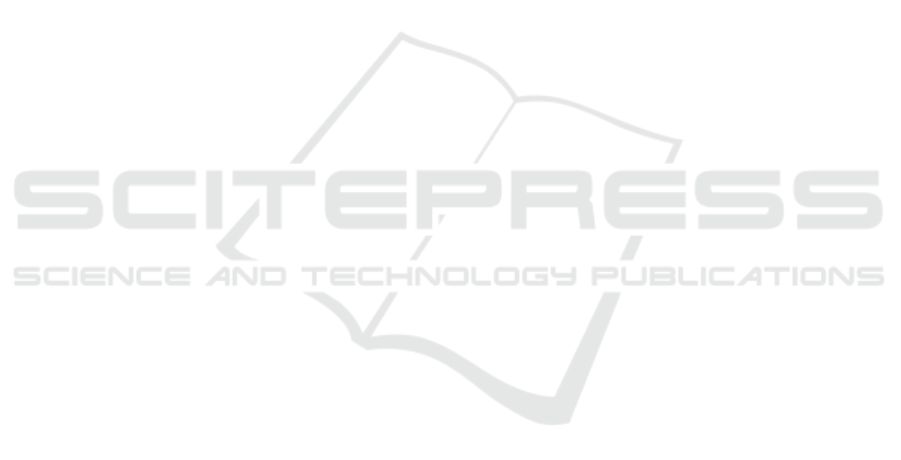
Goldblum, M., Tsipras, D., Xie, C., and Chen, X. (2020).
Dataset Security for Machine Learning: Data Poison-
ing, Backdoor Attacks, and Defenses. IEEE Transac-
tions on Pattern Analysis & Machine Intelligence.
Guidotti, R., Monreale, A., Ruggieri, S., Turini, F., Gian-
notti, F., and Pedreschi, D. (2018). A Survey Of Meth-
ods for Explaining Black Box Models. ACM Comput-
ing Surveys, 51:1–42.
He, K., Zhang, X., Ren, S., and Sun, J. (2016). Deep Resid-
ual Learning for Image Recognition. In Conference on
Computer Vision and Pattern Recognition, Las Vegas,
USA.
Hendrycks, D. and Dietterich, T. (2019). Benchmarking
Neural Network Robustness to Common Corruptions
and Perturbations. In International Conference on
Learning Representations, New Orleans, USA.
ISO 21448 (2022). Road vehicles - Safety of the intended
funtionality. Standard, International Organization for
Standardization.
ISO 26262 (2018). Road vehicles - Functional safety. Stan-
dard, International Organization for Standardization.
ISO/AWI PAS 8800 (2022). Road vehicles - Safety and
artificial intelligence. Standard, International Organi-
zation for Standardization.
ISO/AWI TS 5083 (2022). Road vehicles - Safety for auto-
mated driving systems - Design, verification and vali-
dation. Standard, International Organization for Stan-
dardization.
ISO/IEC DTR 5469 (2022). Artificial intelligence - Func-
tional safety and AI systems. Standard, International
Organization for Standardization, International Elec-
trotechnical Commission.
ISO/IEC PRF TS 4213 (2022). Artificial intelligence -
Assessment of machine learning classification perfor-
mance. Standard, International Organization for Stan-
dardization, International Electrotechnical Commis-
sion.
ISO/IEC TR 24028 (2020). Artificial intelligence -
Overview of trustworthiness in artificial intelligence.
Standard, International Organization for Standardiza-
tion, International Electrotechnical Commission.
ISO/IEC TR 24029-1 (2021). Artificial intelligence - As-
sessment of the robustness of neural networks - Part
1: Overview. Standard, International Organization for
Standardization, International Electrotechnical Com-
mission.
ISO/SAE 21434 (2021). Road vehicles - Cybersecurity en-
gineering. Standard, International Organization for
Standardization, SAE International.
Karpathy, A. (2021). Workshop on Autonomous Driving
- Tesla Keynote. In Conference on Computer Vision
and Pattern Recognition, Nashville, USA.
Li, B., Qi, P., Liu, B., Di, S., Liu, J., Pei, J., Yi, J., and
Zhou, B. (2022). Trustworthy AI: From Principles to
Practices. ACM Computing Surveys.
Lim, K., Hong, Y., Choi, Y., and Byun, H. (2017). Real-time
Traffic Sign Recognition based on a General Purpose
GPU and Deep Learning. PLOS ONE, 12:1–22.
Madry, A., Makelov, A., Schmidt, L., Tsipras, D., and
Vladu, A. (2018). Towards Deep Learning Models
Resistant to Adversarial Attacks. In International
Conference on Learning Representations, Vancouver,
Canada.
Mohseni, S., Pitale, M., Singh, V., and Wang, Z. (2020).
Practical Solutions for Machine Learning Safety in
Autonomous Vehicles. In Conference on Artificial In-
telligence: Workshop on Safe AI, New York, USA.
Orekondy, T., Schiele, B., and Fritz, M. (2019). Knockoff
Nets: Stealing Functionality of Black-Box Models. In
Conference on Computer Vision and Pattern Recogni-
tion, Long Beach, USA.
Papernot, N., McDaniel, P., Goodfellow, I., Jha, S., Celik,
B., and Swami, A. (2017). Practical Black-Box At-
tacks against Machine Learning. In ACM Asia Con-
ference on Computer and Communications Security,
Abu Dhabi, United Arab Emirates.
Radlak, K., Szczepankiewicz, M., Jones, T., and Serwa,
P. (2020). Organization of Machine Learning based
Product Development as per ISO 26262 and ISO/PAS
21448. In Pacific Rim International Symposium on
Dependable Computing, Perth, Australia.
Raji, I. D., Smart, A., White, R., Mitchell, M., Gebru,
T., Hutchinson, B., Smith-Loud, J., Theron, D., and
Barnes, P. (2020). Closing the AI Accountability Gap:
Defining an End-to-End Framework for Internal Al-
gorithmic Auditing. In ACM Conference on Fairness,
Accountability, and Transparency, Barcelona, Spain.
SAE J3016 (2014). Levels of Driving Automation. Stan-
dard, SAE International.
Schwarzschild, A., Goldblum, M., Gupta, A., Dickerson,
J., and Goldstein, T. (2021). Just How Toxic is Data
Poisoning? A Unified Benchmark for Backdoor and
Data Poisoning Attacks. In International Conference
on Machine Learning, Vienna, Austria.
Selvaraju, R., Cogswell, M., Das, A., Vedantam, R., Parikh,
D., and Batra, D. (2017). Grad-CAM: Visual Expla-
nations from Deep Networks via Gradient-based Lo-
calization. In International Conference on Computer
Vision, Venice, Italy.
Stallkamp, J., Schlipsing, M., Salmen, J., and Igel, C.
(2011). Man vs. Computer: Benchmarking Machine
Learning Algorithms for Traffic Sign Recognition. In
International Joint Conference on Neural Networks,
San Jose, USA.
Szegedy, C., Zaremba, W., Sutskever, I., Bruna, J., Erhan,
D., Goodfellow, I., and Fergus, R. (2014). Intriguing
Properties of Neural Networks. In International Con-
ference on Learning Representations, Banff, Canada.
UNECE R 155 (2021). Uniform provisions concerning the
approval of vehicles with regards to cyber security and
cyber security management system. Standard, United
Nations Economic Commission for Europe.
Waymo (2021). How we’ve built the World’s Most Experi-
enced Urban Driver. Waypoint.
Yurtsever, E., Lambert, J., Carballo, A., and Takeda, K.
(2020). A Survey of Autonomous Driving: Common
Practices and Emerging Technologies. IEEE Access,
8:58443–58469.
Ziebinski, A., Cupek, R., Erdogan, H., and Waechter, S.
(2016). A Survey of ADAS Technologies for the Fu-
ture Perspective of Sensor Fusion. In International
Conference on Computational Collective Intelligence,
Halkidiki, Greece.
ICISSP 2023 - 9th International Conference on Information Systems Security and Privacy
348