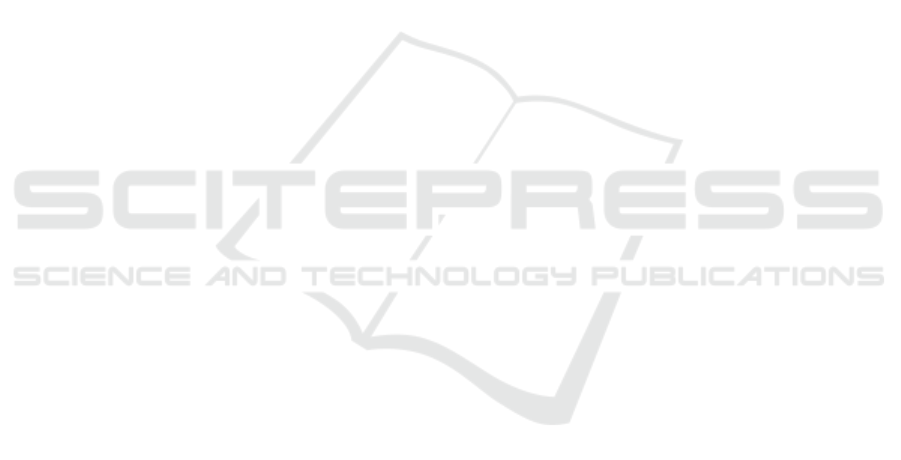
interesting to jointly solve the aspect term extraction
and the other subtasks of the Aspect Based Sentiment
Analysis.
REFERENCES
Abdul-Mageed, M., Elmadany, A., and Nagoudi, E. M. B.
(2020). Arbert & marbert: deep bidirectional trans-
formers for arabic. arXiv preprint arXiv:2101.01785.
Al-Dabet, S., Tedmori, S., and Al-Smadi, M. (2020). Ex-
tracting opinion targets using attention-based neural
model. SN Computer Science, 1(5):1–10.
Al-Smadi, M., Qawasmeh, O., Talafha, B., and Quwaider,
M. (2015). Human annotated arabic dataset of book
reviews for aspect based sentiment analysis. In 2015
3rd International Conference on Future Internet of
Things and Cloud, pages 726–730. IEEE.
Al-Smadi, M., Talafha, B., Al-Ayyoub, M., and Jararweh,
Y. (2019). Using long short-term memory deep neural
networks for aspect-based sentiment analysis of arabic
reviews. International Journal of Machine Learning
and Cybernetics, 10(8):2163–2175.
Antoun, W., Baly, F., and Hajj, H. (2020). Arabert:
Transformer-based model for arabic language under-
standing. arXiv preprint arXiv:2003.00104.
Bahdanau, D., Cho, K., and Bengio, Y. (2014). Neural ma-
chine translation by jointly learning to align and trans-
late. arXiv preprint arXiv:1409.0473.
Bensoltane, R. and Zaki, T. (2022). Towards arabic aspect-
based sentiment analysis: a transfer learning-based
approach. Social Network Analysis and Mining,
12(1):1–16.
Bing, L. (2012). Sentiment analysis and opinion mining
(synthesis lectures on human language technologies).
University of Illinois: Chicago, IL, USA.
Brownlee, J. (2021). Encoder-decoder recurrent neural net-
work models for neural machine translation. Machine
Learning Mastery.
Cho, K., Van Merri
¨
enboer, B., Gulcehre, C., Bahdanau, D.,
Bougares, F., Schwenk, H., and Bengio, Y. (2014).
Learning phrase representations using rnn encoder-
decoder for statistical machine translation. arXiv
preprint arXiv:1406.1078.
Chouikhi, H., Chniter, H., and Jarray, F. (2021). Arabic
sentiment analysis using bert model. In International
Conference on Computational Collective Intelligence,
pages 621–632. Springer.
Chouikhi., H., Chniter., H., and Jarray., F. (2021). Stack-
ing bert based models for arabic sentiment analysis.
In Proceedings of the 13th International Joint Con-
ference on Knowledge Discovery, Knowledge Engi-
neering and Knowledge Management - KEOD,, pages
144–150. INSTICC, SciTePress.
Devlin, J., Chang, M.-W., Lee, K., and Toutanova, K.
(2018). Bert: Pre-training of deep bidirectional trans-
formers for language understanding. arXiv preprint
arXiv:1810.04805.
Fadel, A. S., Saleh, M. E., and Abulnaja, O. A. (2022).
Arabic aspect extraction based on stacked contextu-
alized embedding with deep learning. IEEE Access,
10:30526–30535.
Fouad, M. M., Mahany, A., and Katib, I. (2019). Masdar: a
novel sequence-to-sequence deep learning model for
arabic stemming. In Proceedings of SAI Intelligent
Systems Conference, pages 363–373. Springer.
Inoue, G., Alhafni, B., Baimukan, N., Bouamor, H., and
Habash, N. (2021). The interplay of variant, size, and
task type in arabic pre-trained language models. arXiv
preprint arXiv:2103.06678.
Kingma, D. P. and Ba, J. (2014). Adam: A
method for stochastic optimization. arXiv preprint
arXiv:1412.6980.
Mohammad, A.-S., Qwasmeh, O., Talafha, B., Al-Ayyoub,
M., Jararweh, Y., and Benkhelifa, E. (2016). An en-
hanced framework for aspect-based sentiment analy-
sis of hotels’ reviews: Arabic reviews case study. In
2016 11th International conference for internet tech-
nology and secured transactions (ICITST), pages 98–
103. IEEE.
Nallapati, R., Zhou, B., Gulcehre, C., Xiang, B.,
et al. (2016). Abstractive text summarization us-
ing sequence-to-sequence rnns and beyond. arXiv
preprint arXiv:1602.06023.
Naous, T., Antoun, W., Mahmoud, R. A., and Hajj, H.
(2021). Empathetic bert2bert conversational model:
Learning arabic language generation with little data.
arXiv preprint arXiv:2103.04353.
Oueslati, O., Cambria, E., HajHmida, M. B., and Ounelli,
H. (2020). A review of sentiment analysis research
in arabic language. Future Generation Computer Sys-
tems, 112:408–430.
Pontiki, M., Galanis, D., Papageorgiou, H., Androutsopou-
los, I., Manandhar, S., Al-Smadi, M., Al-Ayyoub,
M., Zhao, Y., Qin, B., De Clercq, O., et al. (2016).
Semeval-2016 task 5: Aspect based sentiment anal-
ysis. In International workshop on semantic evalua-
tion, pages 19–30.
Radford, A., Narasimhan, K., Salimans, T., Sutskever, I.,
et al. (2018). Improving language understanding by
generative pre-training.
Radford, A., Wu, J., Child, R., Luan, D., Amodei, D.,
Sutskever, I., et al. (2019). Language models are un-
supervised multitask learners. OpenAI blog, 1(8):9.
Rothe, S., Narayan, S., and Severyn, A. (2020). Leverag-
ing pre-trained checkpoints for sequence generation
tasks. Transactions of the Association for Computa-
tional Linguistics, 8:264–280.
Safaya, A., Abdullatif, M., and Yuret, D. (2020). Kuisail at
semeval-2020 task 12: Bert-cnn for offensive speech
identification in social media. In Proceedings of the
Fourteenth Workshop on Semantic Evaluation, pages
2054–2059.
Saidi, R. and Jarray, F. (2022). Combining bert repre-
sentation and pos tagger for arabic word sense dis-
ambiguation. In International Conference on Intel-
ligent Systems Design and Applications, pages 676–
685. Springer.
ICAART 2023 - 15th International Conference on Agents and Artificial Intelligence
122