, Y. and Schapire, R. E. (1997). A decision-theoretic
generalization of on-line learning and an application
to boosting. Journal of computer and system sciences,
55(1):119–139.
Friedman, J. (2001). Greedy function approximation: A
gradient boosting machine. The Annals of Statistics,
29(5).
Glorot, X. and Bengio, Y. (2010). Understanding the dif-
ficulty of training deep feedforward neural networks.
In International Conference on Artificial Intelligence
and Statistics.
Gou, J., Ma, H., Ou, W., Zeng, S., Rao, Y., and Yang, H.
(2019). A generalized mean distance-based k-nearest
neighbor classifier. Expert Systems with Applications,
115:356–372.
Jiang, L., Cai, Z., Wang, D., and Jiang, S. (2007). Survey
of improving k-nearest-neighbor for classification. In
Fourth international conference on fuzzy systems and
knowledge discovery (FSKD 2007), pages 679–683.
Kleinbaum, D. G., Dietz, K., Gail, M., Klein, M., and Klein,
M. (2002). Logistic regression. New York: Springer-
Verlag.
Li, H., Wang, Z., and Hong, T. (2021a). A synthetic build-
ing operation dataset. Scientific data, 8(1):1–13.
Li, L., Meinrenken, C. J., Modi, V., and Culligan, P. J.
(2021b). Impacts of covid-19 related stay-at-home
restrictions on residential electricity use and implica-
tions for future grid stability. Energy and Buildings,
251:111330.
Maitre, J. and Glon, G. (2015). Efficient appliances recog-
nition in smart homes based on active and reactive
power, fast fourier transform and decision trees. In
Workshops at the Twenty-Ninth AAAI Conference on
Artificial Intelligence. SCITEPRESS.
Mantovani, R. G., Horv
´
ath, T., Cerri, R., Vanschoren, J.,
and de Carvalho, A. C. (2016). Hyper-parameter
tuning of a decision tree induction algorithm. In
2016 5th Brazilian Conference on Intelligent Systems
(BRACIS), pages 37–42. IEEE.
Mbinkar, E. N., Asoh, D. A., and Kujabi, S. (2022). Mi-
crocontroller control of reactive power compensation
for growing industrial loads. Energy and Power Engi-
neering, 14(9):460–476.
Meinrenken, C. J., Abrol, S., Gite, G. B., Hidey, C.,
McKeown, K., Mehmani, A., Modi, V., Turcan, E.,
Xie, W., and Culligan, P. J. (2021). Residential
electricity conservation in response to auto-generated,
multi-featured, personalized eco-feedback designed
for large scale applications with utilities. Energy and
Buildings, 232:110652.
Meinrenken, C. J., Rauschkolb, N., Abrol, S., Chakrabarty,
T., Decalf, V. C., Hidey, C., McKeown, K., Mehmani,
A., Modi, V., and Culligan, P. J. (2020). Mfred, 10
second interval real and reactive power for groups of
390 us apartments of varying size and vintage. Scien-
tific Data, 7:375.
Mukherjee, S., Ganguly, A., Paul, A. K., and Datta, A. K.
(2018). Load flow analysis and reactive power com-
pensation. In 2018 International Conference on
Computing, Power and Communication Technologies
(GUCON), pages 207–211. IEEE.
Raschka, S. (2018). Mlxtend: Providing machine learning
and data science utilities and extensions to python’s
scientific computing stack. The Journal of Open
Source Software, 3(24).
Rish, I. (2001). An empirical study of the naive bayes clas-
sifier. IJCAI 2001 workshop on empirical methods in
artificial intelligence, 3(22):41–46.
Sarkar, M. N. I., Meegahapola, L. G., and Datta, M. (2018).
Reactive power management in renewable rich power
grids: A review of grid-codes, renewable generators,
support devices, control strategies and optimization
algorithms. IEEE Access, 6:41458–41489.
T
´
ellez, A. A., L
´
opez, G., Isaac, I., and Gonz
´
alez, J. W.
(2018). Optimal reactive power compensation in elec-
trical distribution systems with distributed resources.
review. Heliyon, 4(8):e00746.
Vishnu, M. and Kumar, S. (2020). An improved solution
for reactive power dispatch problem using diversity-
enhanced particle swarm optimization. Energies,
13(11):2862.
Wu, J., Chen, X. Y., Zhang, H., Xiong, L. D., Lei, H., and
Deng, S. H. (2019). Hyperparameter optimization for
machine learning models based on bayesian optimiza-
tion. Journal of Electronic Science and Technology,
17(1):26–40.
Yang, L. and Shami, A. (2020). On hyperparameter opti-
mization of machine learning algorithms: Theory and
practice. Neurocomputing, 415:295–316.
Yoshida, K., Rijal, H. B., Bogaki, K., Mikami, A., and
Abe, H. (2021). Field study on energy-saving be-
haviour and patterns of air-conditioning use in a con-
dominium. Energies, 14(24):8572.
Zhao, Y., Irving, M. R., and Song, Y. (2005). A cost alloca-
tion and pricing method for reactive power service in
the new deregulated electricity market environment.
In 2005 IEEE/PES Transmission & Distribution Con-
ference & Exposition: Asia and Pacific, pages 1–6.
IEEE.
Zhou, X., Wei, K., Ma, Y., and Gao, Z. (2018). A re-
view of reactive power compensation devices. In 2018
IEEE International Conference on Mechatronics and
Automation (ICMA), pages 2020–2024. IEEE.
Automatic Reactive Power Classification Based on Selected Machine Learning Methods
107