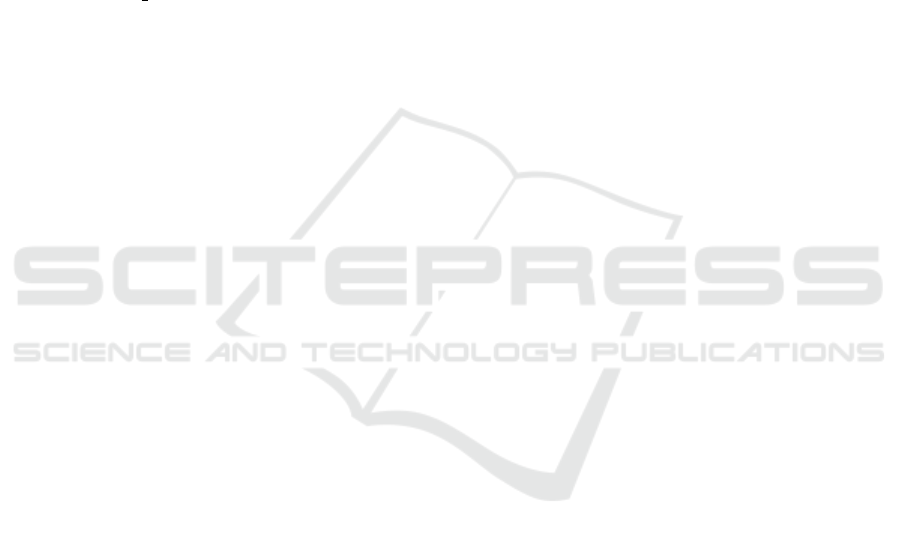
Kang, G., Li, J., and Tao, D. (2018). Shakeout: A
new approach to regularized deep neural net-
work training. IEEE Transactions on Pattern
Analysis and Machine Intelligence, 40(5):1245–
1258.
Liang, T., Glossner, J., Wang, L., Shi, S., and Zhang,
X. (2021). Pruning and quantization for deep
neural network acceleration: A survey. Neuro-
computing, 461:370–403.
Longuetaud, F., Pot, G., Mothe, F., Barthelemy, A.,
Decelle, R., Delconte, F., Ge, X., Guillaume,
G., Mancini, T., Ravoajanahary, T., et al. (2022).
Traceability and quality assessment of douglas
fir (pseudotsuga menziesii (mirb.) franco) logs:
the treetrace douglas database. Annals of Forest
Science, 79(1):1–21.
Luo, J.-H., Wu, J., and Lin, W. (2017). Thinet: A fil-
ter level pruning method for deep neural network
compression. In Proceedings of the IEEE inter-
national conference on computer vision, pages
5058–5066.
Mellouli, D., Hamdani, T. M., Sanchez-Medina, J. J.,
Ayed, M. B., and Alimi, A. M. (2019). Mor-
phological convolutional neural network archi-
tecture for digit recognition. IEEE transac-
tions on neural networks and learning systems,
30(9):2876–2885.
Misra, D., Nalamada, T., Arasanipalai, A. U., and
Hou, Q. (2021). Rotate to attend: Convolutional
triplet attention module. In Proceedings of the
IEEE/CVF Winter Conference on Applications
of Computer Vision, pages 3139–3148.
Mondal, R., Purkait, P., Santra, S., and Chanda, B.
(2019). Morphological networks for image de-
raining. In International Conference on Discrete
Geometry for Computer Imagery, pages 262–
275. Springer.
Oktay, O., Schlemper, J., Folgoc, L. L., Lee, M.,
Heinrich, M., Misawa, K., Mori, K., McDonagh,
S., Hammerla, N. Y., Kainz, B., et al. (2018).
Attention u-net: Learning where to look for the
pancreas. arXiv preprint arXiv:1804.03999.
Raatevaara, A., Korpunen, H., Tiitta, M., Tomppo,
L., Kulju, S., Antikainen, J., and Uusitalo, J.
(2020). Electrical impedance and image analysis
methods in detecting and measuring scots pine
heartwood from a log end during tree harvest-
ing. Computers and Electronics in Agriculture,
177:105690.
Ronneberger, O., Fischer, P., and Brox, T. (2015). U-
net: Convolutional networks for biomedical im-
age segmentation. In International Conference
on Medical image computing and computer-
assisted intervention, pages 234–241. Springer.
Ruifrok, A. C., Johnston, D. A., et al. (2001). Quan-
tification of histochemical staining by color de-
convolution. Analytical and quantitative cytol-
ogy and histology, 23(4):291–299.
Schraml, R. and Uhl, A. (2014). Similarity based
cross-section segmentation in rough log end im-
ages. In IFIP International Conference on Arti-
ficial Intelligence Applications and Innovations,
pages 614–623. Springer.
Trebing, K., Stanczyk, T., and Mehrkanoon, S.
(2021). Smaat-unet: Precipitation nowcasting
using a small attention-unet architecture. Pattern
Recognition Letters, 145:178–186.
Wimmer, G., Schraml, R., Hofbauer, H.,
Petutschnigg, A., and Uhl, A. (2021). Two-stage
cnn-based wood log recognition. arXiv preprint
arXiv:2101.04450.
Woo, S., Park, J., Lee, J.-Y., and Kweon, I. S. (2018).
Cbam: Convolutional block attention module.
In Proceedings of the European conference on
computer vision (ECCV), pages 3–19.
Yang, H.-H., Yang, C.-H. H., and Wang, Y.-C. F.
(2020). Wavelet channel attention module with
a fusion network for single image deraining. In
2020 IEEE International Conference on Image
Processing (ICIP), pages 883–887. IEEE.
Zhu, B., Hofstee, P., Lee, J., and Al-Ars, Z. (2021).
An attention module for convolutional neural
networks. In International Conference on Artifi-
cial Neural Networks, pages 167–178. Springer.
ICPRAM 2023 - 12th International Conference on Pattern Recognition Applications and Methods
766