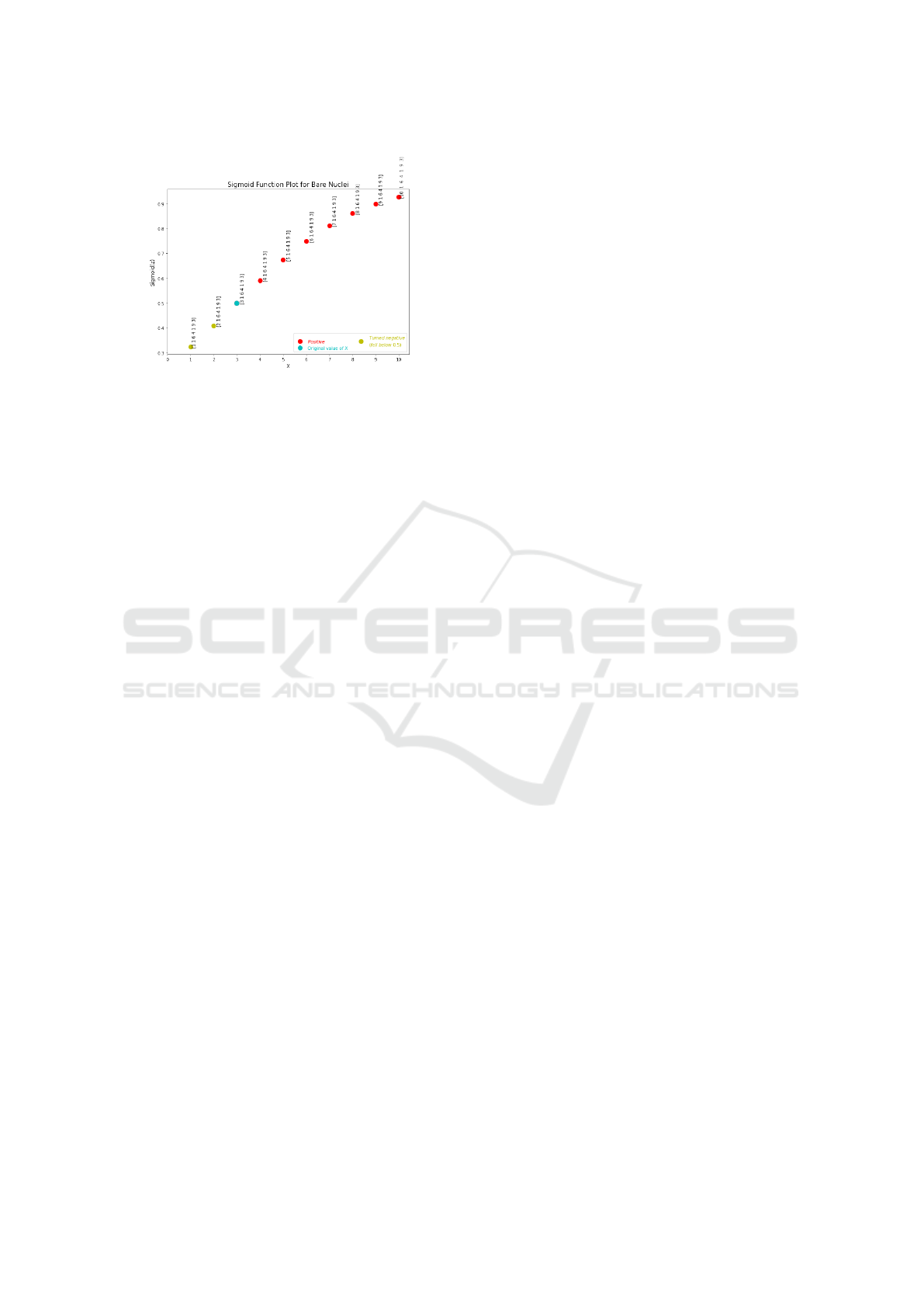
Figure 10: Classification shift at classification border for
bare nuclei.
as a powerful tool, it is important not to forget its lim-
itations, such as the assumption of the absence of high
intercorrelations among the predictors. Therefore, in
future work, we will examine the XAI setup for med-
ical applications with more powerful methods such as
Deep Learning.
Our contribution is a completely transparent, in-
terpretable, and explainable model, developed with
the purpose of aiding medical personnel in the
decision-making process. It contributes towards ex-
tending XAI to regression models, by adapting an
NLP method as a way to access desired explanations.
In future work, we plan to perform user experiments
in order to rate the helpfulness of our model.
REFERENCES
Anisha, P., Reddy C, K., Apoorva, K., and Mangipudi, C.
(2021). Early diagnosis of breast cancer prediction
using random forest classifier. IOP Conference Series:
Materials Science and Engineering, 1116:012187.
Aviv, R. I., d’Esterre, C. D., Murphy, B. D., Hopyan, J. J.,
Buck, B., Mallia, G., Li, V., Zhang, L., Symons, S. P.,
and Lee, T.-Y. (2009). Hemorrhagic transformation of
ischemic stroke: Prediction with ct perfusion. Radiol-
ogy, 250(3):867–877.
Ayer, T., Chhatwal, J., Alagoz, O., Kahn, C. E., Woods,
R. W., and Burnside, E. S. (2010). Comparison of
logistic regression and artificial neural network mod-
els in breast cancer risk estimation. RadioGraphics,
30(1):13–22.
Cashmore, M., Collins, A., Krarup, B., Krivic, S., Maga-
zzeni, D., and Smith, D. E. (2019). Towards explain-
able AI planning as a service. CoRR, abs/1908.05059.
Chakrobartty, S. and El-Gayar, O. F. (2021). Explainable
artificial intelligence in the medical domain: A sys-
tematic review. In Chan, Y. E., Boudreau, M., Aubert,
B., Par
´
e, G., and Chin, W., editors, 27th Americas
Conference on Information Systems. Association for
Information Systems.
Gunning, D. and Aha, D. W. (2019). Darpa’s explain-
able artificial intelligence (XAI) program. AI Mag.,
40(2):44–58.
Hastie, T., Tibshirani, R., and Friedman, J. H. (2009). The
Elements of Statistical Learning: Data Mining, Infer-
ence, and Prediction, 2nd Edition. Springer Series in
Statistics. Springer.
Krarup, B., Krivic, S., Magazzeni, D., Long, D., Cashmore,
M., and Smith, D. E. (2021). Contrastive explanations
of plans through model restrictions. J. Artif. Intell.
Res., 72:533–612.
Krieger, H. (2016). Capturing graded knowledge and uncer-
tainty in a modalized fragment of OWL. In van den
Herik, H. J. and Filipe, J., editors, Proceedings of the
8th International Conference on Agents and Artificial
Intelligence, Vol.2, pages 19–30. SciTePress.
Letzgus, S., Wagner, P., Lederer, J., Samek, W., M
¨
uller, K.,
and Montavon, G. (2021). Toward explainable AI for
regression models. CoRR, abs/2112.11407.
Lipton, Z. C. (2018). The mythos of model interpretability.
Commun. ACM, 61(10):36–43.
Lou, Y., Caruana, R., and Gehrke, J. (2012). Intelligible
models for classification and regression. In The 18th
ACM SIGKDD International Conference on Knowl-
edge Discovery and Data Mining, KDD ’12, 2012,
pages 150–158. ACM.
Mangasarian, O. L., Street, W. N., and Wolberg, W. H.
(1995). Breast cancer diagnosis and prognosis via lin-
ear programming. Oper. Res., 43(4):570–577.
Miller, T. (2019). Explanation in artificial intelligence: In-
sights from the social sciences. Artif. Intell., 267:1–
38.
Naji, M. A., Filali, S. E., Aarika, K., Benlahmar, E. H., Ab-
delouhahid, R. A., and Debauche, O. (2021). Machine
learning algorithms for breast cancer prediction and
diagnosis. Procedia Computer Science, 191:487–492.
Sultana, J. and Jilani, A. K. (2018). Predicting breast cancer
using logistic regression and multi-class classifiers.
International Journal of Engineering & Technology,
7(4.20).
Wolberg, W. H. and Mangasarian, O. L. (1990). Multisur-
face method of pattern separation for medical diag-
nosis applied to breast cytology. Proceedings of the
National Academy of Sciences, 87(23):9193–9196.
Yang, G., Ye, Q., and Xia, J. (2022). Unbox the black-box
for the medical explainable ai via multi-modal and
multi-centre data fusion: A mini-review, two show-
cases and beyond. Information Fusion, 77:29–52.
Zhao, Y., Yang, F., Fang, Y., Liu, H., Zhou, N., Zhang,
J., Sun, J., Yang, S., Menze, B. H., Fan, X., and
Yao, J. (2020). Predicting lymph node metastasis
using histopathological images based on multiple in-
stance learning with deep graph convolution. In 2020
IEEE/CVF Conference on Computer Vision and Pat-
tern Recognition, CVPR 2020, Seattle, WA, USA,
June 13-19, 2020, pages 4836–4845. Computer Vision
Foundation / IEEE.
ICAART 2023 - 15th International Conference on Agents and Artificial Intelligence
168