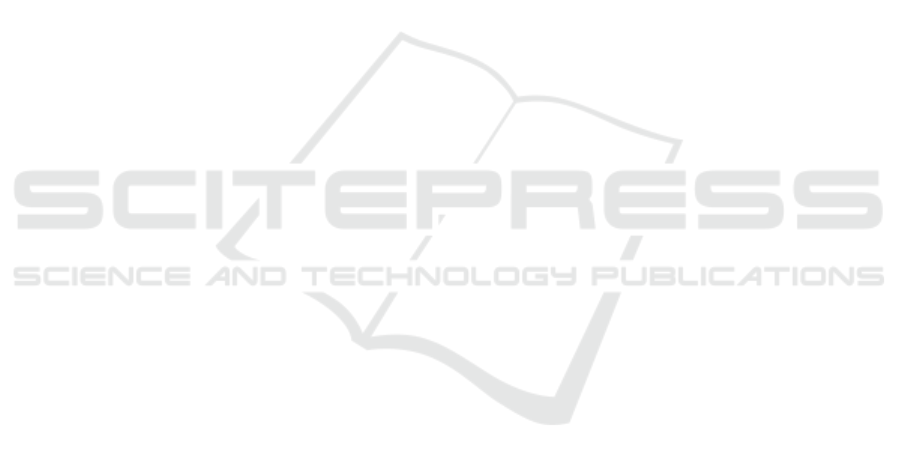
performance.
In summary, after introducing a theoretical frame-
work for studying predictive explanations in RL, we
presented a novel practical model-agnostic predictive-
explanation method.
REFERENCES
Amir, D. and Amir, O. (2018). HIGHLIGHTS: summariz-
ing agent behavior to people. In Andr
´
e, E., Koenig,
S., Dastani, M., and Sukthankar, G., editors, Pro-
ceedings of the 17th International Conference on Au-
tonomous Agents and MultiAgent Systems, AAMAS,
pages 1168–1176. International Foundation for Au-
tonomous Agents and Multiagent Systems / ACM.
Bastani, O., Pu, Y., and Solar-Lezama, A. (2018). Verifiable
reinforcement learning via policy extraction. In Ben-
gio, S., Wallach, H. M., Larochelle, H., Grauman, K.,
Cesa-Bianchi, N., and Garnett, R., editors, NeurIPS,
pages 2499–2509.
Brockman, G., Cheung, V., Pettersson, L., Schneider, J.,
Schulman, J., Tang, J., and Zaremba, W. (2016). Ope-
nai gym. arXiv preprint arXiv:1606.01540.
Cruz, F., Dazeley, R., and Vamplew, P. (2019). Memory-
based explainable reinforcement learning. In Liu, J.
and Bailey, J., editors, AI 2019: Advances in Arti-
ficial Intelligence - 32nd Australasian Joint Confer-
ence, volume 11919 of Lecture Notes in Computer
Science, pages 66–77. Springer.
Darwiche, A. (2018). Human-level intelligence or animal-
like abilities? Commun. ACM, 61(10):56–67.
Downey, R. G. and Fellows, M. R. (1995). Fixed-parameter
tractability and completeness II: on completeness for
W[1]. Theor. Comput. Sci., 141(1&2):109–131.
European Commission (2021). Artificial Intelligence Act.
Greydanus, S., Koul, A., Dodge, J., and Fern, A. (2018). Vi-
sualizing and understanding Atari agents. In Dy, J. G.
and Krause, A., editors, ICML, volume 80 of Pro-
ceedings of Machine Learning Research, pages 1787–
1796. PMLR.
Guo, W., Wu, X., Khan, U., and Xing, X. (2021). EDGE:
explaining deep reinforcement learning policies. In
Ranzato, M., Beygelzimer, A., Dauphin, Y. N., Liang,
P., and Vaughan, J. W., editors, NeurIPS, pages
12222–12236.
Hasselt, H. (2010). Double q-learning. Advances in neural
information processing systems, 23.
Iyer, R., Li, Y., Li, H., Lewis, M., Sundar, R., and Sycara,
K. P. (2018). Transparency and explanation in deep re-
inforcement learning neural networks. In Furman, J.,
Marchant, G. E., Price, H., and Rossi, F., editors, Pro-
ceedings of the 2018 AAAI/ACM Conference on AI,
Ethics, and Society, AIES, pages 144–150. ACM.
Juozapaitis, Z., Koul, A., Fern, A., Erwig, M., and Doshi-
Velez, F. (2019). Explainable reinforcement learning
via reward decomposition. In IJCAI/ECAI workshop
on explainable artificial intelligence, page 7.
Lipton, Z. C. (2018). The mythos of model interpretability.
Commun. ACM, 61(10):36–43.
Madumal, P., Miller, T., Sonenberg, L., and Vetere, F.
(2020). Explainable reinforcement learning through a
causal lens. In AAAI, pages 2493–2500. AAAI Press.
Milani, S., Topin, N., Veloso, M., and Fang, F. (2022). A
survey of explainable reinforcement learning. CoRR,
abs/2202.08434.
Mnih, V., Kavukcuoglu, K., Silver, D., Rusu, A. A., Ve-
ness, J., Bellemare, M. G., Graves, A., Riedmiller,
M., Fidjeland, A. K., Ostrovski, G., Petersen, S.,
Beattie, C., Sadik, A., Antonoglou, I., King, H., Ku-
maran, D., Wierstra, D., Legg, S., and Hassabis, D.
(2015). Human-level control through deep reinforce-
ment learning. Nature, 518(7540):529–533.
Sequeira, P. and Gervasio, M. T. (2020). Interestingness
elements for explainable reinforcement learning: Un-
derstanding agents’ capabilities and limitations. Artif.
Intell., 288:103367.
Shu, T., Xiong, C., and Socher, R. (2017). Hierarchical and
interpretable skill acquisition in multi-task reinforce-
ment learning. CoRR, abs/1712.07294.
Sutton, R. S. and Barto, A. G. (2018). Reinforcement learn-
ing: An introduction. MIT press.
Tsirtsis, S., De, A., and Rodriguez, M. (2021). Coun-
terfactual explanations in sequential decision making
under uncertainty. In Ranzato, M., Beygelzimer, A.,
Dauphin, Y. N., Liang, P., and Vaughan, J. W., editors,
NeurIPS 2021, pages 30127–30139.
van der Waa, J., van Diggelen, J., van den Bosch, K., and
Neerincx, M. A. (2018). Contrastive explanations for
reinforcement learning in terms of expected conse-
quences. CoRR, abs/1807.08706.
Watkins, C. J. and Dayan, P. (1992). Q-learning. Machine
learning, 8(3):279–292.
Yau, H., Russell, C., and Hadfield, S. (2020). What did
you think would happen? explaining agent behaviour
through intended outcomes. In Larochelle, H., Ran-
zato, M., Hadsell, R., Balcan, M., and Lin, H., editors,
NeurIPS.
ICAART 2023 - 15th International Conference on Agents and Artificial Intelligence
44