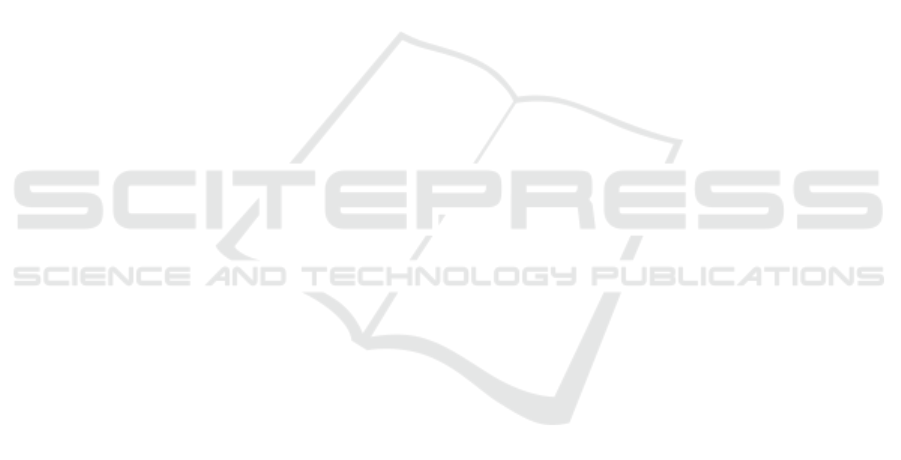
SalienceNet is a useful new step for nuclei seg-
mentation workflows.
7 CODE AVAILABILITY
SalienceNet network’s code for training and testing
nuclei enhancement is fully open source and available
on GitHub at https://github.com/cbib/SalienceNet.
Our best pre-trained model M used in this study is
also available from the same GitHub page.
REFERENCES
Bankhead, P., Loughrey, M. B., Fern
´
andez, J. A., Dom-
browski, Y., McArt, D. G., Dunne, P. D., McQuaid,
S., Gray, R. T., Murray, L. J., Coleman, H. G., et al.
(2017). Qupath: Open source software for digital
pathology image analysis. Scientific reports, 7(1):1–7.
Beghin, A., Grenci, G., Sahni, G., Guo, S., Rajendiran, H.,
Delaire, T., Mohamad Raffi, S. B., Blanc, D., de Mets,
R., Ong, H. T., et al. (2022). Automated high-speed 3d
imaging of organoid cultures with multi-scale pheno-
typic quantification. Nature Methods, 19(7):881–892.
Caicedo, J. C., Goodman, A., Karhohs, K. W., Cimini,
B. A., Ackerman, J., Haghighi, M., Heng, C., Becker,
T., Doan, M., McQuin, C., et al. (2019). Nucleus seg-
mentation across imaging experiments: the 2018 data
science bowl. Nature methods, 16(12):1247–1253.
Chouaib, R., Safieddine, A., Pichon, X., Imbert, A., Kwon,
O. S., Samacoits, A., Traboulsi, A.-M., Robert, M.-C.,
Tsanov, N., Coleno, E., Poser, I., Zimmer, C., Hyman,
A., Le Hir, H., Zibara, K., Peter, M., Mueller, F., Wal-
ter, T., and Bertrand, E. (2020). A dual protein-mrna
localization screen reveals compartmentalized trans-
lation and widespread co-translational rna targeting.
Developmental Cell, 54(6):773–791.e5.
De Chaumont, F., Dallongeville, S., Chenouard, N., Herv
´
e,
N., Pop, S., Provoost, T., Meas-Yedid, V., Panka-
jakshan, P., Lecomte, T., Le Montagner, Y., et al.
(2012). Icy: an open bioimage informatics platform
for extended reproducible research. Nature methods,
9(7):690.
Du, R., Li, W., Chen, S., Li, C., and Zhang, Y. (2021). Un-
paired underwater image enhancement based on cy-
clegan. Information, 13(1):1.
Ettinger, A. and Wittmann, T. (2014). Fluorescence live cell
imaging. Methods in cell biology, 123:77–94.
Galland, R., Grenci, G., Aravind, A., Viasnoff, V., Studer,
V., and Sibarita, J.-B. (2015). 3d high-and super-
resolution imaging using single-objective spim. Na-
ture methods, 12(7):641–644.
Gao, P., Tian, T., Li, L., Ma, J., and Tian, J. (2021). De-
cyclegan: An object enhancement network for weak
vehicle detection in satellite images. IEEE Journal
of Selected Topics in Applied Earth Observations and
Remote Sensing, 14:3403–3414.
G
´
omez-de Mariscal, E., Garc
´
ıa-L
´
opez-de Haro, C.,
Ouyang, W., Donati, L., Lundberg, E., Unser, M.,
Mu
˜
noz-Barrutia, A., and Sage, D. (2021). Deepim-
agej: A user-friendly environment to run deep learn-
ing models in imagej. Nature Methods, 18(10):1192–
1195.
Haase, R., Royer, L. A., Steinbach, P., Schmidt, D., Di-
brov, A., Schmidt, U., Weigert, M., Maghelli, N.,
Tomancak, P., Jug, F., et al. (2020). Clij: Gpu-
accelerated image processing for everyone. Nature
methods, 17(1):5–6.
He, J., Wang, C., Jiang, D., Li, Z., Liu, Y., and Zhang,
T. (2020). Cyclegan with an improved loss func-
tion for cell detection using partly labeled images.
IEEE Journal of Biomedical and Health Informatics,
24(9):2473–2480.
Hollandi, R., Moshkov, N., Paavolainen, L., Tasnadi, E.,
Piccinini, F., and Horvath, P. (2022). Nucleus seg-
mentation: towards automated solutions. Trends in
Cell Biology.
Hollandi, R., Szkalisity, A., Toth, T., Tasnadi, E., Molnar,
C., Mathe, B., Grexa, I., Molnar, J., Balind, A., Gorbe,
M., et al. (2020). nucleaizer: a parameter-free deep
learning framework for nucleus segmentation using
image style transfer. Cell Systems, 10(5):453–458.
Isola, P., Zhu, J.-Y., Zhou, T., and Efros, A. A. (2017).
Image-to-image translation with conditional adversar-
ial networks. In Proceedings of the IEEE conference
on computer vision and pattern recognition, pages
1125–1134.
Kanopoulos, N., Vasanthavada, N., and Baker, R. L. (1988).
Design of an image edge detection filter using the
sobel operator. IEEE Journal of solid-state circuits,
23(2):358–367.
Kim, Y. and Varshney, A. (2006). Saliency-guided enhance-
ment for volume visualization. IEEE Transactions
on Visualization and Computer Graphics, 12(5):925–
932.
Li, G., Liu, T., Tarokh, A., Nie, J., Guo, L., Mara, A., Hol-
ley, S., and Wong, S. T. (2007). 3d cell nuclei seg-
mentation based on gradient flow tracking. BMC cell
biology, 8(1):1–10.
Lu, Z. and Chen, Y. (2022). Single image super-resolution
based on a modified u-net with mixed gradient loss.
signal, image and video processing, 16(5):1143–
1151.
Malpica, N., De Sol
´
orzano, C. O., Vaquero, J. J., Santos, A.,
Vallcorba, I., Garc
´
ıa-Sagredo, J. M., and Del Pozo, F.
(1997). Applying watershed algorithms to the seg-
mentation of clustered nuclei. Cytometry: The Jour-
nal of the International Society for Analytical Cytol-
ogy, 28(4):289–297.
McQuin, C., Goodman, A., Chernyshev, V., Kamentsky, L.,
Cimini, B. A., Karhohs, K. W., Doan, M., Ding, L.,
Rafelski, S. M., Thirstrup, D., et al. (2018). Cellpro-
filer 3.0: Next-generation image processing for biol-
ogy. PLoS biology, 16(7):e2005970.
Pang, Y., Lin, J., Qin, T., and Chen, Z. (2021). Image-to-
image translation: Methods and applications. IEEE
Transactions on Multimedia.
BIOIMAGING 2023 - 10th International Conference on Bioimaging
50