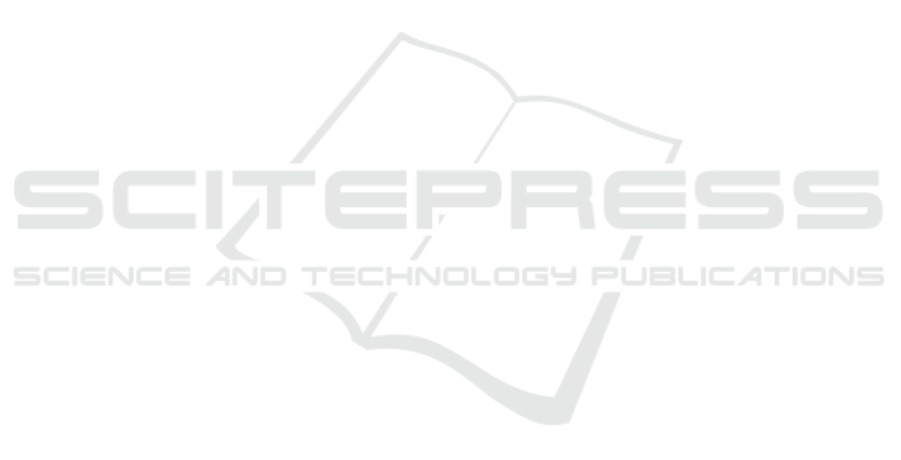
PCTL properties to check the adequacy of the model.
We also ran an experiment to explore the sensitivity
of inhibitory control to the modulation of some con-
nections. The modified model complies with Parkin-
son’s disease. Further modifications to represent, e.g.,
Alzheimer’s disease are planned as future work.
Probabilistic formal models can represent a wide
variety of behaviors while enabling model checking.
To check our model with standard tools, it was neces-
sary to brought up a new generalization of the LI&F
classical neuron model to represent small networks
behavior with a single module. This work opens new
avenues for the formal modeling of cognitive func-
tions. Moreover, it has proven the feasibility of such
model exploration using only off the shelf laptops.
In the future, the model will be coupled with the
activity model of a patient playing a serious game tar-
geting the inhibitory control function. The goal is to
explore modifications in the brain neural network that
may generate a patient behavior characteristic of neu-
rocognitive disorders.
REFERENCES
Braak, H., Tredici, K. D., Bratzke, H., Hamm-Clement, J.,
Sandmann-Keil, D., and R
¨
ub, U. (2002). Staging of
the intracerebral inclusion body pathology associated
with idiopathic Parkinson's disease (preclinical and
clinical stages). Journal of Neurology, 249.
Brunel, N. and Van Rossum, M. C. (2007). Lapicque’s 1907
paper: from frogs to integrate-and-fire. Biological cy-
bernetics, 97.
Cheng, H. C., Ulane, C. M., and Burke, R. E. (2010). Clin-
ical progression in Parkinson disease and the neurobi-
ology of axons. Annals of Neurology, 67.
Clarke, E. M., Emerson, E. A., and Sistla, A. P. (1986).
Automatic verification of finite-state concurrent sys-
tems using temporal logic specifications. ACM Trans-
actions on Programming Languages and Systems
(TOPLAS).
Crawford, T., Higham, S., Renvoize, T., Patel, J., Dale, M.,
Suriya, A., and Tetley, S. (2005). Inhibitory control of
saccadic eye movements and cognitive impairment in
Alzheimer’s disease. Biological Psychiatry, 57.
Graybiel, A. M. (2000). The basal ganglia. Current biology,
10.
Hansson, H. and Jonsson, B. (1994). A logic for reasoning
about time and reliability. Formal aspects of comput-
ing.
Heeger, D. (2000). Poisson model of spike generation.
Handout, University of Standford, 5.
Hopfield, J. J. (1982). Neural networks and physical sys-
tems with emergent collective computational abilities.
Proceedings of the national academy of sciences, 79.
Hu, S., Chao, H. H.-A., Zhang, S., Ide, J. S., and Li, C.-
S. R. (2014). Changes in cerebral morphometry and
amplitude of low-frequency fluctuations of bold sig-
nals during healthy aging: correlation with inhibitory
control. Brain Structure and Function, 219.
Inoue, H., Hukushima, K., and Omori, T. (2021). Esti-
mation of Neuronal Dynamics of Izhikevich Neuron
Models from Spike-Train Data with Particle Markov
Chain Monte Carlo Method. Journal of the Physical
Society of Japan, 90.
Izhikevich, E. M. (2004). Which model to use for cortical
spiking neurons? IEEE transactions on neural net-
works, 15.
Jahanshahi, M., Obeso, I., Rothwell, J. C., and Obeso,
J. A. (2015). A fronto–striato–subthalamic–pallidal
network for goal-directed and habitual inhibition. Na-
ture Reviews Neuroscience, 16.
Johns, P. (2014). Chapter 3 - functional neuroanatomy.
In Johns, P., editor, Clinical Neuroscience. Churchill
Livingstone.
Kiely, K. M. (2014). Cognitive function. In Michalos, A. C.,
editor, Encyclopedia of Quality of Life and Well-Being
Research. Springer Netherlands, Dordrecht.
Kwiatkowska, M., Norman, G., and Parker, D. (2011).
PRISM 4.0: Verification of probabilistic real-time sys-
tems. In Proc. 23rd Int. Conf. on Computer Aided Ver-
ification (CAV’11).
Mink, J. W. (1996). The basal ganglia: Focused selec-
tion and inhibition of competing motor programs.
Progress in Neurobiology, 50.
Nossenson, N. and Messer, H. (2010). Modeling neuron fir-
ing pattern using a two state markov chain. In 2010
IEEE Sensor Array and Multichannel Signal Process-
ing Workshop.
Paugam-Moisy, H. and Bohte, S. M. (2012). Computing
with spiking neuron networks. Handbook of natural
computing, 1.
Purves, D., Augustine, G.-J., Fitzpatrick, D., and Hall, W.
(2019). Neurosciences. Broch
´
e.
Sakumura, Y., Konno, N., and Aihara, K. (2001). Markov
chain model approximating the hodgkin-huxley neu-
ron. In Dorffner, G., Bischof, H., and Hornik, K.,
editors, Artificial Neural Networks — ICANN 2001,
Berlin, Heidelberg. Springer Berlin Heidelberg.
Schall, J. D. and Godlove, D. C. (2012). Current advances
and pressing problems in studies of stopping. Current
Opinion in Neurobiology, 22. Decision making.
Schmidt, R., Herrojo Ruiz, M., Kilavik, B. E., Lundqvist,
M., Starr, P. A., and Aron, A. R. (2019). Beta oscilla-
tions in working memory, executive control of move-
ment and thought, and sensorimotor function. Journal
of Neuroscience, 39.
Schmidt, R., Leventhal, D. K., Mallet, N., Chen, F., and
Berke, J. D. (2013). Canceling actions involves a
race between basal ganglia pathways. Nature neuro-
science, 16.
Schroll, H. and Hamker, F. (2013). Computational models
of basal-ganglia pathway functions: focus on func-
tional neuroanatomy. Frontiers in Systems Neuro-
science, 7.
Verbruggen, F. and Logan, G. D. (2009). Models of re-
sponse inhibition in the stop-signal and stop-change
A Formal Probabilistic Model of the Inhibitory Control Circuit in the Brain
153