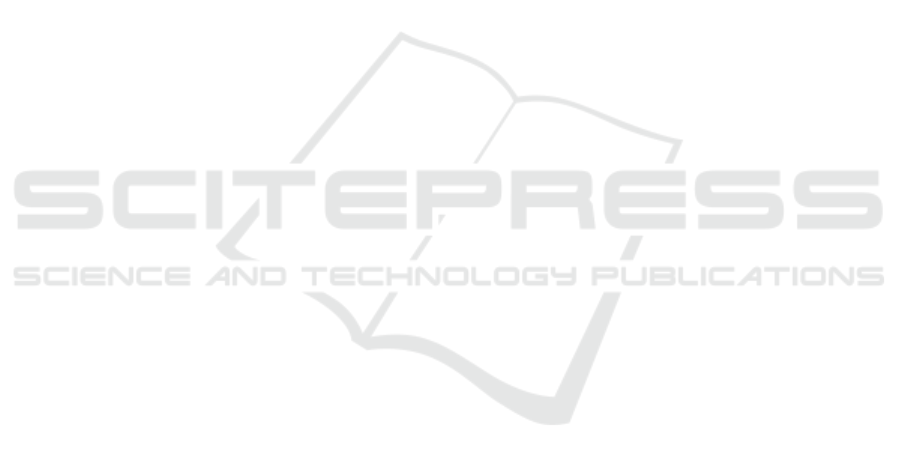
to further investigate the effects of other aggregation
algorithms that can overcome FedAvg limitations in
unbalanced data scenarios, as well as specific tech-
niques for dealing with unrepresented attacks classes
to improve the overall classification performance.
REFERENCES
Al-Garadi, M. A., Mohamed, A., Al-Ali, A. K., Du, X.,
Ali, I., and Guizani, M. (2020). A survey of machine
and deep learning methods for internet of things (iot)
security. IEEE Communications Surveys & Tutorials,
22(3):1646–1685.
Alsaedi, A., Moustafa, N., Tari, Z., Mahmood, A., and An-
war, A. (2020). Ton-iot telemetry dataset: A new gen-
eration dataset of iot and iiot for data-driven intrusion
detection systems. IEEE Access, 8:165130–165150.
Aouedi, O., Piamrat, K., Muller, G., and Singh, K. (2022).
Intrusion detection for softwarized networks with
semi-supervised federated learning. In ICC 2022 -
IEEE International Conference on Communications,
pages 5244–5249.
Campos, E. M., Saura, P. F., Gonz
´
alez-Vidal, A.,
Hern
´
andez-Ramos, J. L., and et al. (2021). Evaluating
federated learning for intrusion detection in internet of
things: Review and challenges. Computer Networks,
page 108661.
Chaabouni, N., Mosbah, M., Zemmari, A., Sauvignac, C.,
and Faruki, P. (2019). Network intrusion detection for
iot security based on learning techniques. IEEE Com-
munications Surveys & Tutorials, 21(3):2671–2701.
Ghosh, A., Chung, J., Yin, D., and Ramchandran, K.
(2020). An efficient framework for clustered federated
learning. Advances in Neural Information Processing
Systems, 33:19586–19597.
Hassija, V., Chamola, V., Saxena, V., Jain, D., and et al.
(2019). A survey on iot security: Application areas,
security threats, and solution architectures. IEEE Ac-
cess, 7:82721–82743.
Hellinger, E. (1909). Journal f
¨
ur die reine und angewandte
Mathematik, 210.
Hsu, T.-M. H., Qi, H., and Brown, M. (2019). Mea-
suring the effects of non-identical data distribution
for federated visual classification. arXiv preprint
arXiv:1909.06335.
Jiang, Y., Kone
ˇ
cn
`
y, J., Rush, K., and Kannan, S.
(2019). Improving federated learning personaliza-
tion via model agnostic meta learning. arXiv preprint
arXiv:1909.12488.
Kairouz, P., McMahan, H. B., Avent, B., Bellet, A.,
et al. (2021). Advances and open problems in feder-
ated learning. Foundations and Trends® in Machine
Learning, 14(1–2):1–210.
Khan, W. Z., Ahmed, E., Hakak, S., Yaqoob, I., and Ahmed,
A. (2019). Edge computing: A survey. Future Gener-
ation Computer Systems, 97:219–235.
Li, T., Sahu, A. K., Talwalkar, A., and Smith, V. (2020).
Federated learning: Challenges, methods, and fu-
ture directions. IEEE Signal Processing Magazine,
37(3):50–60.
Lin, J. (1991). Divergence measures based on the shannon
entropy. IEEE Transactions on Information Theory,
37(1):145–151.
Liu, H. and Lang, B. (2019). Machine learning and deep
learning methods for intrusion detection systems: A
survey. Applied Sciences, 9:4396.
MacKay, D. J. C. (2003). Information Theory, Inference,
and Learning Algorithms. Copyright Cambridge Uni-
versity Press.
McMahan, B., Moore, E., Ramage, D., Hampson, S., and
y Arcas, B. A. (2017). Communication-efficient learn-
ing of deep networks from decentralized data. In Ar-
tificial intelligence and statistics, pages 1273–1282.
PMLR.
Mothukuri, V., Parizi, R., Pouriyeh, S., Huang, Y., and et al.
(2020). A survey on security and privacy of federated
learning. Future Generation Computer Systems.
Moustafa, N., Hu, J., and Slay, J. (2018). A holistic re-
view of network anomaly detection systems: A com-
prehensive survey. Journal of Network and Computer
Applications, 128.
Rahman, S. A., Tout, H., Talhi, C., and Mourad, A. (2020).
Internet of things intrusion detection: Centralized,
on-device, or federated learning? IEEE Network,
34(6):310–317.
Rey, V., S
´
anchez, P. M. S., Celdr
´
an, A. H., and Bovet, G.
(2022). Federated learning for malware detection in
iot devices. Computer Networks, 204:108693.
Sarhan, M., Layeghy, S., Moustafa, N., Gallagher, M., and
Portmann, M. (2022). Feature extraction for ma-
chine learning-based intrusion detection in iot net-
works. Digital Communications and Networks.
Sarhan, M., Layeghy, S., and Portmann, M. (2021). Evalu-
ating standard feature sets towards increased general-
isability and explainability of ml-based network intru-
sion detection. arXiv preprint arXiv:2104.07183.
Sattler, F., M
¨
uller, K.-R., and Samek, W. (2020). Clustered
federated learning: Model-agnostic distributed mul-
titask optimization under privacy constraints. IEEE
transactions on neural networks and learning systems,
32(8):3710–3722.
Savi, M. and Olivadese, F. (2021). Short-term energy con-
sumption forecasting at the edge: A federated learning
approach. IEEE Access, 9:95949–95969.
Shone, N., Ngoc, T. N., Phai, V. D., and Shi, Q. (2018). A
deep learning approach to network intrusion detection.
IEEE Transactions on Emerging Topics in Computa-
tional Intelligence, 2(1):41–50.
Sinha, B. and Dhanalakshmi, R. (2021). Recent advance-
ments and challenges of internet of things in smart
agriculture: A survey. Future Generation Computer
Systems, 126.
Smith, V., Chiang, C.-K., Sanjabi, M., and Talwalkar, A.
(2017). Federated multi-task learning. In Proceed-
ings of the 31st International Conference on Neu-
ral Information Processing Systems, NIPS’17, page
4427–4437, Red Hook, NY, USA. Curran Associates
Inc.
A Clustering Strategy for Enhanced FL-Based Intrusion Detection in IoT Networks
159