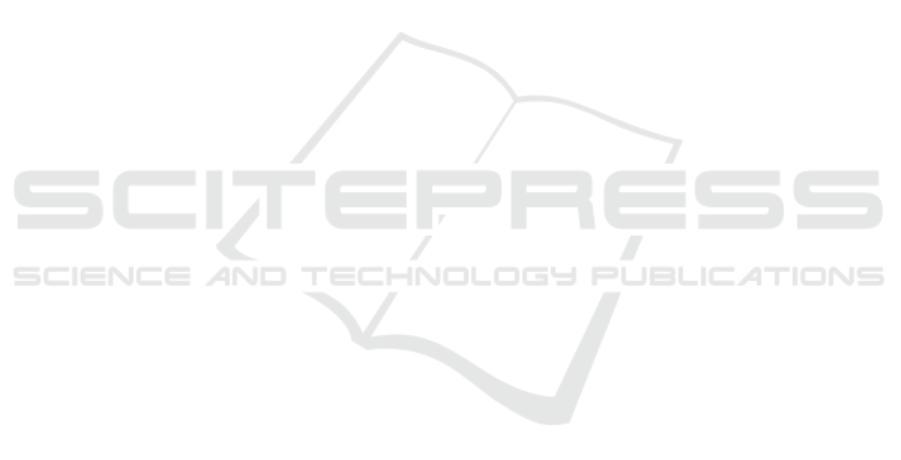
were any instances that belonged to the intersection
of the classes, but none were found. This demon-
strates the coherence of our KG, proving that it is free
of noise and contradicting information.
Our POI management mechanism received strong
F1 scores for both the visual (93.1%) and textual
(95.6%) message, demonstrating that it can be utilized
independently to create/update POIs in a broad me-
dia planning scenario. Additionally, we can comment
that this occurred when updating POIs, which means
that new information could not be added to POIs that
already existed in the area, and were missing relevant
instances for both textual and visual messages. The
updated messages were straying outside of the bound-
ing boxes of all existing POIs because each POI has
a box around it. Be aware that the POIs’ bounding
boxes are part of a broader bounding box that encom-
passes the area that requires news coverage. It seems
reasonable to take into account a bounding box for the
POIs and the area that requires news coverage; other-
wise, we risk adding POIs to the area that are situated
in a completely other area of the map.
The high Recall scores—100% for both the visual
and textual messages—can also be mentioned. This
essentially indicates that there were no textual or vi-
sual messages that indicated an error. If we examine
the two scenarios in which an error may be returned,
the reason for not doing so is pretty clear: (i) The mes-
sage’s coordinates do not fall within a bounding box
that designates the location where an event has oc-
curred, or (ii) The user will identify a non-matching
category-subcategory tuple. It is difficult for the user
to choose the incorrect selection in both cases since
the user sends messages using a mobile application
(which is now private) that displays the permissible
category-subcategory tuples, and the bounding box
with a blue hue over an area.
In terms of future work, we intend to provide a
method that will make the POIs more beneficial while
making decisions. Additionally, we will provide POIs
with a list of tasks that must be taken in order to com-
plete a remote production mission more accurately.
ACKNOWLEDGEMENTS
This work has been funded by XR4DRAMA Horizon
2020 project, grant agreement number 952133.
REFERENCES
Andreadis, S., Moumtzidou, A., Apostolidis, K., Gkoun-
takos, K., Galanopoulos, D., Michail, E., Gialam-
poukidis, I., Vrochidis, S., Mezaris, V., and Kompat-
siaris, I. (2020). Verge in vbs 2020. In International
Conference on Multimedia Modeling, pages 778–783.
Springer.
Batziou, E., Ioannidis, K., Patras, I., Vrochidis, S., and
Kompatsiaris, I. (2023). Low-light image enhance-
ment based on u-net and haar. In In Proceedings of the
29th International Conference on Multimedia Model-
ing (MMM 2023). Springer.
Berners-Lee, T., Hendler, J., and Lassila, O. (2001). The
semantic web. Scientific american, 284(5):34–43.
Berven, A., Christensen, O. A., Moldeklev, S., Opdahl,
A. L., and Villanger, K. J. (2018). News hunter: build-
ing and mining knowledge graphs for newsroom sys-
tems. NOKOBIT, 26:1–11.
Ehrlinger, L. and W
¨
oß, W. (2016). Towards a definition
of knowledge graphs. SEMANTiCS (Posters, Demos,
SuCCESS), 48(1-4):2.
Ni, J., Liu, X., Zhou, Q., and Cao, L. (2019). A knowl-
edge graph based disaster storyline generation frame-
work. In 2019 Chinese Control And Decision Confer-
ence (CCDC), pages 4432–4437. IEEE.
Opdahl, A. L., Al-Moslmi, T., Dang-Nguyen, D.-T.,
Gallofr
´
e Oca
˜
na, M., Tessem, B., and Veres, C. (2022).
Semantic knowledge graphs for the news: A review.
ACM Computing Surveys (CSUR).
Opdahl, A. L., Berven, A., Alipour, K., Christensen, O. A.,
and Villanger, K. J. (2016). Knowledge graphs for
newsroom systems. NOKOBIT, 24:1–4.
Pan, J. Z., Pavlova, S., Li, C., Li, N., Li, Y., and Liu,
J. (2018). Content based fake news detection us-
ing knowledge graphs. In International semantic web
conference, pages 669–683. Springer.
Qiu, W., Li, W., Liu, X., and Huang, X. (2021). Subjective
street scene perceptions for shanghai with large-scale
application of computer vision and machine learning.
Technical report, EasyChair.
Rospocher, M., van Erp, M., Vossen, P., Fokkens, A., Ald-
abe, I., Rigau, G., Soroa, A., Ploeger, T., and Bogaard,
T. (2016). Building event-centric knowledge graphs
from news. Journal of Web Semantics, 37:132–151.
Su
´
arez-Figueroa, M. C., G
´
omez-P
´
erez, A., and Villaz
´
on-
Terrazas, B. (2009). How to write and use the
ontology requirements specification document. In
OTM Confederated International Conferences” On
the Move to Meaningful Internet Systems”, pages
966–982. Springer.
Tang, J., Feng, Y., and Zhao, D. (2019). Learning to up-
date knowledge graphs by reading news. In Proceed-
ings of the 2019 Conference on Empirical Methods
in Natural Language Processing and the 9th Inter-
national Joint Conference on Natural Language Pro-
cessing (EMNLP-IJCNLP), pages 2632–2641.
Villazon-Terrazas, B., Ortiz-Rodriguez, F., Tiwari, S. M.,
and Shandilya, S. K. (2021). Knowledge graphs and
semantic web. Springer.
Wang, Y. and Hou, X. (2018). A method for constructing
knowledge graph of disaster news based on address
tree. In 2018 5th International Conference on Systems
and Informatics (ICSAI), pages 305–310. IEEE.
XR4DRAMA Knowledge Graph: A Knowledge Graph for Media Planning
131