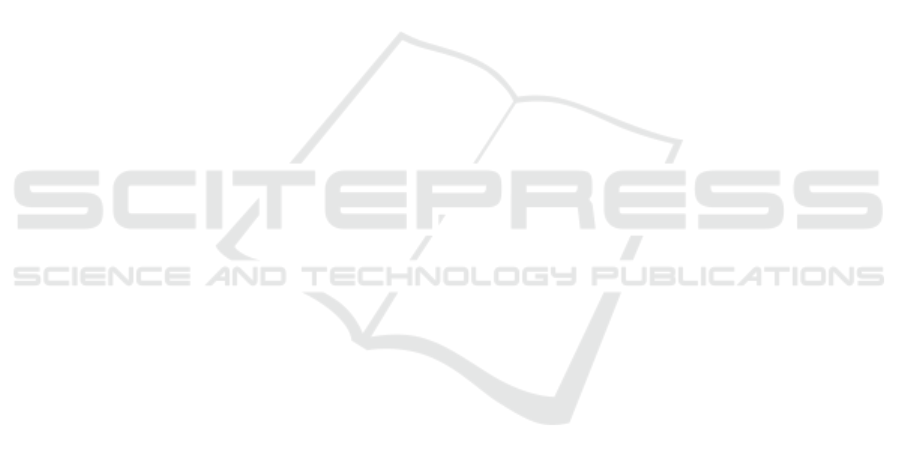
conference on computer vision and pattern recogni-
tion, pages 5297–5307.
Bai, Y., Ding, H., Qiao, Y., Marinovic, A., Gu, K., Chen,
T., Sun, Y., and Wang, W. (2019). Unsupervised in-
ductive graph-level representation learning via graph-
graph proximity. arXiv preprint arXiv:1904.01098.
Chatpatanasiri, R., Korsrilabutr, T., Tangchanachaianan, P.,
and Kijsirikul, B. (2010). A new kernelization frame-
work for mahalanobis distance learning algorithms.
Neurocomputing, 73(10-12):1570–1579.
Dey, S., Dutta, A., Toledo, J. I., Ghosh, S. K., Llad
´
os, J.,
and Pal, U. (2017). Signet: Convolutional siamese
network for writer independent offline signature veri-
fication. arXiv preprint arXiv:1707.02131.
Em, Y., Gag, F., Lou, Y., Wang, S., Huang, T., and Duan, L.-
Y. (2017). Incorporating intra-class variance to fine-
grained visual recognition. In 2017 IEEE Interna-
tional Conference on Multimedia and Expo (ICME),
pages 1452–1457. IEEE.
Hadsell, R., Chopra, S., and LeCun, Y. (2006). Dimen-
sionality reduction by learning an invariant mapping.
In 2006 IEEE Computer Society Conference on Com-
puter Vision and Pattern Recognition (CVPR’06), vol-
ume 2, pages 1735–1742. IEEE.
Harman, D. K. (1993). The first text retrieval conference
(TREC-1), volume 500. US Department of Com-
merce, National Institute of Standards and Technol-
ogy.
Hoffer, E. and Ailon, N. (2015). Deep metric learn-
ing using triplet network. In International workshop
on similarity-based pattern recognition, pages 84–92.
Springer.
Melekhov, I., Kannala, J., and Rahtu, E. (2016). Siamese
network features for image matching. In 2016 23rd in-
ternational conference on pattern recognition (ICPR),
pages 378–383. IEEE.
Mescheder, L., Oechsle, M., Niemeyer, M., Nowozin, S.,
and Geiger, A. (2019). Occupancy networks: Learn-
ing 3d reconstruction in function space. In Proceed-
ings of the IEEE/CVF Conference on Computer Vision
and Pattern Recognition, pages 4460–4470.
Nazir, D., Afzal, M. Z., Pagani, A., Liwicki, M., and
Stricker, D. (2021). Contrastive learning for 3d point
clouds classification and shape completion. Sensors,
21(21):7392.
Park, J. J., Florence, P., Straub, J., Newcombe, R., and
Lovegrove, S. (2019). Deepsdf: Learning continuous
signed distance functions for shape representation. In
Proceedings of the IEEE/CVF Conference on Com-
puter Vision and Pattern Recognition, pages 165–174.
Peng, S., Niemeyer, M., Mescheder, L., Pollefeys, M.,
and Geiger, A. (2020). Convolutional occupancy net-
works. In European Conference on Computer Vision,
pages 523–540. Springer.
Qi, C. R., Su, H., Mo, K., and Guibas, L. J. (2017a). Point-
net: Deep learning on point sets for 3d classification
and segmentation. In Proceedings of the IEEE con-
ference on computer vision and pattern recognition,
pages 652–660.
Qi, C. R., Yi, L., Su, H., and Guibas, L. J. (2017b). Point-
net++: Deep hierarchical feature learning on point sets
in a metric space. Advances in neural information pro-
cessing systems, 30.
Schroff, F., Kalenichenko, D., and Philbin, J. (2015).
Facenet: A unified embedding for face recognition
and clustering. In Proceedings of the IEEE conference
on computer vision and pattern recognition, pages
815–823.
Szegedy, C., Liu, W., Jia, Y., Sermanet, P., Reed, S.,
Anguelov, D., Erhan, D., Vanhoucke, V., and Rabi-
novich, A. (2015). Going deeper with convolutions.
In Proceedings of the IEEE conference on computer
vision and pattern recognition, pages 1–9.
Uy, M. A. and Lee, G. H. (2018). Pointnetvlad: Deep point
cloud based retrieval for large-scale place recognition.
In Proceedings of the IEEE conference on computer
vision and pattern recognition, pages 4470–4479.
Wang, J., Song, Y., Leung, T., Rosenberg, C., Wang, J.,
Philbin, J., Chen, B., and Wu, Y. (2014). Learning
fine-grained image similarity with deep ranking. In
Proceedings of the IEEE conference on computer vi-
sion and pattern recognition, pages 1386–1393.
Wang, J., Zhou, F., Wen, S., Liu, X., and Lin, Y. (2017).
Deep metric learning with angular loss. In Proceed-
ings of the IEEE international conference on com-
puter vision, pages 2593–2601.
Wang, Y., Sun, Y., Liu, Z., Sarma, S. E., Bronstein, M. M.,
and Solomon, J. M. (2019). Dynamic graph cnn
for learning on point clouds. Acm Transactions On
Graphics (tog), 38(5):1–12.
Wen, Y., Zhang, K., Li, Z., and Qiao, Y. (2016). A discrim-
inative feature learning approach for deep face recog-
nition. In European conference on computer vision,
pages 499–515. Springer.
Wu, Z., Song, S., Khosla, A., Yu, F., Zhang, L., Tang, X.,
and Xiao, J. (2015). 3d shapenets: A deep representa-
tion for volumetric shapes. In Proceedings of the IEEE
conference on computer vision and pattern recogni-
tion, pages 1912–1920.
Xing, E., Jordan, M., Russell, S. J., and Ng, A. (2002).
Distance metric learning with application to cluster-
ing with side-information. Advances in neural infor-
mation processing systems, 15.
Xu, W. (2021). Deep large margin nearest neighbor
for gait recognition. Journal of Intelligent Systems,
30(1):604–619.
Deep Distance Metric Learning for Similarity Preserving Embedding of Point Clouds
581