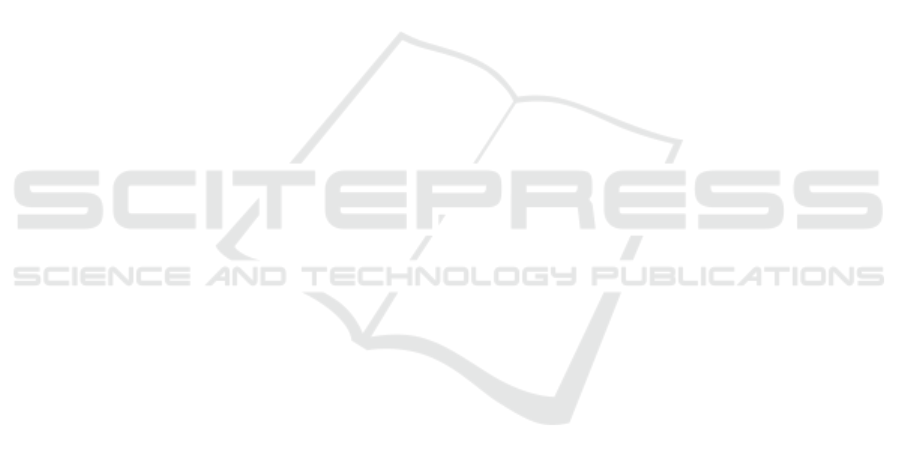
Hasan DIKYUVA, Bahtiyar MAKAROGLU, E. A. (1995).
Turkish Sign Language Manual for Adults. Turkish
Ministry of Education, Ankara (1995). Ankara.
Ioffe, S. and Szegedy, C. (2015a). Batch normalization: Ac-
celerating deep network training by reducing internal
covariate shift. In International conference on machine
learning, pages 448–456. PMLR.
Ioffe, S. and Szegedy, C. (2015b). Batch normalization: Ac-
celerating deep network training by reducing internal
covariate shift. In International conference on machine
learning, pages 448–456. PMLR.
Keskin, C., Kiraç, F., Kara, Y. E., and Akarun, L. (2012).
Randomized decision forests for static and dynamic
hand shape classification. In 2012 IEEE Computer
Society Conference on Computer Vision and Pattern
Recognition Workshops, pages 31–36.
Krogh, A. and Hertz, J. A. (1992). A simple weight decay
can improve generalization. In Advances in neural
information processing systems, pages 950–957.
Kuznetsova, A., Leal-Taixé, L., and Rosenhahn, B. (2013).
Real-time sign language recognition using a consumer
depth camera. In Proceedings of the IEEE interna-
tional conference on computer vision workshops, pages
83–90.
LeCun, Y., Bengio, Y., et al. (1995). Convolutional networks
for images, speech, and time series. The handbook of
brain theory and neural networks, 3361(10):1995.
LeCun, Y., Bottou, L., Bengio, Y., and Haffner, P. (1998).
Gradient-based learning applied to document recogni-
tion. Proceedings of the IEEE, 86(11):2278–2324.
LeCun, Y., Denker, J. S., and Solla, S. A. (1990). Opti-
mal brain damage. In Advances in neural information
processing systems, pages 598–605.
Li, S.-Z., Yu, B., Wu, W., Su, S.-Z., and Ji, R.-R. (2015).
Feature learning based on sae–pca network for human
gesture recognition in rgbd images. Neurocomputing,
151:565–573.
Mercanoglu, O. and Keles, H. (2020). Autsl: A large scale
multi-modal turkish sign language dataset and baseline
methods. IEEE Access, 8:181340–181355.
Nagi, J., Ducatelle, F., Di Caro, G. A., Cire¸san, D., Meier,
U., Giusti, A., Nagi, F., Schmidhuber, J., and Gam-
bardella, L. M. (2011). Max-pooling convolutional
neural networks for vision-based hand gesture recogni-
tion. In 2011 IEEE International Conference on Signal
and Image Processing Applications (ICSIPA), pages
342–347.
Oz, C. and Leu, M. C. (2011). American sign language
word recognition with a sensory glove using artificial
neural networks. Engineering Applications of Artificial
Intelligence, 24(7):1204–1213.
Park, J.-J. and Kwon, C.-K. (2021). Korean finger num-
ber gesture recognition based on cnn using surface
electromyography signals. Journal of Electrical Engi-
neering & Technology, 16(1):591–598.
Pigou, L., Dieleman, S., Kindermans, P.-J., and Schrauwen,
B. (2014). Sign language recognition using convolu-
tional neural networks. In European Conference on
Computer Vision, pages 572–578. Springer.
Prechelt, L. (1998). Early stopping-but when? In Neural
Networks: Tricks of the trade, pages 55–69. Springer.
Pugeault, N. and Bowden, R. (2011). Spelling it out: Real-
time asl fingerspelling recognition. In 2011 IEEE In-
ternational conference on computer vision workshops
(ICCV workshops), pages 1114–1119. IEEE.
Rao, G. A., Syamala, K., Kishore, P., and Sastry, A. (2018a).
Deep convolutional neural networks for sign language
recognition. In 2018 Conference on Signal Processing
And Communication Engineering Systems (SPACES),
pages 194–197. IEEE.
Rao, G. A., Syamala, K., Kishore, P., and Sastry, A. (2018b).
Deep convolutional neural networks for sign language
recognition. In 2018 Conference on Signal Processing
And Communication Engineering Systems (SPACES),
pages 194–197. IEEE.
Shin, H., Kim, W. J., and Jang, K.-a. (2019). Korean sign
language recognition based on image and convolution
neural network. In Proceedings of the 2nd Interna-
tional Conference on Image and Graphics Processing,
pages 52–55.
Van den Bergh, M. and Van Gool, L. (2011). Combining
rgb and tof cameras for real-time 3d hand gesture in-
teraction. In 2011 IEEE workshop on applications of
computer vision (WACV), pages 66–72. IEEE.
Wadhawan, A. and Kumar, P. (2020). Deep learning-based
sign language recognition system for static signs. Neu-
ral computing and applications, 32(12):7957–7968.
Weigend, A. S., Rumelhart, D. E., and Huberman, B. A.
(1991). Generalization by weight-elimination with
application to forecasting. In Advances in neural infor-
mation processing systems, pages 875–882.
Wu, J., Tian, Z., Sun, L., Estevez, L., and Jafari, R. (2015).
Real-time american sign language recognition using
wrist-worn motion and surface emg sensors. In 2015
IEEE 12th International Conference on Wearable and
Implantable Body Sensor Networks (BSN), pages 1–6.
IEEE.
Yamashita, R., Nishio, M., Do, R. K. G., and Togashi, K.
(2018). Convolutional neural networks: an overview
and application in radiology. Insights into imaging,
9(4):611–629.
Zhang, C., Yang, X., and Tian, Y. (2013). Histogram of
3d facets: A characteristic descriptor for hand gesture
recognition. In 2013 10th IEEE International Confer-
ence and Workshops on Automatic Face and Gesture
Recognition (FG), pages 1–8.
VISAPP 2023 - 18th International Conference on Computer Vision Theory and Applications
186