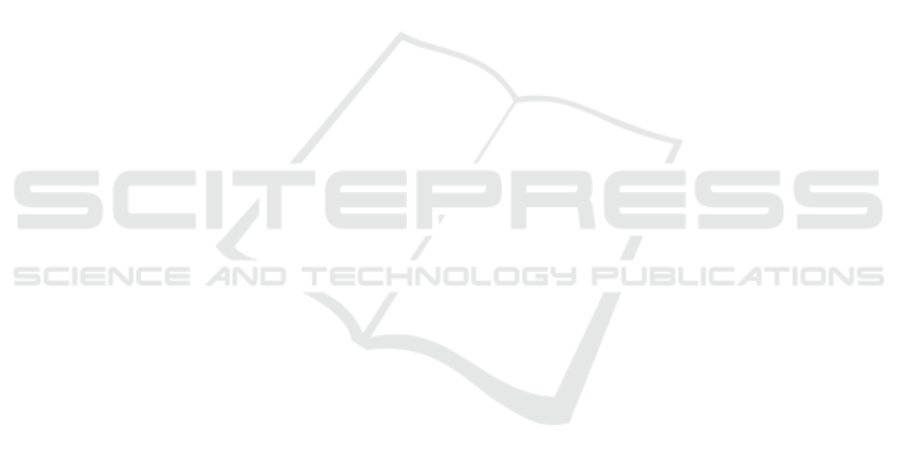
360: An Extensible Toolkit for Detecting, Under-
standing, and Mitigating Unwanted Algorithmic Bias.
arXiv:1810.01943 [cs]. arXiv: 1810.01943.
Bogen, M. (2019). All the Ways Hiring Algorithms Can
Introduce Bias. Harvard Business Review. Section:
Hiring.
Carrera, F. (2020). Race and gender of aesthetics and
affections: algorithmization of racism and sexism
in contemporary digital image databases. Matrizes,
14(2):217–240.
Chiang, C. and Saw, Y. (2018). Do good looks matter when
applying for jobs in the hospitality industry? Interna-
tional Journal of Hospitality Management.
Choi, Y., Uh, Y., Yoo, J., and Ha, J.-W. (2020). StarGAN
v2: Diverse Image Synthesis for Multiple Domains.
arXiv:1912.01865 [cs]. arXiv: 1912.01865.
Danner, M., Weber, T., Peng, L. P., Gerlach, T., Su, X.,
and R
¨
atsch, M. (2021). Ethically aligned deep learn-
ing: Unbiased facial aesthetic prediction. CoRR,
abs/2111.05149.
Deng, J., Dong, W., Socher, R., Li, L., Kai Li, and Li
Fei-Fei (2009). ImageNet: A large-scale hierarchical
image database. In 2009 IEEE Conference on Com-
puter Vision and Pattern Recognition, pages 248–255.
ISSN: 1063-6919.
Dornaika, F., Moujahid, A., Wang, K., and Feng, X. (2020).
Efficient deep discriminant embedding: Application
to face beauty prediction and classification. Engineer-
ing Applications of Artificial Intelligence, 95:103831.
Gerlach, T., Danner, M., Peng, L., Kaminickas, A., Fei, W.,
and R
¨
atsch, M. (2020). Who Loves Virtue as much
as He Loves Beauty?: Deep Learning based Estimator
for Aesthetics of Portraits:. In Proceedings of the 15th
International Joint Conference on Computer Vision,
Imaging and Computer Graphics Theory and Applica-
tions, pages 521–528, Valletta, Malta. SCITEPRESS
- Science and Technology Publications.
Grant, R. M. (1991). The resource-based theory of compet-
itive advantage: Implications for strategy formulation.
California Management Review.
Gupta, S. and Gupta, A. (2019). Dealing with Noise Prob-
lem in Machine Learning Data-sets: A Systematic Re-
view. Procedia Computer Science, 161:466–474.
Gyawali, D., Pokharel, P., Chauhan, A., and Shakya, S. C.
(2020). Age Range Estimation Using MTCNN and
VGG-Face Model. In 2020 11th International Confer-
ence on Computing, Communication and Networking
Technologies (ICCCNT), pages 1–6.
He, K., Zhang, X., Ren, S., and Sun, J. (2015). Deep
residual learning for image recognition. CoRR,
abs/1512.03385.
Kingma, D. P. and Ba, J. (2017). Adam: A Method
for Stochastic Optimization. arXiv:1412.6980 [cs].
arXiv: 1412.6980.
Krizhevsky, A., Sutskever, I., and Hinton, G. E. (2017). Im-
ageNet classification with deep convolutional neural
networks. Communications of the ACM, 60(6):84–90.
K
¨
arkk
¨
ainen, K. and Joo, J. (2019). FairFace: Face At-
tribute Dataset for Balanced Race, Gender, and Age.
arXiv:1908.04913 [cs]. arXiv: 1908.04913.
Laurim, V., Arpaci, S., Prommegger, B., and Krcmar, H.
(2021). Computer, whom should i hire?–acceptance
criteria for artificial intelligence in the recruitment
process. In Proceedings of the 54th Hawaii Interna-
tional Conference on System Sciences, page 5495.
Levin, S. (2016). A beauty contest was judged by AI and
the robots didn’t like dark skin. Section: Technology.
Liang, L., Lin, L., Jin, L., Xie, D., and Li, M.
(2018). SCUT-FBP5500: A Diverse Benchmark
Dataset for Multi-Paradigm Facial Beauty Prediction.
arXiv:1801.06345 [cs]. arXiv: 1801.06345.
Little, A. C., Jones, B. C., and DeBruine, L. M. (2011).
Facial attractiveness: evolutionary based research.
Philosophical Transactions of the Royal Society B: Bi-
ological Sciences.
Lloyd, K. (2018). Bias amplification in artificial intelli-
gence systems.
Parkhi, O. M., Vedaldi, A., and Zisserman, A. (2015).
Deep Face Recognition. In Procedings of the British
Machine Vision Conference 2015, pages 41.1–41.12,
Swansea. British Machine Vision Association.
Prijatelj, D. S., McCurrie, M., and Scheirer, W. J. (2020).
A Bayesian Evaluation Framework for Ground Truth-
Free Visual Recognition Tasks. arXiv:2007.06711 [cs,
stat]. arXiv: 2007.06711.
Reilly, P. (2018). The impact of artificial intelligence on the
hr function.
Schlick, A. M. and Reich, K. (2021). Vereinbarkeit von
akzeptanzfaktoren beim einsatz von ki in der bewer-
berauswahl. Master Thesis, Reutlingen University.
Shank, D. B. and DeSanti, A. (2018). Attributions of moral-
ity and mind to artificial intelligence after real-world
moral violations. Computers in Human Behavior,
86:401–411.
Sharma, S., Zhang, Y., R
´
ıos Aliaga, J. M., Bouneffouf, D.,
Muthusamy, V., and Varshney, K. R. (2020). Data
Augmentation for Discrimination Prevention and Bias
Disambiguation. In Proceedings of the AAAI/ACM
Conference on AI, Ethics, and Society, AIES ’20,
pages 358–364, New York, NY, USA. Association for
Computing Machinery.
Shelhamer, E., Long, J., and Darrell, T. (2017). Fully con-
volutional networks for semantic segmentation. IEEE
Trans. Pattern Anal. Mach. Intell., 39(4):640–651.
Simonyan, K. and Zisserman, A. (2015). Very deep con-
volutional networks for large-scale image recognition.
In Bengio, Y. and LeCun, Y., editors, 3rd Interna-
tional Conference on Learning Representations, ICLR
2015, San Diego, CA, USA, May 7-9, 2015, Confer-
ence Track Proceedings.
Xie, D., Liang, L., Jin, L., Xu, J., and Li, M. (2015). SCUT-
FBP: A benchmark dataset for facial beauty percep-
tion. In 2015 IEEE International Conference on Sys-
tems, Man, and Cybernetics, Kowloon Tong, Hong
Kong, October 9-12, 2015, pages 1821–1826. IEEE.
Zeiler, M. D. and Fergus, R. (2013). Visualizing and Under-
standing Convolutional Networks. arXiv:1311.2901
[cs]. arXiv: 1311.2901.
Zhang, M. and Kreiman, G. (2021). Beauty is in the eye of
the machine. In Nat Hum Behav 5, 675–676 ().
Overcome Ethnic Discrimination with Unbiased Machine Learning for Facial Data Sets
471