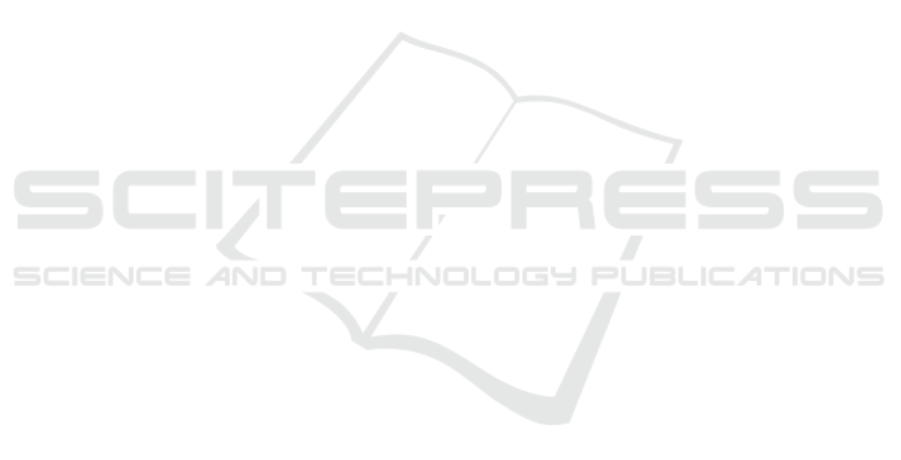
Sensors, 19(9):1991.
Anders, C. and Arnrich, B. (2022). Wearable electroen-
cephalography and multi-modal mental state classifi-
cation: A systematic literature review. Computers in
Biology and Medicine, page 106088.
Antonenko, P., Paas, F., Grabner, R., and Van Gog,
T. (2010). Using electroencephalography to mea-
sure cognitive load. Educational psychology review,
22(4):425–438.
Arpaia, P., Moccaldi, N., Prevete, R., Sannino, I., and
Tedesco, A. (2020). A wearable eeg instrument
for real-time frontal asymmetry monitoring in worker
stress analysis. IEEE Transactions on Instrumentation
and Measurement, 69(10):8335–8343.
Arsalan, A., Majid, M., Butt, A. R., and Anwar, S. M.
(2019). Classification of perceived mental stress using
a commercially available eeg headband. IEEE jour-
nal of biomedical and health informatics, 23(6):2257–
2264.
Asif, A., Majid, M., and Anwar, S. M. (2019). Human stress
classification using eeg signals in response to music
tracks. Computers in biology and medicine, 107:182–
196.
Ayres, P., Lee, J. Y., Paas, F., and van Merri
¨
enboer, J. J.
(2021). The validity of physiological measures to
identify differences in intrinsic cognitive load. Fron-
tiers in Psychology, 12.
Barros, C., Pereira, A. R., Sampaio, A., Buj
´
an, A., and
Pinal, D. (2022). Frontal alpha asymmetry and neg-
ative mood: A cross-sectional study in older and
younger adults. Symmetry, 14(8):1579.
Barua, S., Ahmed, M. U., and Begum, S. (2020). Towards
intelligent data analytics: A case study in driver cog-
nitive load classification. Brain sciences, 10(8):526.
Bashivan, P., Rish, I., and Heisig, S. (2016). Mental
state recognition via wearable eeg. arXiv preprint
arXiv:1602.00985.
Borghini, G., Astolfi, L., Vecchiato, G., Mattia, D., and Ba-
biloni, F. (2014). Measuring neurophysiological sig-
nals in aircraft pilots and car drivers for the assessment
of mental workload, fatigue and drowsiness. Neuro-
science & Biobehavioral Reviews, 44:58–75.
Chen, F., Zhou, J., Wang, Y., Yu, K., Arshad, S. Z., Khawaji,
A., and Conway, D. (2016). Robust multimodal cog-
nitive load measurement. Springer.
Fangmeng, Z., Siriaraya, P., Choi, D., and Kuwahara, N.
(2020). Textile eeg cap using dry-comb electrodes
for emotion detection of elderly people. International
Journal of Advanced Computer Science and Applica-
tions, 11(4).
Gevins, A., Smith, M. E., Leong, H., McEvoy, L., Whit-
field, S., Du, R., and Rush, G. (1998). Monitoring
working memory load during computer-based tasks
with eeg pattern recognition methods. Human factors,
40(1):79–91.
Grimes, D., Tan, D. S., Hudson, S. E., Shenoy, P., and Rao,
R. P. (2008). Feasibility and pragmatics of classifying
working memory load with an electroencephalograph.
In Proceedings of the SIGCHI Conference on Human
Factors in Computing Systems, pages 835–844.
Hart, S. G. and Staveland, L. E. (1988). Development of
nasa-tlx (task load index): Results of empirical and
theoretical research. In Advances in psychology, vol-
ume 52, pages 139–183. Elsevier.
Ismail, L. E. and Karwowski, W. (2020). Applications of
eeg indices for the quantification of human cognitive
performance: A systematic review and bibliometric
analysis. PLoS One, 15(12):e0242857.
Jap, B. T., Lal, S., Fischer, P., and Bekiaris, E. (2009). Us-
ing eeg spectral components to assess algorithms for
detecting fatigue. Expert Systems with Applications,
36(2):2352–2359.
Jim
´
enez-Guarneros, M. and G
´
omez-Gil, P. (2017). Cross-
subject classification of cognitive loads using a
recurrent-residual deep network. In 2017 IEEE Sym-
posium Series on Computational Intelligence (SSCI),
pages 1–7. IEEE.
Kane, M. J. and Engle, R. W. (2002). The role of prefrontal
cortex in working-memory capacity, executive atten-
tion, and general fluid intelligence: An individual-
differences perspective. Psychonomic bulletin & re-
view, 9(4):637–671.
Katmah, R., Al-Shargie, F., Tariq, U., Babiloni, F., Al-
Mughairbi, F., and Al-Nashash, H. (2021). A review
on mental stress assessment methods using eeg sig-
nals. Sensors, 21(15):5043.
Kutafina, E., Heiligers, A., Popovic, R., Brenner, A., Han-
kammer, B., Jonas, S. M., Mathiak, K., and Zweer-
ings, J. (2021). Tracking of mental workload with a
mobile eeg sensor. Sensors, 21(15):5205.
Longo, L., Wickens, C. D., Hancock, P. A., and Hancock,
G. M. (2022). Human mental workload: A survey and
a novel inclusive definition. Frontiers in psychology,
13.
Marschall, J
¨
org, H. S. K. K.-M. N. H.-D. (2020). Gesund-
heitsreport 2020 stress in der modernen arbeitswelt
sonderanalyse: Digitalisierung und homeoffice in der
corona-krise.
Moontaha, S., Steckhan, N., Kappattanavar, A., Surges, R.,
and Arnrich, B. (2020). Self-prediction of seizures in
drug resistance epilepsy using digital phenotyping: a
concept study. In Proceedings of the 14th EAI Interna-
tional Conference on Pervasive Computing Technolo-
gies for Healthcare, pages 384–387.
M
¨
uhl, C., Jeunet, C., and Lotte, F. (2014). Eeg-based work-
load estimation across affective contexts. Frontiers in
neuroscience, 8:114.
Negi, S. and Mitra, R. (2018). Eeg metrics to determine
cognitive load and affective states: a pilot study. In
Proceedings of the 2018 ACM international joint con-
ference and 2018 international symposium on perva-
sive and ubiquitous computing and wearable comput-
ers, pages 182–185.
Pang, L., Guo, L., Zhang, J., Wanyan, X., Qu, H., and
Wang, X. (2021). Subject-specific mental workload
classification using eeg and stochastic configuration
network (scn). Biomedical Signal Processing and
Control, 68:102711.
Peirce, J., Hirst, R., and MacAskill, M. (2022). Building
experiments in PsychoPy. Sage.
HEALTHINF 2023 - 16th International Conference on Health Informatics
50