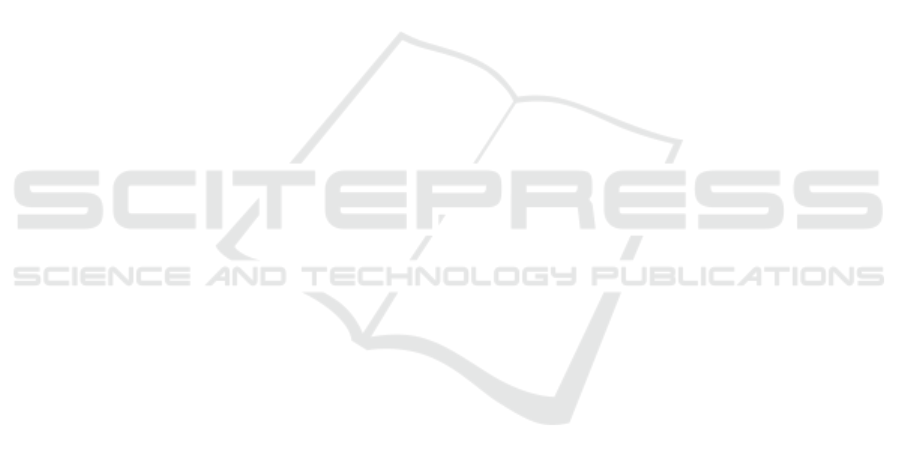
Iwashita, Y., Ogawara, K., and Kurazume, R. (2014). Iden-
tification of people walking along curved trajectories.
Pattern Recognition Letters, 48:60–69.
Kopp, S., Gesellensetter, L., Kr
¨
amer, N. C., and
Wachsmuth, I. (2005). A conversational agent as mu-
seum guide – design and evaluation of a real-world
application. In Panayiotopoulos, T., Gratch, J., Aylett,
R., Ballin, D., Olivier, P., and Rist, T., editors, Intel-
ligent Virtual Agents, pages 329–343, Berlin, Heidel-
berg. Springer Berlin Heidelberg.
Le, H. V., Tsuyoshi, and Iguchi, M. (2018). Deep mod-
ular multimodal fusion on multiple sensors for vol-
cano activity recognition. In Machine Learning and
Knowledge Discovery in Databases, pages 602–617.
Springer International Publishing.
Lecun, Y., Bottou, L., Bengio, Y., and Haffner, P. (1998).
Gradient-based learning applied to document recogni-
tion. Proceedings of the IEEE, 86(11):2278–2324.
Leder, H., Belke, B., Oeberst, A., and Augustin, D. (2004).
A model of aesthetic appreciation and aesthetic judg-
ments. British Journal of Psychology, 95(4):489–508.
Leder, H., Carbon, C.-C., and Ripsas, A.-L. (2006). Enti-
tling art: Influence of title information on understand-
ing and appreciation of paintings. Acta Psychologica,
121(2):176–198.
Li, X., Makihara, Y., Xu, C., Yagi, Y., and Ren, M. (2018).
Gait-based human age estimation using age group-
dependent manifold learning and regression. Multi-
media Tools and Applications, 77(21):28333–28354.
Liu, J., Akash, K., Misu, T., and Wu, X. (2021). Clus-
tering human trust dynamics for customized real-time
prediction. In Proceedings of 2021 IEEE Interna-
tional Intelligent Transportation Systems Conference
(ITSC’21), pages 1705–1712.
L
¨
oning, M., Bagnall, A. J., Ganesh, S., Kazakov, V.,
Lines, J., and Kir
´
aly, F. J. (2019). sktime: A uni-
fied interface for machine learning with time series.
arXiv:1909.07872 [cs.LG].
Martella, C., Miraglia, A., Frost, J., Cattani, M., and van
Steen, M. (2017). Visualizing, clustering, and predict-
ing the behavior of museum visitors. Pervasive and
Mobile Computing, 38:430–443.
Monfort, S. S., Graybeal, J. J., Harwood, A. E., McKnight,
P. E., and Shaw, T. H. (2017). A single-item assess-
ment for remaining mental resources: development
and validation of the gas tank questionnaire (GTQ).
Theoretical Issues in Ergonomics Science, 19(5):530–
552.
Ngo, T. T., Makihara, Y., Nagahara, H., Mukaigawa, Y.,
and Yagi, Y. (2014). The largest inertial sensor-
based gait database and performance evaluation of
gait-based personal authentication. Pattern Recogni-
tion, 47(1):228–237.
Parietti, F. and Asada, H. H. (2013). Dynamic analysis and
state estimation for wearable robotic limbs subject to
human-induced disturbances. In Proceedings of 2013
IEEE International Conference on Robotics and Au-
tomation (ICRA’13), pages 3880–3887.
Pedregosa, F., Varoquaux, G., Gramfort, A., Michel, V.,
Thirion, B., Grisel, O., Blondel, M., Prettenhofer,
P., Weiss, R., Dubourg, V., Vanderplas, J., Passos,
A., Cournapeau, D., Brucher, M., Perrot, M., and
Duchesnay, E. (2011). Scikit-learn: Machine learning
in Python. Journal of Machine Learning Research,
12:2825–2830.
Triloka, J., Senanayake, S. M. N. A., and Lai, D. (2016).
Neural computing for walking gait pattern identifi-
cation based on multi-sensor data fusion of lower
limb muscles. Neural Computing and Applications,
28(S1):65–77.
Vallez, N., Krauss, S., Espinosa-Aranda, J. L., Pagani, A.,
Seirafi, K., and Deniz, O. (2020). Automatic museum
audio guide. Sensors, 20(3):779.
Vaswani, A., Shazeer, N., Parmar, N., Uszkoreit, J., Jones,
L., Gomez, A. N., Kaiser, Ł., and Polosukhin, I.
(2017). Attention is all you need. Advances in neural
information processing systems (NIPS’17), 30:1–11.
Xu, Y., Lin, W., and Hu, Y. (2020). Stock trend pre-
diction using historical data and financial online
news. In Proceedings of 2020 IEEE Interna-
tional Conference on Parallel & Distributed Pro-
cessing with Applications, Big Data & Cloud
Computing, Sustainable Computing & Com-
munications, Social Computing & Networking
(ISPA/BDCloud/SocialCom/SustainCom’20), pages
1507–1512.
Yalowitz, S. S. and Bronnenkant, K. (2009). Timing and
tracking: Unlocking visitor behavior. Visitor Studies,
12(1):47–64.
Yoshimura, Y., Girardin, F., Carrascal, J. P., Ratti, C.,
and Blat, J. (2012). New tools for studying visi-
tor behaviours in museums: A case study at the lou-
vre. Information and Communication Technologies in
Tourism 2012, pages 391–402.
Zancanaro, M., Kuflik, T., Boger, Z., Goren-Bar, D., and
Goldwasser, D. (2007). Analyzing museum visitors’
behavior patterns. In Conati, C., McCoy, K., and
Paliouras, G., editors, User Modeling 2007, pages
238–246, Berlin, Heidelberg. Springer Berlin Heidel-
berg.
ICAART 2023 - 15th International Conference on Agents and Artificial Intelligence
64